FRTB: Improving the Modellability of Risk Factors
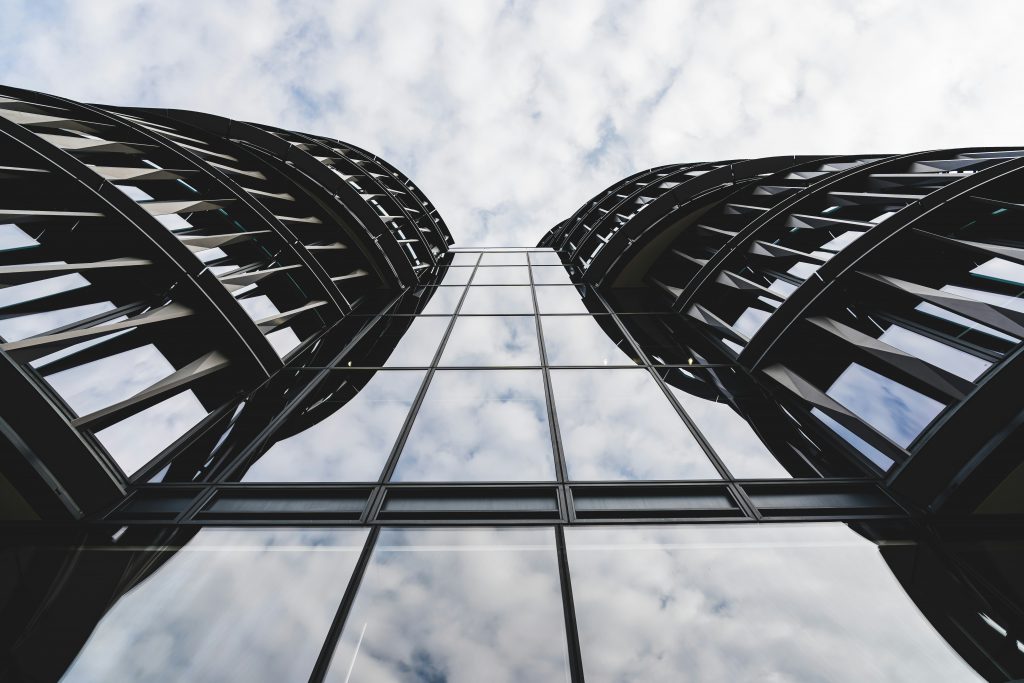
Explore key strategies to minimize non-modellable risk factors (NMRFs) under FRTB’s internal models approach, including enhancing data, creating proxies, and customizing bucketing, to manage your bank’s capital requirements more effectively.
Under the FRTB internal models approach (IMA), the capital calculation of risk factors is dependent on whether the risk factor is modellable. Insufficient data will result in more non-modellable risk factors (NMRFs), significantly increasing associated capital charges.
NMRFs
Risk factor modellability and NMRFs
The modellability of risk factors is a new concept which was introduced under FRTB and is based on the liquidity of each risk factor. Modellability is measured using the number of ‘real prices’ which are available for each risk factor. Real prices are transaction prices from the institution itself, verifiable prices for transactions between arms-length parties, prices from committed quotes, and prices from third party vendors.
For a risk factor to be classed as modellable, it must have a minimum of 24 real prices per year, no 90-day period with less than four prices, and a minimum of 100 real prices in the last 12 months (with a maximum of one real price per day). The Risk Factor Eligibility Test (RFET), outlined in FRTB, is the process which determines modellability and is performed quarterly. The results of the RFET determine, for each risk factor, whether the capital requirements are calculated by expected shortfall or stressed scenarios.
Consequences of NMRFs for banks
Modellable risk factors are capitalised via expected shortfall calculations which allow for diversification benefits. Conversely, capital for NMRFs is calculated via stressed scenarios which result in larger capital charges. This is due to longer liquidity horizons and more prudent assumptions used for aggregation. Although it is expected that a low proportion of risk factors will be classified as non-modellable, research shows that they can account for over 30% of total capital requirements.
