Zanders listed on Swift Customer Security Programme (CSP) Assessment Providers directory
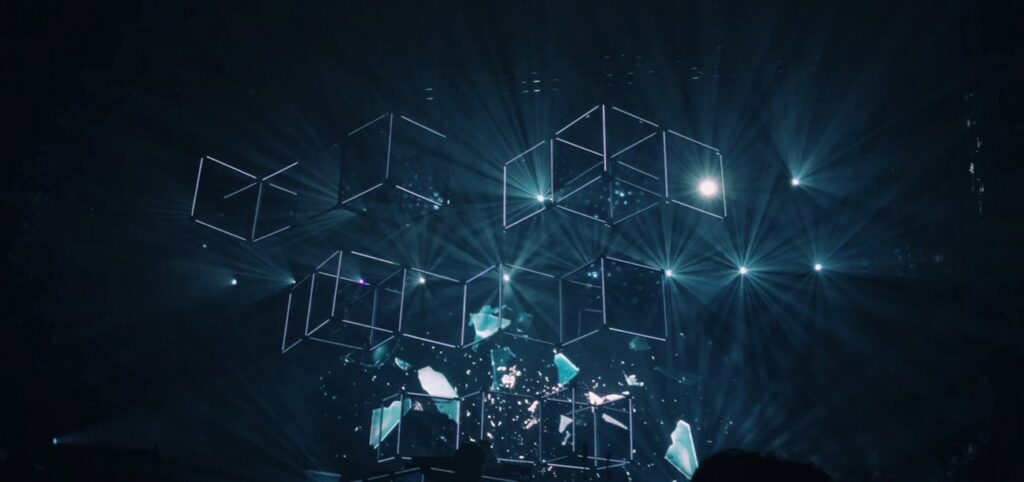
We are excited to announce that Zanders has been listed on the Swift Customer Security Programme (CSP) Assessment Providers directory*.
The CSP helps reinforce the controls protecting participants from cyberattack and ensures their effectivity and that they adhere to the current Swift security requirements.
*Swift does not certify, warrant, endorse or recommend any service provider listed in its directory and Swift customers are not required to use providers listed in the directory.
Swift Customer Security Programme
A new attestation must be submitted at least once a year between July and December, and also any time a change in architecture or compliance status occurs. Customer attestation and independent assessment of the CSCF v2023 version is now open and valid until 31 December 2023. July 2023 also marks the release of Swifts CSCF v2024 for early consultation, which is valid until 31 December 2024.
Swift introduced the Customer Security Programme to promote cybersecurity amongst its customers with the core component of the CSP being the Customer Security Controls Framework (CSCF). Independent assessment has been introduced as a prerequisite for attestation to enhance the integrity, consistency, and accuracy of attestations. Each year, Swift releases an updated version of the CSCF that needs to be attested to with support of an independent assessment.
The Attestation is a declaration of compliance with the Swift Customer Security Controls Policy and is submitted via the Swift KYC-SA tool. Dependent on the Swift Architecture used, the number of controls to be implemented vary; of which certain are mandatory, and others advisory.
Further details on the Swift CSCF can be found on their website:
- https://www.swift.com/myswift/customer-security-programme-csp
- https://www.swift.com/myswift/customer-security-programme-csp/find-external-support/directory-csp-assessment-providers
Our services
Do you have arrangements in place to complete the independent assessment required to support the attestation?
Zanders has experience with and can support the completion of an independent external assessment of your compliance to the Swift Customer Security Control Framework that can then be used to fully complete and sign-off the Swift attestation for this year.
With an extensive track record of designing and deploying bank integrations, our intricate knowledge of treasury systems across both IT architecture as well as business processes positions us well to be a trusted independent assessor. We draw on past projects and assessments to ask the right questions during the assessment phase, aligning our customers with the framework provided by Swift.
The Swift attestation can also form part of a wider initiative to further optimise your banking landscape, whether that be increasing the use of Swift within your organisation, bank rationalization or improving your existing processes. The availability of your published attestation and its possible consultation with counterparties (upon request) helps equally in performing day-to-day risk management.
Approach
Planning
We start with rigorous planning of the assessment project, developing a scope of work and planning resources accordingly. Our team of experts will work with clients to formulate an Impact Assessment based on the most recent version of the Swift Customer Security Controls Framework.
Architecture Classification
A key part of our support will be working with the client to formulate a comprehensive overview of the system architecture and identify the applicable controls dictated by the CSCF.
Perform Assessment
Using our wide-ranging experience, we will test the individual controls against specific scenarios designed to root out any weaknesses and document evidence of their compliance or where they can be improved.
Independent Assessment Report
Based on the evidence collected, we will prepare an Independent Assessment report which includes status of the compliance against individual controls, baselining them against the CSCF and recommendations for improvement areas within the system architecture.
Post Assessment Activities
Once completed, the Independent Assessment report will support you with the submission of the Attestation in line with the requirements of the CSCF version in force, which is required annually by Swift. In tandem, Zanders can deliver a plan for implementation of the recommendations within the report to ensure compliance with current and future years’ attestations. Swift expects controls compliance annually, together with the submission of the attestation by 31 December at the latest, in order to avoid being reported to your supervisor. Non-compliant status is visible to your counterparties.
Do you need support with your Swift CSP Independent Assessment?
We are thrilled to offer a Swift CSP Independent Assessment service and look forward to supporting our clients with their attestations, continuing their commitment to protecting the integrity of the Swift network, and in doing so supporting their businesses too. If you are interested in learning more about our services, please contact us directly below.
Get performance now
- Contact me
You are currently viewing a placeholder content from HubSpot. To access the actual content, click the button below. Please note that doing so will share data with third-party providers.
More Information
Machine learning in CRR-compliant IRB models
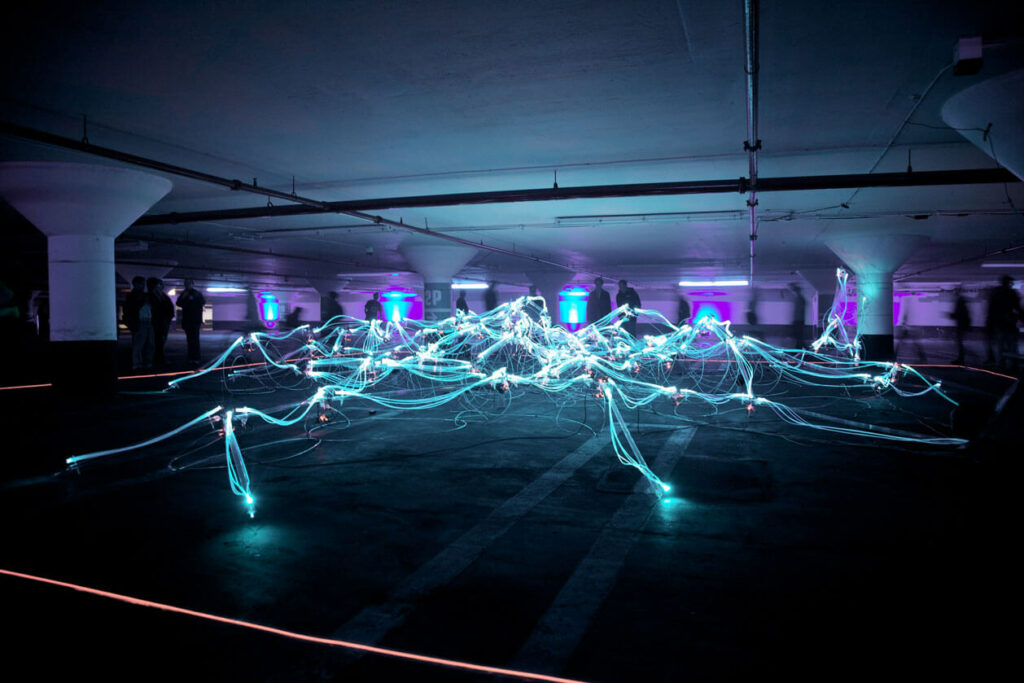
Even though machine learning is rapidly transforming the financial risk landscape, it is underused within internal ratings based-models. Why is it uncommon within this field, how will this change in the near future, and who will take the lead?
Machine learning (ML) models have proven to be highly effective in the field of credit risk,
outperforming traditional regression models in their predictive power. Thanks to the exponential growth in the data availability, storage capacity and computational power, these models can be effectively trained on vast amounts of complex, unstructured data.