There are multiple techniques that banks can use to reduce the number and impact of NMRFs, including the use of external data, developing proxies, and modifying the parameterisation of risk factor curves and surfaces. As well as focusing on reducing the number of NMRFs, banks will also need to develop early warning systems and automated reporting infrastructures to monitor the modellability of risk factors. These tools help to track and predict modellability issues, reducing the likelihood that risk factors will fail the RFET and increase capital requirements.

Methods for reducing the number of NMRFs
Banks should focus on reducing their NMRFs as they are associated with significantly higher capital charges. There are multiple approaches which can be taken to increase the likelihood that a risk factor passes the RFET and is classed as modellable.
Enhancing internal data
The simplest way for banks to reduce NMRFs is by increasing the amount of data available to them. Augmenting internal data with external data increases the number of real prices available for the RFET and reduces the likelihood of NMRFs. Banks can purchase additional data from external data vendors and data pooling services to increase the size and quality of datasets.
It is important for banks to initially investigate their internal data and understand where the gaps are. As data providers vary in which services and information they provide, banks should not only focus on the types and quantity of data available. For example, they should also consider data integrity, user interfaces, governance, and security. Many data providers also offer FRTB-specific metadata, such as flags for RFET liquidity passes or fails.
Finally, once a data provider has been chosen, additional effort will be required to resolve discrepancies between internal and external data and ensure that the external data follows the same internal standards.
Creating risk factor proxies
Proxies can be developed to reduce the number or magnitude of NMRFs, however, regulation states that their use must be limited. Proxies are developed using either statistical or rules-based approaches.
Rules-based approaches are simplistic, yet generally less accurate. They find the “closest fit” modellable risk factor using more qualitative methods, e.g. using the closest tenor on the interest rate curve. Alternatively, more accurate approaches model the relationship between the NMRF and modellable risk factors using statistical methods. Once a proxy is determined, it is classified as modellable and only the basis between it and the NMRF is required to be capitalised using stressed scenarios.
Determining proxies can be time-consuming as it requires exploratory work with uncertain outcomes. Additional ongoing effort will also be required by validation and monitoring units to ensure the relationship holds and the regulator is satisfied.
Developing own bucketing approach
Instead of using the prescribed bucketing approach, banks can use their own approach to maximise the number of real price observations for each risk factor.
For example, if a risk model requires a volatility surface to price, there are multiple ways this can be parametrised. One method could be to split the surface into a 5x5 grid, creating 25 buckets that would each require sufficient real price observations to be classified as modellable. Conversely, the bank could instead split the surface into a 2x2 grid, resulting in only four buckets. The same number of real price observations would then need to be allocated between significantly less buckets, decreasing the chances of a risk factor being a NMRF.
It should be noted that the choice of bucketing approach affects other aspects of FRTB. Profit and Loss Attribution (PLA) uses the same buckets of risk factors as chosen for the RFET. Increasing the number of buckets may increase the chances of passing PLA, however, also increases the likelihood of risk factors failing the RFET and being classed as NMRFs.
Conclusion
In this article, we have described several potential methods for reducing the number of NMRFs. Although some of the suggested methods may be more cost effective or easier to implement than others, banks will most likely, in practice, need to implement a combination of these strategies in parallel. The modellability of risk factors is clearly an important part of the FRTB regulation for banks as it has a direct impact on required capital. Banks should begin to develop strategies for reducing the number of NMRFs as early as possible if they are to minimise the required capital when FRTB goes live.
Targeted Review of Internal Models (TRIM): Review of observations and findings for Traded Risk
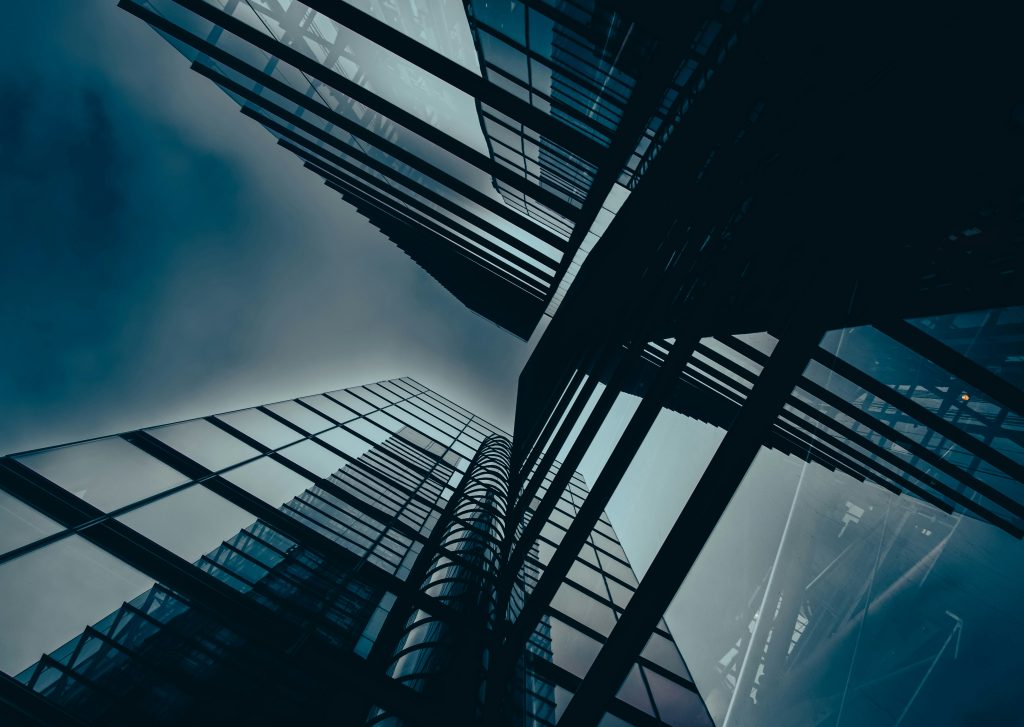
Explore key strategies to minimize non-modellable risk factors (NMRFs) under FRTB’s internal models approach, including enhancing data, creating proxies, and customizing bucketing, to manage your bank’s capital requirements more effectively.
The EBA has recently published the findings and observations from their TRIM on-site inspections. A significant number of deficiencies were identified and are required to be remediated by institutions in a timely fashion.
Since the Global Financial Crisis 2007-09, concerns have been raised regarding the complexity and variability of the models used by institutions to calculate their regulatory capital requirements. The lack of transparency behind the modelling approaches made it increasingly difficult for regulators to assess whether all risks had been appropriately and consistently captured.
The TRIM project was a large-scale multi-year supervisory initiative launched by the ECB at the beginning of 2016. The project aimed to confirm the adequacy and appropriateness of approved Pillar I internal models used by Significant Institutions (SIs) in euro area countries. This ensured their compliance with regulatory requirements and aimed to harmonise supervisory practices relating to internal models.
TRIM executed 200 on-site internal model investigations across 65 SIs from over 10 different countries. Over 5,800 deficiencies were identified. Findings were defined as deficiencies which required immediate supervisory attention. They were categorised depending on the actual or potential impact on the institution’s financial situation, the levels of own funds and own funds requirements, internal governance, risk control, and management.
The findings have been followed up with 253 binding supervisory decisions which request that the SIs mitigate these shortcomings within a timely fashion. Immediate action was required for findings that were deemed to take a significant time to address.
Assessment of Market Risk
TRIM assessed the VaR/sVaR models of 31 institutions. The majority of severe findings concerned the general features of the VaR and sVaR modelling methodology, such as data quality and risk factor modelling.
19 out of 31 institutions used historical simulation, seven used Monte Carlo, and the remainder used either a parametric or mixed approach. 17 of the historical simulation institutions, and five using Monte Carlo, used full revaluation for most instruments. Most other institutions used a sensitivities-based pricing approach.