Despite these advantages, however, ML models have yet to be integrated into internal rating-based (IRB) modeling methodologies used by banks. This is mainly due to the fact that existing methods for calculating regulatory capital have remained largely unchanged for over 15 years, and the complexity of ML models can make it difficult to comply with the Capital Requirements Regulation (CRR). Nonetheless, the European Banking Authority (EBA) recognizes the potential of ML in the future of IRB modeling and is considering providing a set of principle-based recommendations to ensure its appropriate use.
In sight of these recommendations, the EBA published a discussion paper (EBA/DP/2021/04) to seek stakeholders’ feedback on the practical use of ML in the context of IRB modeling, aiming to provide clarity on supervisory expectations. This paper outlines the challenges and opportunities in using ML to develop CRR-compliant IRB models, and presents the point of view for various banking stakeholders on the topic.
Current use and potential benefits of ML in IRB models
According to research conducted by the Institute of International Finance (IIF) in 2019, the most
common uses of ML within credit risk are credit approval, credit monitoring and collections, and
restructuring and recovery. However, the use of ML within other regulatory areas, such as capital
requirements, stress testing and provisioning is highly limited. For IRB models, ML is used to
complement standard models used for capital requirement calculation. Examples of current uses of ML to complement standard models include (i) model validation, where the ML model serves as a challenger model, (ii) data improvements, where ML is used for more efficient data preparation and exploration, and (iii) variable selection, where ML is used to detect explanatory variables.
ML has the potential to provide a range of benefits for risk differentiation, including improvements in the model discriminatory power 1 , identification of relevant risk drivers 2 , and optimization of the portfolio segmentation. Based on their superior predictive ability and capacity to detect bias , ML models can also help to improve risk quantification 3 . Furthermore, ML can be used to enhance the data collection and preparation process, leading to improved data quality. Finally, ML models can enable the use of unstructured data, expanding the possible data sets allowing for the use of new parameters and estimation of these parameters.
Challenges to CRR compliance
The following table summarizes the challenges involved in using ML to develop IRB models that are compliant to prudential requirements.
Area | Topic | Article ref. | Challenge |
Risk differentiation | The definition and assignment criteria to grades or pools | CRR 171(1)(a) and (b) RTS on AM for IRB 24(1) | Use of ML is constrained when no clear economic relation between the input and the output variables. Institutions should explore suitable tools to interpret complex ML models. |
Risk differentiation | Complementing human judgement | CRR 172(3) and 174(e) GL on PD and LGD 58 | Complexity in ML models may make it more difficult to take into account expert involvement and analyze the impact of human judgement on the performance of the model. |
Risk differentiation | Documentation of modeling assumptions and theory behind the model | CRR 175(1) and (2), 175(4)(a) RTS on AM for IRB 41(d) | To document a clear outline of the theory, assumptions and mathematical basis of the final assignment of estimates to grades, exposures or pools may be difficult in complex ML models. Also, the institution’s relevant staff should fully understand the model’s capabilities and limitations. |
Risk quantification | Plausibility and intuitiveness of the estimates | CRR 179(1)(a) | ML models can result in non-intuitive estimates, particularly when the structure of the model is not easily interpretable. |
Risk quantification | Underlying historical observation period | CRR 180(1)(a) and (h), 180(2)(a) and (e), and 181(1)(j) and 181(2). | For PD and LGD estimation, the minimum length of the historical observation period is five years. This can be a challenge for the use of big data, which might not be available for a sufficient time horizon. |
Validation | Interpreting and resolving validation findings | CRR 185(b) | Difficulties may arise in ML models in explaining material differences between the realized default rates and the expected range of variability of the PD estimates per grade. This also holds for assessing the effect of the economic cycle on the logic of the model. |
Validation | Validation tasks | CRR 185 | It may be more difficult to assess the representativeness and to fulfill operational data requirements (e.g. data quality and maintenance). Furthermore, the validation function is expected to challenge the model design, assumptions and methodology, whereas a more complex model will be harder to challenge efficiently. |
Governance | Corporate governance | CRR 189 | The institution’s management body is required to possess a general understanding of the rating systems of the institution and detailed comprehension of the associated management reports. |
Operational | Implementation process | CRR 144, 171 RTS on AM for IRB 11(2)(b) | The complexity of ML models may make it more difficult to verify the correct implementation of internal ratings and risk parameters in IT systems. In particular, the heavy utilization of different packages will become challenging. |
Operational | Categorization of model changes | CRR 143(3) | If models are updated at a high frequency with time-varying weights associated to variables, it may be difficult to categorize the model changes. For the validation function it is unfeasible to validate each model iteration. |
Expectations for a possible and prudent use of ML in IRB modeling
In January 2020, the EBA published a report on the recent trends of big data and advanced analytics (BD&AA) in the banking sector. In order to support ongoing technological neutrality – the freedom to choose the most appropriate technology adequate to their needs and requirements - the BD&AA report recommends the use of ML and suggests safeguards to ensure compliance. Meanwhile, the EBA has provided the following principles to clarify how to adhere to the regulatory requirements set out in the CRR for IRB models.
- All relevant stakeholders should have an appropriate level of knowledge of the model’s functioning. This includes the model development unit, credit risk control unit and validation unit, but also the management body and senior management, to a lesser extent.
- Zanders believes that appropriate trainings on the use of ML to the relevant stakeholders ensures the appropriate level of knowledge.
- Institutions should avoid unnecessary complexity in the modeling approach if it is not justified by a significant improvement in the predictive capabilities.
- Zanders advocates to focus on the use of explanatory drivers with significant predictive information to avoid including an excessive number of drivers. In addition, Zanders advises on which data type (unstructured or more conventional) and what modeling choice (simplistic or sophisticated) is appropriate for the institution to avoid unnecessary complexity.
- Institutions should ensure that the model is correctly interpreted and understood by relevant stakeholders.
- Zanders assesses the relationship of each single risk driver with the output variable, ceteris paribus, the weight of each risk driver to detect the level of influence on the model prediction, the economic relationship to ensure plausible and intuitive estimates, and the potential biases in the model, such that the model is correctly interpreted and understood.
- The application of human judgement in the development of the model and in performing overrides should be understood in terms of economic meaning, model logic, and model behavior.
- Zanders provides best market practice expertise in applying human judgement in the development and application of the model.
- The parameters of the model should generally be stable. Therefore, institutions should perform sensitivity analysis and identify/monitor reasons for regular updates.
- Zanders analyses whether a break in the economic conditions or in the institution’s processes or in the underlying data might justify a model update. Furthermore, Zanders evaluates the changes required to obtain stable parameters over a longer time horizon.
- Institutions should have a reliable validation, which covers overfitting issues, challenging the model design, reviewing representativeness and data quality issues, and analyzing the stability of estimates.
- Zanders provides validation activities following regulatory compliance.
Survey responses
The following stakeholders provided responses to the questions posed in the EBA paper (EBA/DP/2021/04):
- Asociación Española de Banca (AEB) – Spanish Banking Association
- Assilea – Italian Leasing Association
- Association for Financial Markets in Europe (AFME)
- European Association of Co-operative Banks (EACB)
- European Savings and Retail Banking Group (ESBG)
- Fédération Bancaire Française - French Banking Federation (FBF)
- Die deutsche Kreditwirtschaft – The German Banking Industry Committee (GBIC)
- Institute of International Finance (IIF)
- Mazars – audit, tax and advisory firm
- Prometeia SpA – advisory and tech solutions firm (SpA)
- Banca Intesa Sanpaolo – Italian international banking group (IIBG)
A summary of the responses to key questions is provided below.
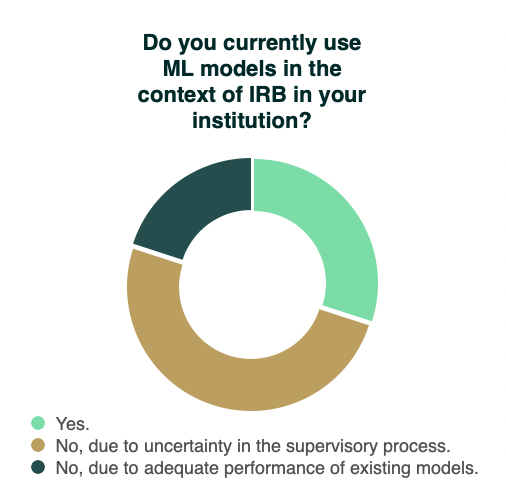
The vast majority of respondents does not currently apply ML for IRB purposes. The respondents argue that they would like to use ML for regulatory capital modeling, but that this is not deemed feasible without explicit regulatory guidelines and certainty in the supervisory process. AEB refers to the report published by the Bank of Spain in February 2021, where it was concluded that ML models perform better than traditional models in estimating the default rate, and that the potential economic benefits would be significant for financial institutions. AECB and GBIC indicate that the need for ML in regulatory capital modeling is currently not necessary as the predictive power of traditional models prove to be satisfactory. Three respondents presently use ML to some extent within IRB, such as for risk driver selection and risk differentiation. Only IIBG has actually developed a complete ML model for the estimation of PD of an SME retail portfolio, which has been validated by the supervisor in 2021.