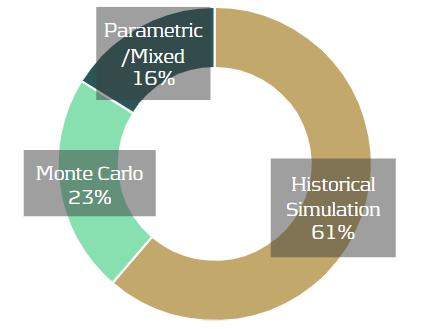
VaR/sVaR Methodology
Data: Issues with data cleansing, processing and validation were seen in many institutions and, on many occasions, data processes were poorly documented.
Risk Factors: In many cases, risk factors were missing or inadequately modelled. There was also insufficient justification or assessment of assumptions related to risk factor modelling.
Pricing: Institutions frequently had inadequate pricing methods for particular products, leading to a failure for the internal model to adequately capture all material price risks. In several cases, validation activities regarding the adequacy of pricing methods in the VaR model were insufficient or missing.
RNIME: Approximately two-thirds of the institutions had an identification process for risks not in model engines (RNIMEs). For ten of these institutions, this directly led to an RNIME add-on to the VaR or to the capital requirements.
Regulatory Backtesting
Period and Business Days: There was a lack of clear definitions of business and non-business days at most institutions. In many cases, this meant that institutions were trading on local holidays without adequate risk monitoring and without considering those days in the P&L and/or the VaR.
APL: Many institutions had no clear definition of fees, commissions or net interest income (NII), which must be excluded from the actual P&L (APL). Several institutions had issues with the treatment of fair value or other adjustments, which were either not documented, not determined correctly, or were not properly considered in the APL. Incorrect treatment of CVAs and DVAs and inconsistent treatment of the passage of time (theta) effect were also seen.
HPL: An insufficient alignment of pricing functions, market data, and parametrisation between the economic P&L (EPL) and the hypothetical P&L (HPL), as well as the inconsistent treatment of the theta effect in the HPL and the VaR, was seen in many institutions.
Internal Validation and Internal Backtesting
Methodology: In several cases, the internal backtesting methodology was considered inadequate or the levels of backtesting were not sufficient.
Hypothetical Backtesting: The required backtesting on hypothetical portfolios was either not carried or was only carried out to a very limited extent
IRC Methodology
TRIM assessed the IRC models of 17 institutions, reviewing a total of 19 IRC models. A total of 120 findings were identified and over 80% of institutions that used IRC models received at least one high-severity finding in relation to their IRC model. All institutions used a Monte Carlo simulation method, with 82% applying a weekly calculation. Most institutions obtained rates from external rating agency data. Others estimated rates from IRB models or directly from their front office function. As IRC lacks a prescriptive approach, the choice of modelling approaches between institutes exhibited a variety of modelling assumptions, as illustrated below.
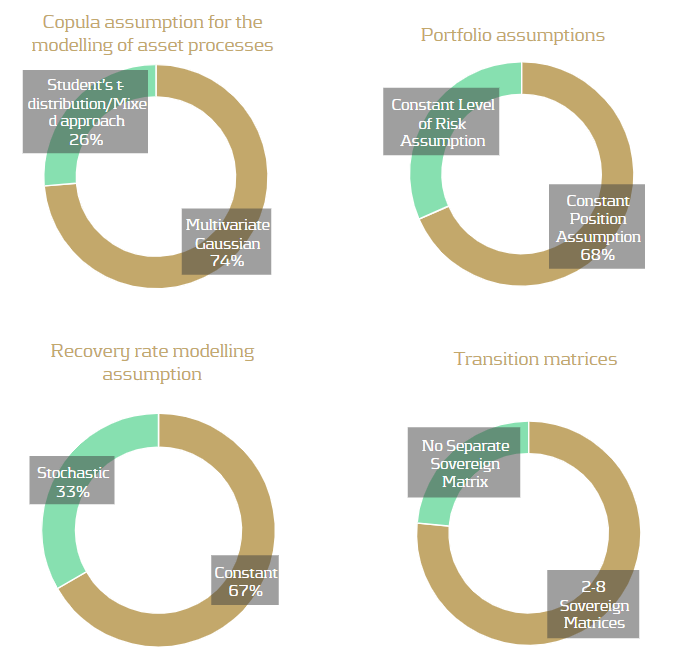
Recovery rates: The use of unjustified or inaccurate Recovery Rates (RR) and Probability of Defaults (PD) values were the cause of most findings. PDs close to or equal to zero without justification was a common issue, which typically arose for the modelling of sovereign obligors with high credit quality. 58% of models assumed PDs lower than one basis point, typically for sovereigns with very good ratings but sometimes also for corporates. The inconsistent assignment of PDs and RRs, or cases of manual assignment without a fully documented process, also contributed to common findings.
Modellingapproach: The lack of adequate modelling justifications presented many findings, including copula assumptions, risk factor choice, and correlation assumptions. Poor quality data and the lack of sufficient validation raised many findings for the correlation calibration.
Assessment of Counterparty Credit Risk
Eight banks faced on-site inspections under TRIM for counterparty credit risk. Whilst the majority of investigations resulted in findings of low materiality, there were severe weaknesses identified within validation units and overall governance frameworks.
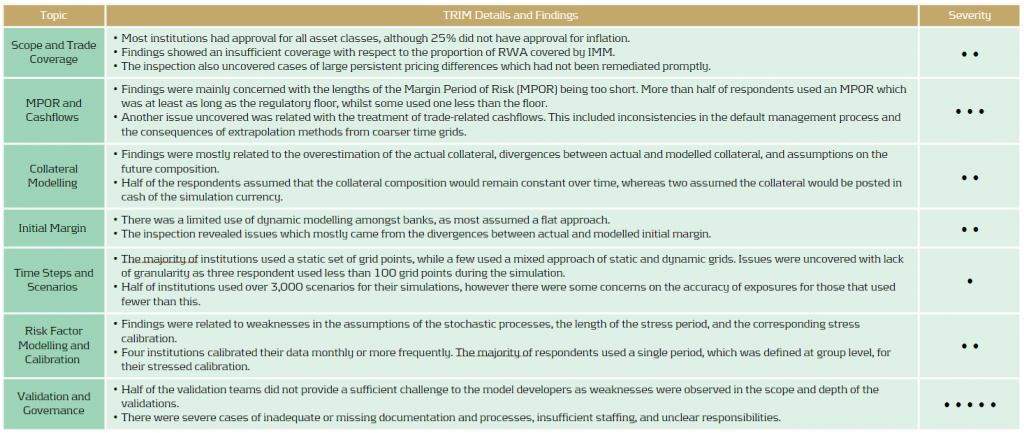
Conclusion
Based on the findings and responses, it is clear that TRIM has successfully highlighted several shortcomings across the banks. As is often the case, many issues seem to be somewhat systemic problems which are seen in a large number of the institutions. The issues and findings have ranged from fundamental problems, such as missing risk factors, to more complicated problems related to inadequate modelling methodologies. As such, the remediation of these findings will also range from low to high effort. The SIs will need to mitigate the shortcomings in a timely fashion, with some more complicated or impactful findings potentially taking a considerable time to remediate.