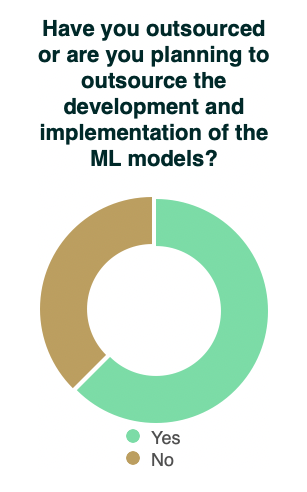
Five respondents answer that they would outsource the ML modeling for IRB to different degrees. AEB states that most of the work will be done internally and consulting services will be required at peak planning times. According to Mazars, the outsourcing is mainly performed on the development phase as banks would take over the ownership of the model and implement it in its IT infrastructure internally. The other respondents that plan to outsource foresee that external support is required for all phases. The remaining respondents state that they have not noticed any intention to outsource any of the parts or phases of the process on the development and implementation of the ML models.
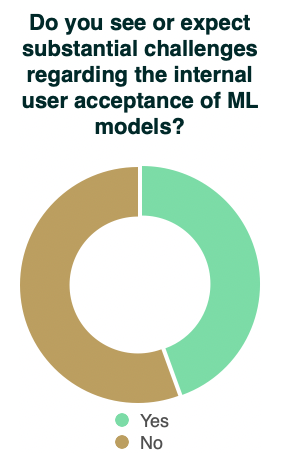
The respondents are split almost evenly on the topic of challenges regarding internal user acceptance of ML models. The respondents that see substantial challenges attribute this to the low explanatory power of ML-driven models and concerns on business representatives and credit officers being comfortable with the understanding and interactions of the standard approaches. However, for the latter it is recognized that specific trainings on ML methods are beneficial in this respect. The respondents that do not expect considerable challenges argue that ML models should be treated in the same manner as traditional methods as the same fundamental principles apply, where it is key to ensure that all Lines of Defense have the appropriate skills and responsibilities. Furthermore, the respondents state that existing ML applications, such as in AML, can be leveraged. The AFM explains that the techniques used to explain the results that are already available in those contexts are proving to be effective to understand the outcomes. SpA shares this sentiment and refers to Shapley values and the Lime test as techniques for model interpretability.
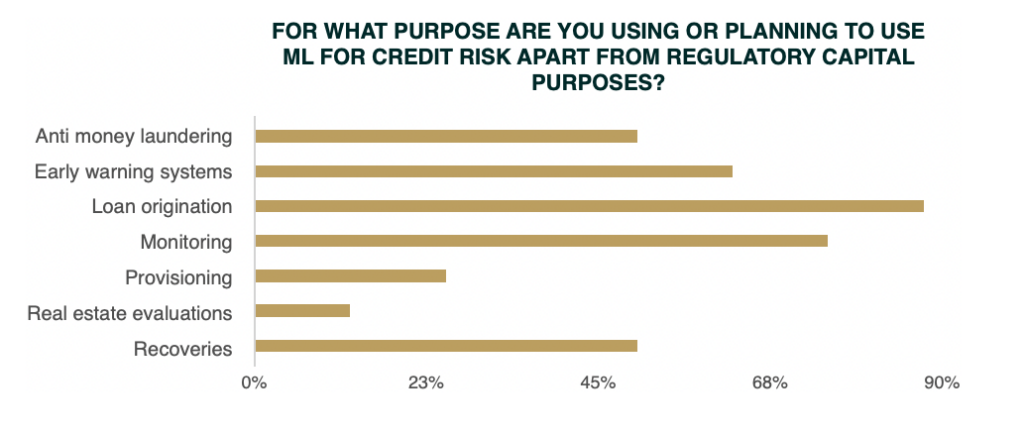
There exists a consensus among the respondents that ML is suitable for various areas within credit risk. For example, ESBG outlines that the opportunities for using ML models within the credit risk area and their advantages are endless. In particular for loan origination (admission), monitoring and early warning systems, the respondents are mutually in favor of applying ML. In general, the application of ML for these purposes is already being adopted by institutions far more than for IRB modeling.
To conclude
Banks are keen to use ML in the context of IRB modeling given the benefits achievable in both risk differentiation and risk quantification processes. The main reason for the limited use of ML in IRB modeling is the uncertainty in the supervisory process. The ball is currently in EBA’s court. The discussion paper and prospective set of principle-based recommendations to bridge the gap in institutional and regulatory expectations show EBA’s interest in making ML in IRB modeling a more common reality.
Zanders believes that institutions are best prepared for this transition by already applying ML to different fields, such as AML, application models and KYC. The EBA defines the enhancement of capacity to combat money laundering in the EU as one of its five main priorities for 2023 (EBA/REP/2022/20). This includes supporting the implementation of robust approaches to advance AML. Zanders anticipates that the technical EBA support in AML will spill over to IRB modeling in the coming three years. Zanders supports institutions in the application of ML in aforementioned fields, which will ensure that those institutions are adequately prepared to fully reap the rewards when ML in the context of IRB modeling is commonly accepted.
What can Zanders offer?
We combine deep credit risk modeling expertise with relevant experience in regulation and programming
- A Risk Advisory Team consisting of 70+ consultants with quantitative backgrounds (e.g., Econometrics and Physics)
- Strong knowledge of credit risk models
- Extensive experience with calibration and implementation of credit risk models
- We offer ready-to-use rating models, Credit Risk Academy modules and expert sessions that can be tailored to you specific needs.
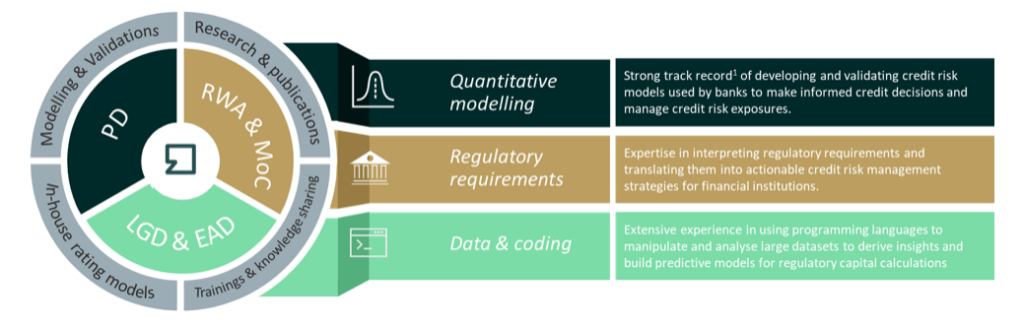
Interested in ML in credit risk, Credit Risk Academy, and other regulatory capital modeling services? Please feel free to contact Jimmy Tang or Elena Paniagua-Avila.
Footnotes
1 CRR article 170(1)(f) and (3)(c), and RTS on AM of IRB articles 36(1)(a) and 37(1)(c)
2 CRR articles 170(3)(a) and (4) and 171(2) and GL on PD and LGD paragraphs 21, 25, and 121
3 RTS on AM of IRB articles 36(1)(a) and 37(1)(c)
The CGI-MP – What’s it All About and Can it Really Deliver?