FRTB: Harnessing Synergies Between Regulations
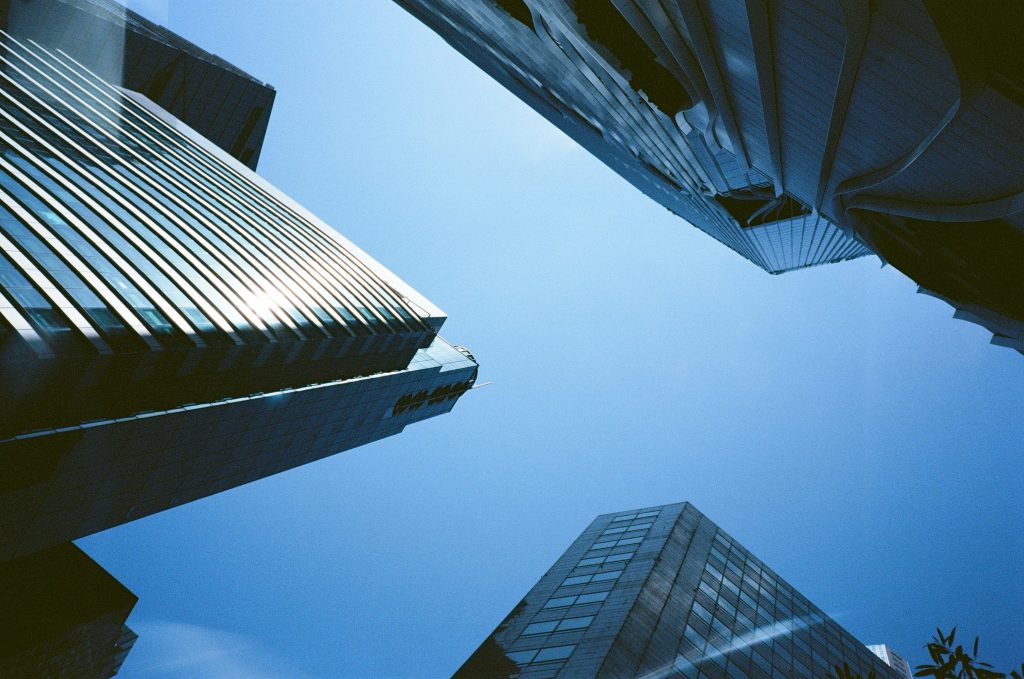
Explore key strategies to minimize non-modellable risk factors (NMRFs) under FRTB’s internal models approach, including enhancing data, creating proxies, and customizing bucketing, to manage your bank’s capital requirements more effectively.
Regulatory Landscape
Despite a delay of one year, many banks are struggling to be ready for FRTB in January 2023. Alongside the FRTB timeline, banks are also preparing for other important regulatory requirements and deadlines which share commonalities in implementation. We introduce several of these below.
SIMM
Initial Margin (IM) is the value of collateral required to open a position with a bank, exchange or broker. The Standard Initial Margin Model (SIMM), published by ISDA, sets a market standard for calculating IMs. SIMM provides margin requirements for financial firms when trading non-centrally cleared derivatives.
BCBS 239
BCBS 239, published by the Basel Committee on Banking Supervision, aims to enhance banks’ risk data aggregation capabilities and internal risk reporting practices. It focuses on areas such as data governance, accuracy, completeness and timeliness. The standard outlines 14 principles, although their high-level nature means that they are open to interpretation.
SA-CVA
Credit Valuation Adjustment (CVA) is a type of value adjustment and represents the market value of the counterparty credit risk for a transaction. FRTB splits CVA into two main approaches: BA-CVA, for smaller banks with less sophisticated trading activities, and SA-CVA, for larger banks with designated CVA risk management desks.
IBOR
Interbank Offered Rates (IBORs) are benchmark reference interest rates. As they have been subject to manipulation and due to a lack of liquidity, IBORs are being replaced by Alternative Reference Rates (ARRs). Unlike IBORs, ARRs are based on real transactions on liquid markets rather than subjective estimates.
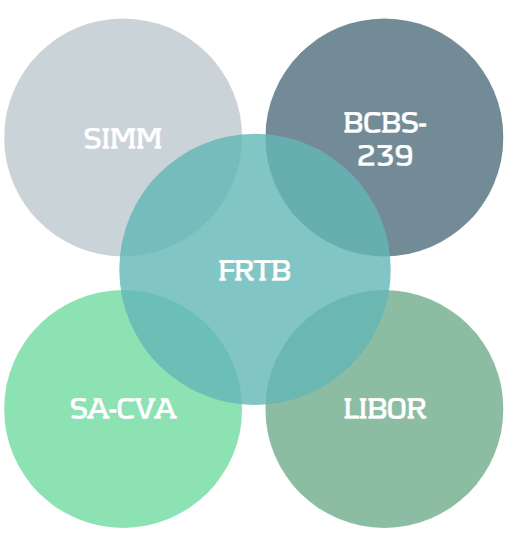
Synergies With Current Regulation
Existing SIMM and BCBS 239 frameworks and processes can be readily leveraged to reduce efforts in implementing FRTB frameworks.