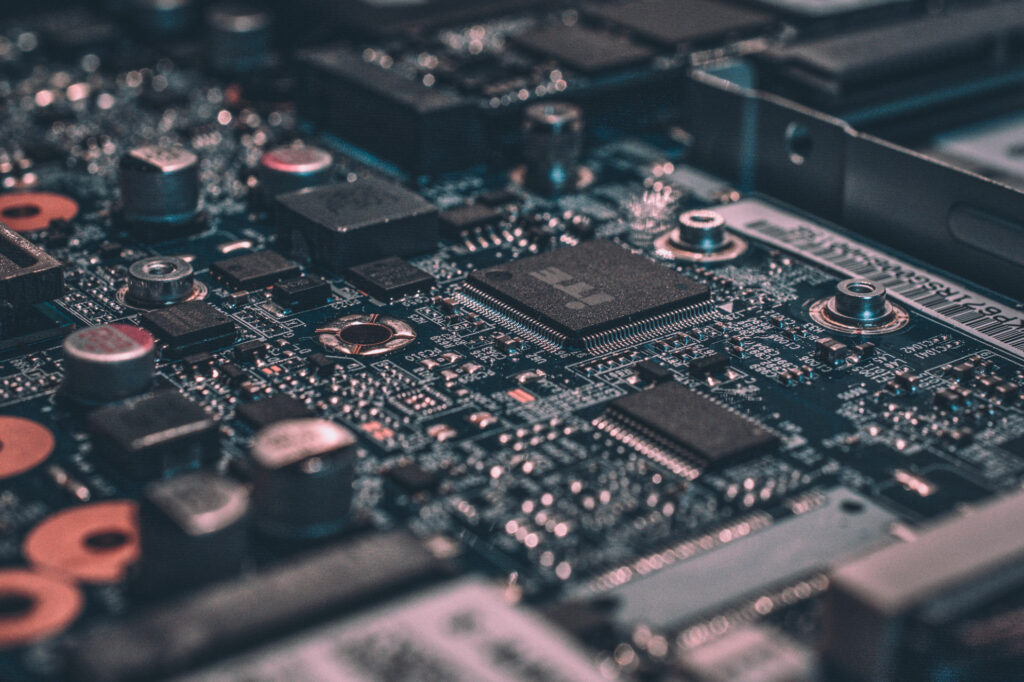
Even though machine learning is rapidly transforming the financial risk landscape, it is underused within internal ratings based-models. Why is it uncommon within this field, how will this change in the near future, and who will take the lead?
In this third article in the ISO 20022 series, Zanders experts Eliane Eysackers and Mark Sutton take a focused look at the Common Global Implementation Market Practice Group (CGI-MP). Explaining its role, objectives and how it has the potential to redefine what is possible in terms of taking the multi-banking cash management model to the next level. With the end-goal being a simplified, standardised, low cost, low maintenance multi-bank cash management architecture.
Who or What is the CGI-MP?
The CGI-MP was formed in October 2009, with Swift playing host for the inaugural meeting of an inclusive collaborative group of key stakeholders – banks, software vendors, corporates, national payment associations, that would redefine the competitive boundaries within the multi-banking cash management space. From these humble beginnings, the CGI-MP now has 355 members globally and has mobilized its domain expertise to create and publish implementation guidelines for the XML corporate payment message (pain.001.001.09) including an updated payment status workflow guide. The CGI-MP objective has always been crystal clear: "A corporate can use the same message structure for all their payments with all of their transaction banks reaching any payment system across the globe.”
What challenges existed with the original CGI-MP implementation guidelines?
Whilst the April 2009 ISO standards maintenance release provided the ideal opportunity to demonstrate the benefits of this initial collaboration through the inaugural implementation guidelines, the past 14 years have highlighted multiple friction points that existed around the corporate adoption of the version 3 XML payment message. So, despite the guidelines, we have witnessed significant divergence in terms of the banking communities implementation of this global financial messaging standard. The main challenges are summarized below:
- Inconsistent Payment Method Identification: Whilst the CGI-MP implementation guidelines recommended the use of standard codes, history reveals both the non-standard use of these codes and continued use of bank proprietary codes.
- Limited Adoption of Data Over-population: This was a core principle of the CGI-MP implementation guidelines which enabled the corporate community to establish a more generic core template. However, few banks actually embraced this core principle.
- Continued Focus on Unstructured Tags: Despite the guidance, the banking community has generally leveraged the unstructured tags to support local country rules like central bank reporting.
- Core References: Corporate implementations have revealed significant differences in the way the key batch and transaction references plus the payment details are supported and processed.
What is different about these new CGI-MP implementation guidelines?
The CGI-MP has taken these lessons learned from the version 3 implementations to try and remove the friction caused by the bank proprietary implementations. A core document within the implementation guidelines is Appendix B which is a supporting document that focuses on the additional specific local country rules. For example, which xml tags are used for central bank reporting in a specific country. This is important as it provides the opportunity to try and achieve a greater level of standardization around interpretation and therefore implementation of the XML payment message.
The most important difference is the ‘change of mindset’ that will be required from the banking community help deliver a win-win situation. So the CGI-MP is recommending a more prescriptive approach to the XML version 9 guidelines, which will help remove the numerous friction points that have been ‘called out’ above. Banks that follow the same ‘lift and shift’ logic to how they developed pain.001 V03, which typically followed the core logic of their own proprietary file formats will be missing a real opportunity to remove friction and accelerate implementations.
Considerations for Corporate Treasury?
The CGI-MP has now published the XML version 9 implementation guidelines, which CGI-MP member banks are now reviewing as part of their own XML version 9 service proposition. Based on the experience around the development and implementation of the original XML version 3 proposition back in 2009, banks are probably 6-9 months away from launch. However, whilst there is no requirement for the corporate community to migrate, XML version 9 presents a real possibility to:
- Remove friction between the banks.
- Simplify and standardise the XML version 3 implementation.
- Maximise the end to end benefits of structured data.
- Achieve greater bank portability.
In Summary
Considering the potential significant impact of the ‘change in mindset’ from the banking community, alignment to the CGI-MP guidelines and specifically Appendix B could become a table stake from a corporate perspective. It will be very important for the corporate community to have a very focused and structured discussion with their banking partners to help determine if the perceived benefits can materialise. And finally, as this is unproven territory, the recommendation remains on proceeding with banking partner harmonisation discussions to ensure the optimum implementation outcome.
FRTB: Profit and Loss Attribution (PLA) Analytics
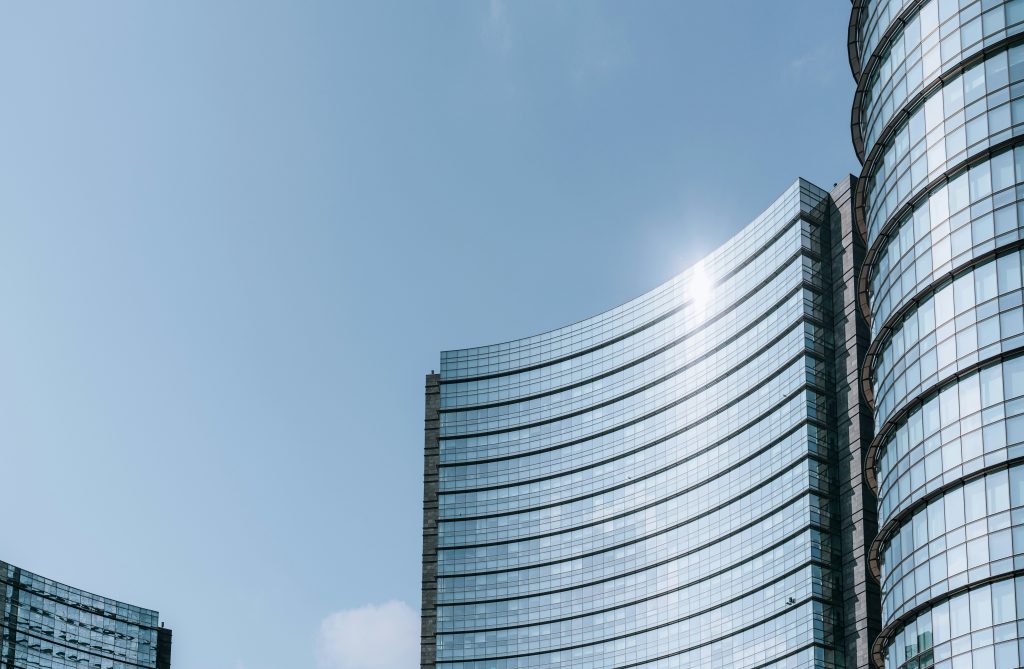
Even though machine learning is rapidly transforming the financial risk landscape, it is underused within internal ratings based-models. Why is it uncommon within this field, how will this change in the near future, and who will take the lead?
Under FRTB regulation, PLA requires banks to assess the similarity between Front Office (FO) and Risk P&L (HPL and RTPL) on a quarterly basis. Desks which do not pass PLA incur capital surcharges or may, in more severe cases, be required to use the more conservative FRTB standardised approach (SA).
What is the purpose of PLA?