SIMM
The overarching process of SIMM is very similar to the FRTB Sensitivities-based Method (SbM), including the identification of risk factors, calculation of sensitivities and aggregation of results. The outputs of SbM and SIMM are both based on delta, vega and curvature sensitivities. SIMM and FRTB both share four risk classes (IR, FX, EQ, and CM). However, in SIMM, credit is split across two risk classes (qualifying and non-qualifying), whereas it is split across three in FRTB (non-securitisation, securitisation and correlation trading). For both SbM and SIMM, banks should be able to decompose indices into their individual constituents.
We recommend that banks leverage the existing sensitivities infrastructure from SIMM for SbM calculations, use a shared risk factor mapping methodology between SIMM and FRTB when there is considerable alignment in risk classes, and utilise a common index look-through procedure for both SIMM and SbM index decompositions.
BCBS 239
BCBS 239 requires banks to review IT infrastructure, governance, data quality, aggregation policies and procedures. A similar review will be required in order to comply with the data standards of FRTB. The BCBS 239 principles are now in “Annex D” of the FRTB document, clearly showing the synergy between the two regulations. The quality, transparency, volume and consistency of data are important for both BCBS 239 and FRTB. Improving these factors allow banks to easily follow the BCBS 239 principles and decrease the capital charges of non-modellable risk factors. BCBS 239 principles, such as data completeness and timeliness, are also necessary for passing P&L attribution (PLA) under FRTB.
We recommend that banks use BCBS 239 principles when designing the necessary data frameworks for the FRTB Risk Factor Eligibility Test (RFET), support FRTB traceability requirements and supervisory approvals with existing BCBS 239 data lineage documentation, and produce market risk reporting for FRTB using the risk reporting infrastructure detailed in BCBS 239.
Synergies With Future Regulation
The IBOR transition and SA-CVA will become effective from 2023. Aligning the timelines and exploiting the similarities between FRTB, SA-CVA and the IBOR transition will support banks to be ready for all three regulatory deadlines.
SA-CVA
Four of the six risk classes in SA-CVA (IR, FX, EQ, and CM) are identical to those in SbM. SA-CVA, however, uses a reduced granularity for risk factors compared to SbM. The SA-CVA capital calculation uses a similar methodology to SbM by combining sensitivities with risk weights. SA-CVA also incorporates the same trade population and metadata as SbM. SA-CVA capital requirements must be calculated and reported to the supervisor at the same monthly frequency as for the market risk standardised approach.
We recommend that banks combine SA-CVA and SbM risk factor bucketing tasks in a common methodology to reduce overall effort, isolate common components of both models as a feeder model, allowing a single stream for model development and validation, and develop a single system architecture which can be configured for either SbM or SA-CVA.
IBOR Transition
Although not a direct synergy, the transition from IBORs will have a direct impact to the Internal Models Approach (IMA) for FRTB and eligibility of risk factors. As the use of IBORs are discontinued, banks may observe a reduction in the number of real-price observations for associated risk factors due to a reduction in market liquidity. It is not certain if these liquidity issues fall under the RFET exemptions for systemic circumstances, which apply to modellable risk factors which can no longer pass the test. It may be difficult for banks to obtain stress-period data for ARRs, which could lead to substantial efforts to produce and justify proxies. The transition may cause modifications to trading desk structure, the integration of external data providers, and enhanced operational requirements, which can all affect FRTB.
We recommend that banks investigate how much data is available for ARRs, for both stress-period calculations and real-price observations, develop any necessary proxies which will be needed to overcome data availability issues, as soon as possible, and Calculate IBOR capital consequences through the existing FRTB engine.
Conclusion
FRTB implementation is proving to be a considerable workload for banks, especially those considering opting for the IMA. Several FRTB requirements, such as PLA and RFET, are completely new requirements for banks. As we have shown in this article, there are several other important regulatory requirements which banks are currently working towards. As such, we recommend that banks should leverage the synergies which are seen across this regulatory landscape to reduce the complexity and workload of FRTB.