PLA ensures that the FO and Risk P&Ls are sufficiently aligned with one another at the desk level. The FO HPL is compared with the Risk RTPL using two statistical tests. The tests measure the materiality of any simplifications in a bank’s Risk model compared with the FO systems. In order to use the Internal Models Approach (IMA), FRTB requires each trading desk to pass the PLA statistical tests. Although the implementation of PLA begins on the date that the IMA capital requirement becomes effective, banks must provide a one-year PLA test report to confirm the quality of the model.
Which statistical measures are used?
PLA is performed using the Spearman Correlation and the Kolmogorov-Smirnov (KS) test using the most recent 250 days of historical RTPL and HPL. Depending on the results, each desk is assigned a traffic light test (TLT) zone (see below), where amber desks are those which are allocated to neither red or green.
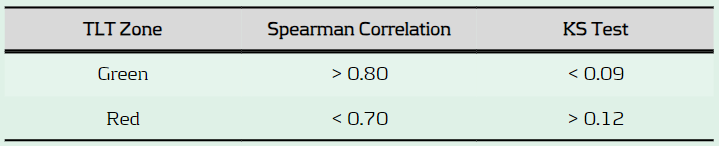
What are the consequences of failing PLA?
Capital increase: Desks in the red zone are not permitted to use the IMA and must instead use the more conservative SA, which has higher capital requirements. Amber desks can use the IMA but must pay a capital surcharge until the issues are remediated.
Difficulty with returning to IMA: Desks which are in the amber or red zone must satisfy statistical green zone requirements and 12-month backtesting requirements before they can be eligible to use the IMA again.
What are some of the key reasons for PLA failure?
Data issues: Data proxies are often used within Risk if there is a lack of data available for FO risk factors. Poor or outdated proxies can decrease the accuracy of RTPL produced by the Risk model. The source, timing and granularity also often differs between FO and Risk data.
Missing risk factors: Missing risk factors in the Risk model are a common cause of PLA failures. Inaccurate RTPL values caused by missing risk factors can cause discrepancies between FO and Risk P&Ls and lead to PLA failures.
Roadblocks to finding the sources of PLA failures
FO and Risk mapping: Many banks face difficulties due to a lack of accurate mapping between risk factors in FO and those in Risk. For example, multiple risk factors in the FO systems may map to a single risk factor in the Risk model. More simply, different naming conventions can also cause issues. The poor mapping can make it difficult to develop an efficient and rapid process to identify the sources of P&L differences.
Lack of existing processes: PLA is a new requirement which means there is a lack of existing infrastructure to identify causes of P&L failures. Although they may be monitored at the desk level, P&L differences are not commonly monitored at the risk factor level on an ongoing basis. A lack of ongoing monitoring of risk factors makes it difficult to pre-empt issues which may cause PLA failures and increase capital requirements.
Our approach: Identifying risk factors that are causing PLA failures
Zanders’ approach overcomes the above issues by producing analytics despite any underlying mapping issues between FO and Risk P&L data. Using our algorithm, risk factors are ranked depending upon how statistically likely they are to be causing differences between HPL and RTPL. Our metric, known as risk factor ‘alpha’, can be tracked on an ongoing basis, helping banks to remediate underlying issues with risk factors before potential PLA failures.
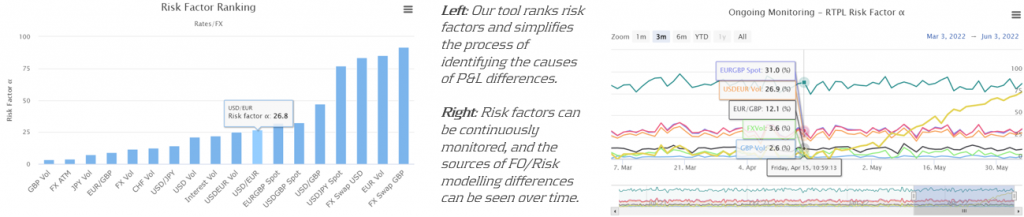
Zanders’ P&L attribution solution has been implemented at a Tier-1 bank, providing the necessary infrastructure to identify problematic risk factors and improve PLA desk statuses. The solution provided multiple benefits to increase efficiency and transparency of workstreams at the bank.
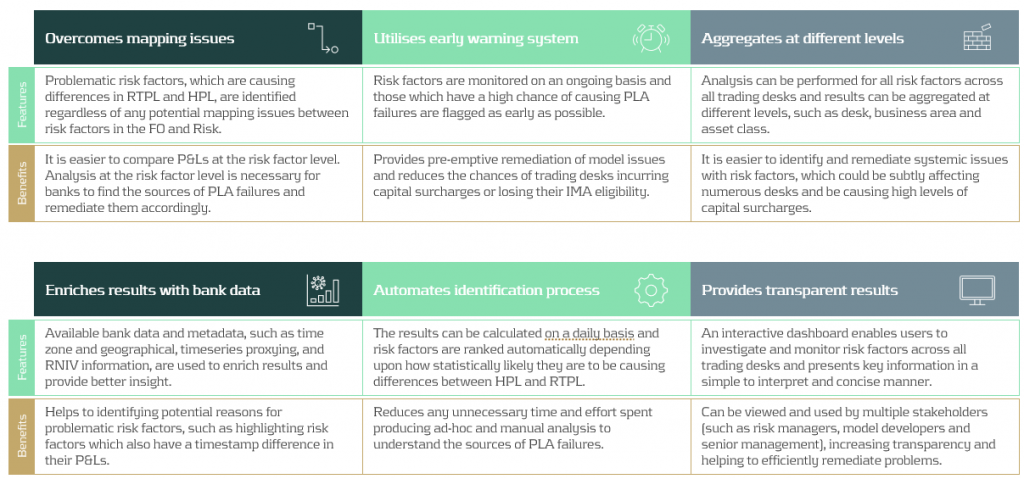
Conclusion
As it is a new regulatory requirement, passing the PLA test has been a key concern for many banks. Although the test itself is not considerably difficult to implement, identifying why a desk may be failing can be complicated. In this article, we present a PLA tool which has already been successfully implemented at one of our large clients. By helping banks to identify the underlying risk factors which are causing desks to fail, remediation becomes much more efficient. Efficient remediation of desks which are failing PLA, in turn, reduces the amount of capital charges which banks may incur.
Cryptocurrencies and Blockchain: Navigating Risk, Compliance, and Future Opportunities in Corporate Treasury
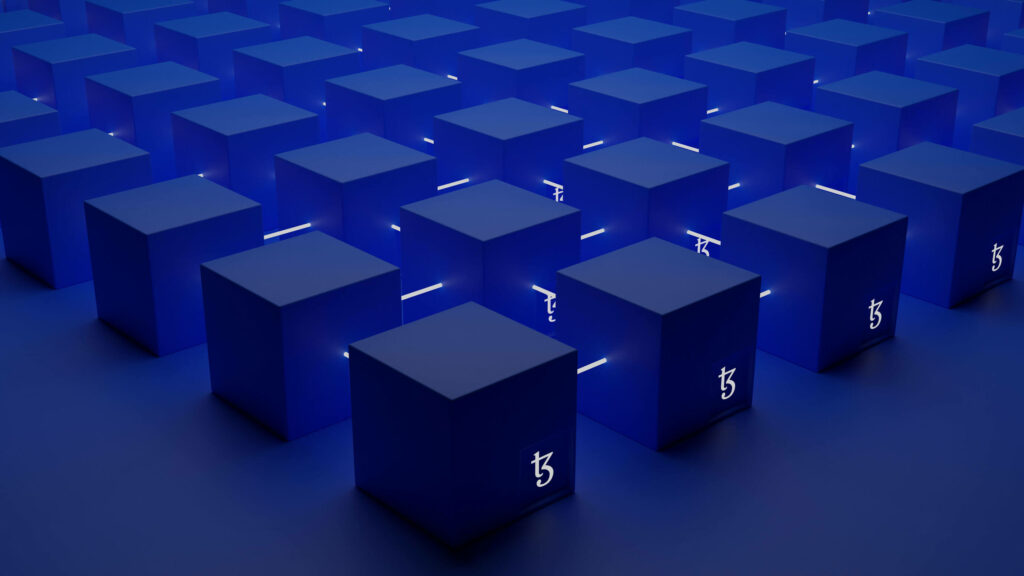
Even though machine learning is rapidly transforming the financial risk landscape, it is underused within internal ratings based-models. Why is it uncommon within this field, how will this change in the near future, and who will take the lead?
As a result of the growing importance of this transformative technology and its applications, various regulatory initiatives and frameworks have emerged, such as Markets in Crypto-Assets Regulation (MiCAR), the Distributed Ledger Technology (DLT) Pilot Regime, and the Basel Committee on Banking Supervision (BCBS) crypto standard were launched, demonstrating the growing importance and adoption at both a global and national level. Given these trends, treasuries will be impacted by Blockchain one way or the other – if they aren’t already.
With the advent of cryptocurrencies and digital assets, it is important for treasurers to understand the issues at hand and have a strategy in place to deal with them. Based on our experience, typical questions that a treasurer faces are how to deal with the volatility of cryptocurrencies, how cryptocurrencies impact FX management, the accounting treatment for cryptocurrencies as well as KYC considerations. These developments are summarized in this article.
FX Risk Management and Volatility
History has shown that cryptocurrencies such as Bitcoin and Ether are highly volatile assets, which implies that the Euro value of 1 BTC can fluctuate significantly. Based on our experience, treasurers opt to sell their cryptocurrencies as quickly as possible in order to convert them into fiat currency – the currencies that they are familiar and which their cost basis is typically in. However, other solutions exist such as hedging positions via derivatives traded on regulated financial markets or conversions into so-called stablecoins1.
Accounting Treatment and Regulatory Compliance
Cryptocurrencies, including stablecoins, require careful accounting treatment and compliance with regulations. In most cases cryptocurrencies are classified as “intangible assets” under IFRS. For broker-traders they are, however, classified as inventory, depending on the circumstances. Inventory is measured at the lower of cost and net realizable value, while intangible assets are measured at cost or revaluation. Under GAAP, most cryptocurrencies are treated as indefinite-lived intangible assets and are impaired when the fair value falls below the carrying value. These impairments cannot be reversed. CBDCs, however, are not considered cryptocurrencies. Similarly, and the classification of stablecoins depends on their status as financial assets or instruments.
KYC/KYT Considerations
The adoption of cryptocurrencies and Blockchain technology introduces challenges for corporate treasurers in verifying counterparties and tracking transactions. When it comes to B2C transactions, treasurers may need to implement KYC (Know Your Customer) processes to verify the age and identity of individuals, ensuring compliance with age restrictions and preventing under-aged purchases, among other regulatory requirements. Whilst the process differs for B2B (business-to-business) transactions, the need for KYC exists nevertheless. However in the B2B space, the KYC process is less likely to be made more complex by transactions done in cryptocurrencies, since the parties involved are typically well-established companies or organizations with known identities and reputations.
Central Bank Digital Currencies
(CBDCs) are emerging as potential alternatives to privately issued stablecoins and other cryptocurrencies. Central banks, including the European Central Bank and the Peoples Banks of China, are actively exploring the development of CBDCs. These currencies, backed by central banks, introduce a new dimension to the financial landscape and will be another arrow in the quiver of end-customers – along with cash, credit and debit cards or PayPal. Corporate treasurers must prepare for the potential implications and opportunities that CBDCs may bring, such as changes in payment options, governance processes, and working capital management.
Adapting to the Future
Corporate treasurers should proactively prepare for the impact of cryptocurrencies and Blockchain technology on their business operations. This includes educating themselves on the basics of cryptocurrencies, stablecoins, and CBDCs, and investigating how these assets can be integrated into their treasury functions. Understanding the infrastructure, processes, and potential hedging strategies is crucial for treasurers to make informed decisions regarding their balance sheets. Furthermore, treasurers must evaluate the impact of new payment options on working capital and adjust their strategies accordingly.
Zanders understands the importance of keeping up with emerging technologies and trends, which is why we offer a comprehensive range of Blockchain services. Our Blockchain offering covers supporting our clients in developing their Blockchain strategy including developing proofs of concept, cryptocurrency integration into Corporate Treasury, support on vendor selection as well as regulatory advice. For decades Zanders has helped corporate treasurers navigate the choppy seas of change and disruption. We are ready to support you during this new era of disruption, so reach out to us today.
Meet the team
Zanders already has a well-positioned, diversified Blockchain team in place, consisting of Blockchain developers, Blockchain experts and business experts in their respective fields. In the following you will find a brief introduction of our lead Blockchain consultants.
ISO 20022 XML (Pain.001.001.09) – Introduction of the Structured Address
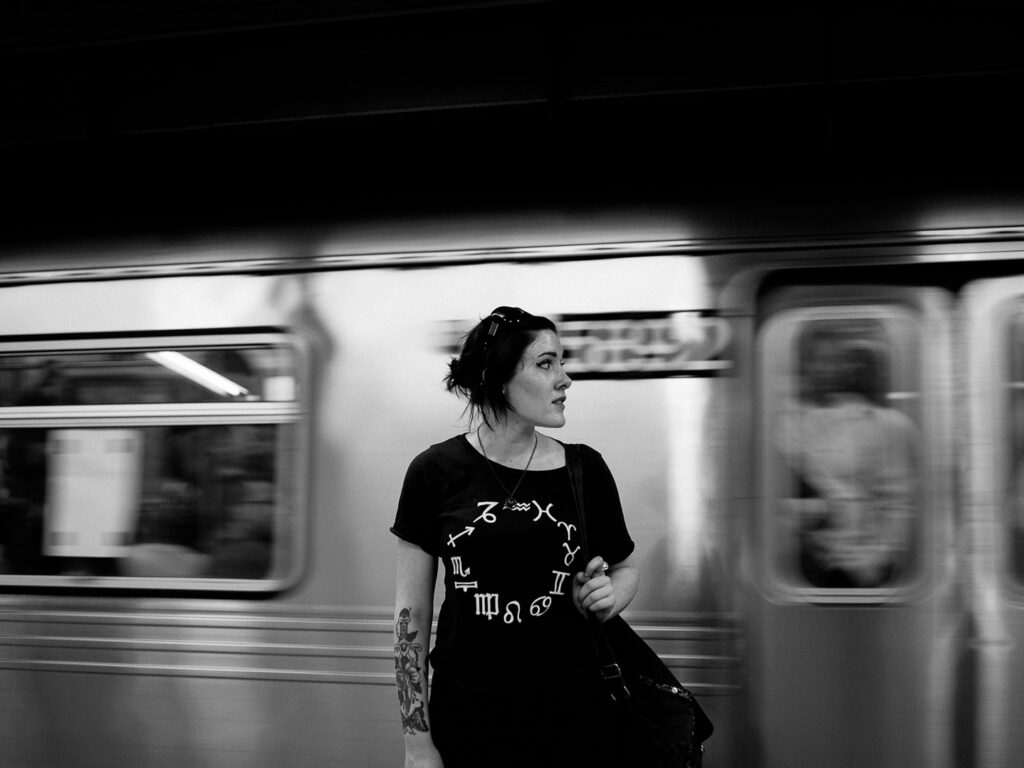
Even though machine learning is rapidly transforming the financial risk landscape, it is underused within internal ratings based-models. Why is it uncommon within this field, how will this change in the near future, and who will take the lead?
However, possibly the most important point for corporates to be aware of is the planned move towards explicit use of the structured address block. In this second article in the ISO 20022 series, Zanders experts Eliane Eysackers and Mark Sutton provide some valuable insights around this industry requirement, the challenges that exists and an important update on this core topic.
What is actually happening with the address information?
One of the key drivers around the MT-MX migration are the significant benefits that can be achieved through the use of structured data. E.g., stronger compliance validation and support STP processing. The SWIFT PMPG1 (Payment Market Practice Group) had advised that a number of market infrastructures2 are planning to mandate the full structured address with the SWIFT ISO migration. The SWIFT PMPG had also planned to make the full structured address mandatory for the interbank messages – so effectively all cross-border payments. The most important point to note is that the SWIFT PMPG had also advised of the plan to reject non-compliant cross border payment messages from November 2025 in line with the end of the MT-MX migration. So, if a cross border payment did not include a full structured address, the payment instruction would be rejected.
What are the current challenges around supporting a full structured address?
Whilst the benefits of structured data are broadly recognised and accepted within the industry, a one size approach does not always work, and detailed analysis conducted by the Zanders team revealed mandating a full structured address would create significant friction and may ultimately be unworkable.
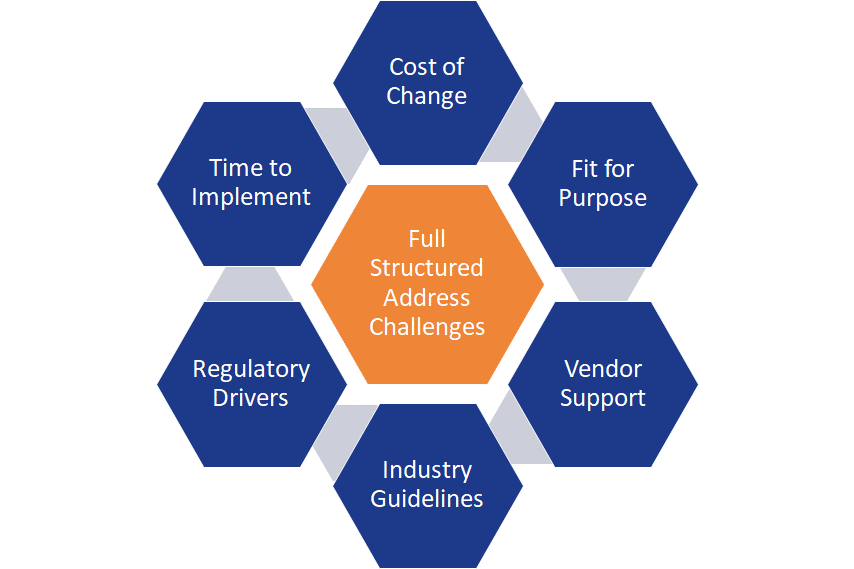
Diagram 1: Challenges around the implementation of the full structured address.
From the detailed analysis performed by the Zanders team, we have identified multiple problems that are all interconnected, and need to be addressed if the industry is to achieve its stated objective of a full structured address. These challenges are summarized below:
- Cost of change: The 2021 online TMI poll highlighted that 70% of respondents confirmed they currently merge the building name, building number, and street name in the same address line field. The key point to note is that the data is not currently separated within the ERP (Enterprise Resource Planning) system. Furthermore, 52% of these respondents highlighted a high impact to change this data, while 26% highlighted a medium impact. As part of Zanders’ continued research, we spoke to two major corporates to gain a better sense of their concerns. Both provided a high-level estimate of the development effort required for them to adapt to the new standard: ½ million euros.
- Fit for Purpose: From the ISO 20022 expert group discussions, it was recognized that the current XML Version 9 message would need a significant re-design to support the level of complexity that exists around the address structure globally.
- Vendor Support: Whilst we have not researched every ERP and TMS (Treasury Management System) system, if you compare the current structured address points including field length in the XML Version 9 message with the master data records currently available in the ERP and TMS systems, you will see gaps in terms of the fields that are supported and the actual field length. This means ERP and TMS software vendors will need to update the current address logic to fully align with the ISO standard for payments – but this software development cannot logically start until the ISO address block has been updated to avoid the need for multiple software upgrades.
- Industry Guidelines: Whilst industry level implementation guidelines are always a positive step, the current published SWIFT PMPG guidelines have primarily focused on the simpler mainstream address structures for which the current address structure is fine. By correctly including the more complex local country address options, it will quickly highlight the gaps that exist, which mean compliance by the November 2025 deadline looks unrealistic at this stage.
- Regulatory Drivers: At this stage, there is still no evidence that any of the in-country payments regulators have actually requested a full structured address. However, we have seen some countries start to request minimum address information (but not structured due to the MT file format), such as Canada and US.
- Time to Implement: We must consider the above dependencies that need to be addressed first before full compliance can logically be considered, which means a new message version would be required. Whilst industry discussions are ongoing, the next ISO maintenance release is November 2023, which will result in XML version 13 being published. If we factor in time for banks to adopt this new version (XML Version 13), time for software vendors to develop the new full structured address including field length and finally, for the corporates to then implement this latest software upgrade and test with their banking partners, the November 2025 timeline looks unrealistic at this point in time.
A very important update
Following a series of focused discussions around the potential address block changes to the XML Version 9 message, including the feedback from the GLEIF3 the ISO payment expert group questioned the need to support a significant redesign of the address block to enable the full structured address to be mandated. The Wolfsberg Group4 also raised concerns about scale of the changes required within the interbank messaging space.
Given this feedback, the SWIFT PMPG completed a survey with the corporate community in April. The survey feedback highlighted a number of the above concerns, and a change request has now been raised with the SWIFT standards working group for discussion at the end of June. The expectation is that the mandatory structured address elements will now be limited to just the Town/City, Postcode, and country, with typical address line 1 complexity continuing to be supported in the unstructured address element. This means a blended address structure will be supported.
Is Corporate Treasury Impacted by this structured address compliance requirement?
There are a number of aspects that need to be considered in answering this question. But at a high level, if you are currently maintaining your address data in a structured format within the ERP/TMS and you are currently providing the core structured address elements to your banking partners, then the impact should be low. However, Zanders recommends each corporate complete a more detailed review of the current address logic as soon as possible, given the current anticipated November 2025 compliance deadline.
In Summary
The ISO 20022 XML financial messages offer significant benefits to the corporate treasury community in terms more structured and richer data combined with a more globally standardised design. The timing is now right to commence the initial analysis so a more informed decision can be made around the key questions.
Notes:
- The PMPG (payment market practice group) is a SWIFT advisory group that reports into the Banking Services Committee (BSC) for all topics related to SWIFT.
- A Market Infrastructure is a system that provides services to the financial industry for trading, clearing and settlement, matching of financial transactions, and depository functions. For example, in-country real-time gross settlement (RTGS) operators (FED, ECB, BoE).
- Global Legal Entity Identifier Foundation (Established by the Financial Stability Board in June 2014, the GLEIF is tasked to support the implementation and use of the Legal Entity Identifier (LEI).
- https://www.wolfsberg-principles.com/sites/default/files/wb/pdfs/wolfsberg-standards/1.%20Wolfsberg-Payment-Transparency-Standards-October-2017.pdf
Grip on your EVE SOT
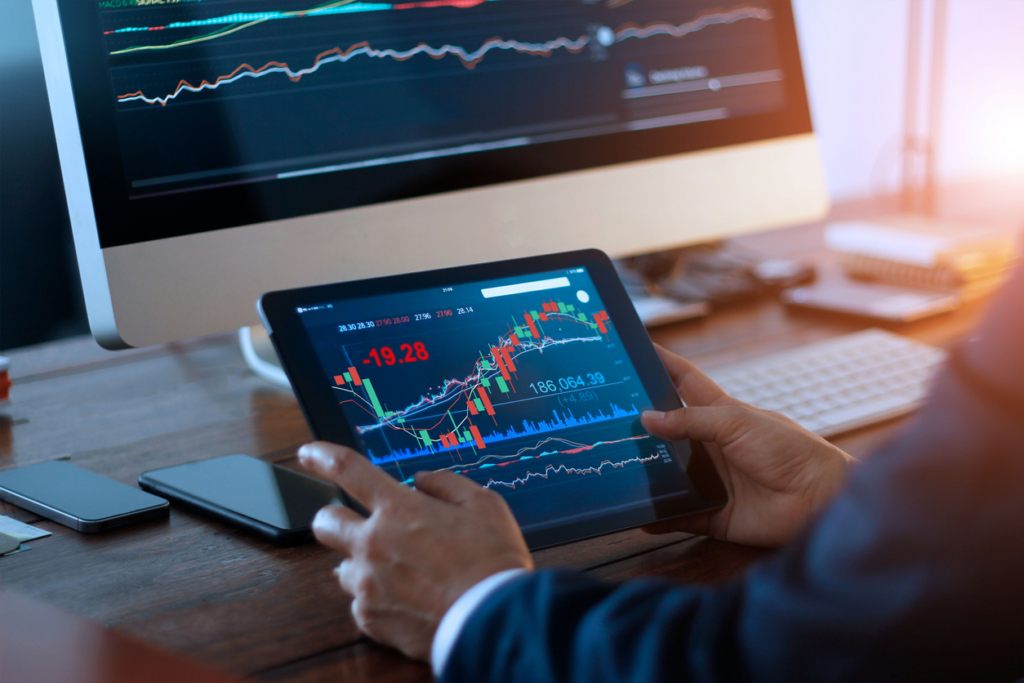
Even though machine learning is rapidly transforming the financial risk landscape, it is underused within internal ratings based-models. Why is it uncommon within this field, how will this change in the near future, and who will take the lead?
Over the past decades, banks significantly increased their efforts to implement adequate frameworks for managing interest rate risk in the banking book (IRRBB). These efforts typically focus on defining an IRRBB strategy and a corresponding Risk Appetite Statement (RAS), translating this into policies and procedures, defining the how of the selected risk metrics, and designing the required (behavioral) models. Aspects like data quality, governance and risk reporting are (further) improved to facilitate effective management of IRRBB.
Main causes of volatility in SOT outcomes
The severely changed market circumstances evidence that, despite all efforts, the impact on the IRRBB framework could not be fully foreseen. The challenge of certain banks to comply with one of the key regulatory metrics defined in the context of IRRBB, the SOT on EVE, illustrates this. Indeed, even if regularities are assumed, there are still several key model choices that turn out to materialize in today’s interest rate environment:
- Interest rate dependency in behavioral models: Behavioral models, in particular when these include interest rate-dependent relationships, typically exhibit a large amount of convexity. In some cases, convexity can be (significantly) overstated due to particular modeling choices, in turn contributing to a violation of the EVE SOT criterium. Some (small and mid-sized) banks, for example, apply the so-called ‘scenario multipliers’ and/or ‘scalar multipliers’ defined within the BCBS-standardized framework for incorporating interest rate-dependent relationships in their behavioral models. These multipliers assume a linear relationship between the modeled variable (e.g., prepayment rate) and the scenario, whereas in practice this relationship is not always linear. In other cases, the calibration approach of certain behavioral models is based on interest rates that have been decreasing for 10 to 15 years, and therefore may not be capable to handle a scenario in which a severe upward shock is added to a significantly increased base case yield curve.
- Level and shape of the yield curve: Related to the previous point, some behavioral models are based on the steepness (defined as the difference between a ‘long tenor’ rate and a ‘short tenor’ rate) of the yield curve. As can be seen in Figure 1, the steepness changed significantly over the past two years, potentially leading to a high impact associated with the behavioral models that are based on it. Further, as illustrated in Figure 2, the yield curve has flattened over time and recently even turned into an inverse yield curve. When calculating the respective forward rates that define the steepness within a particular behavioral model, the downward trend of this variable that results due to the inverse yield curve potentially aggravates this effect.
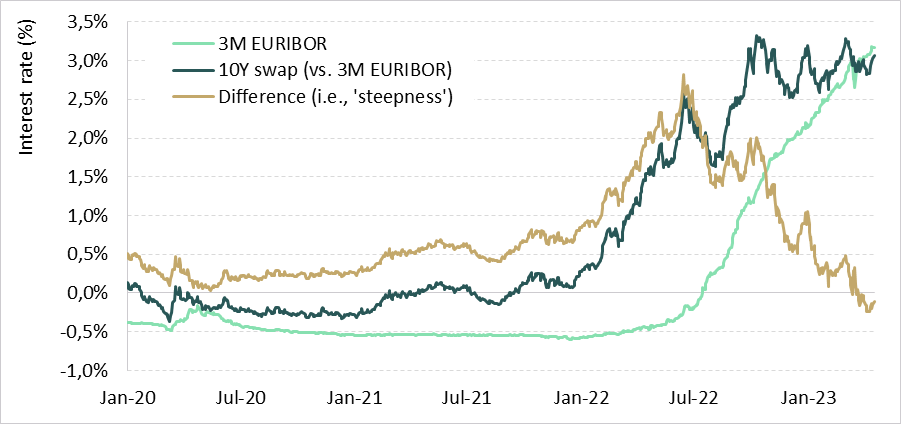
Figure 1: Development of 3M EURIBOR rate and 10Y swap rate (vs. 3M EURIBOR) and the corresponding 'Steepness'
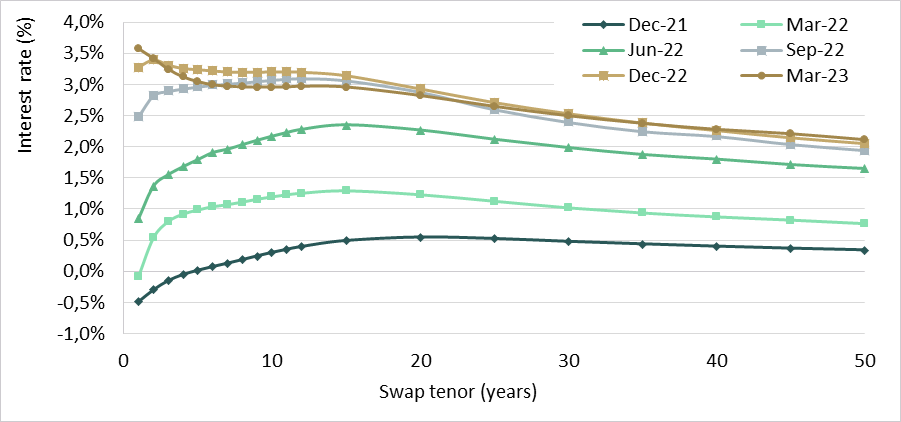
Figure 2: Development of the yield curve over the period December 2021 to March 2023.
- Hidden vulnerability to ‘down’ scenarios: Previously, the interest rates were relatively close to, or even below, the EBA floor that is imposed on the SOT. Consequently, the ‘at-risk’ figures corresponding to scenarios in which (part of) the yield curve is shocked downward, were relatively small. Now that interest rates have moved away from the EBA floor, hidden vulnerability to ‘down’ scenarios become visible and likely the dominating scenario for the SOT on EVE.
- Including ‘margin’ cashflows: Some banks determine their SOT on EVE including the margin cashflows (i.e., the spread added to the swap rate), while discounting at risk-free rates. While this approach is regulatory compliant, the inclusion of margin cashflows leads to higher (shocked) EVE values, and potentially leads to, or at least contributes to, a violation of the EVE threshold.
What can banks do?
Having identified the above issues, the question arises as to what measures banks should consider. Roughly speaking, two categories of actions can be distinguished. The first category encompasses actions that resolve an inadequate reflection of the actual risk. Examples of such actions include:
- Identify and re-solve unintended effects in behavioral models: As mentioned above, behavioral models are key to determine appropriate EVE SOT figures. Next to revisiting the calibration approach, which typically is based on historical data, banks should assess to what extent there are unintended effects present in their behavioral models that adversely impact convexity and lead to unrepresentative sensitivities and unreliably shocked EVE values.
- Adopt a pure IRR approach: An obvious candidate action for banks that still include margins in their cashflows used for the EVE SOT, is to adopt a pure interest rate risk view. In other words, align the cashflows with its discounting. This requires an adequate approach to remove the margin components from the interest cashflows.
The second category of actions addresses the actual, i.e., economic, risk position of bank. One could think of the following aspects that contribute to steering the EVE SOT within regulatory thresholds:
- Evaluate target mismatch: As we wrote in our article ‘What can banks do to address the challenges posed by rising interest rates’, a bank’s EVE is most likely negatively affected by the rise in rates. The impact is dependent on the duration of equity taken by the bank: the higher the equity duration, the larger the decline in EVE when rates rise (and hence a higher EVE risk). In light of the challenges described above, a bank should consider re-evaluating the target mismatch (i.e. the duration of equity).
- Consider swaptions as an additional hedge instrument: Convexity, in essence, cannot be hedged with plain vanilla swaps. Therefore, several banks have entered into ‘far out of the money’ swaptions to manage negative convexity in the SOT on EVE. From a business perspective, these swaptions result in additional, but accepted costs and P&L volatility. In case of an upward-sloping yield curve, the costs can be partly offset since the bank can increase its linear risk position (increase duration), without exceeding the EVE SOT threshold. This being said, swaptions can be considered a complex instrument that presents certain challenges. First, it requires valuation models – and expertise on these models – to be embedded within the organization. Second, setting up a heuristic that adequately matches the sensitivities of the swaptions to those of the commercial products (e.g., mortgages) is not a straightforward task.
How can Zanders support?
Zanders is thought leader in supporting banks with IRRBB-related topics. We enable banks to achieve both regulatory compliance and strategic risk goals, by offering support from strategy to implementation. This includes risk identification, formulating a risk strategy, setting up an IRRBB governance and framework, policy or risk appetite statements. Moreover, we have an extensive track record in IRRBB and behavioral models, hedging strategies, and calculating risk metrics, both from a model development as well as a model validation perspective.
Are you interested in IRRBB related topics? Contact Jaap Karelse, Erik Vijlbrief (Netherlands, Belgium and Nordic countries) or Martijn Wycisk (DACH region) for more information.
SAP Analytics Cloud – Liquidity Planning in SAC
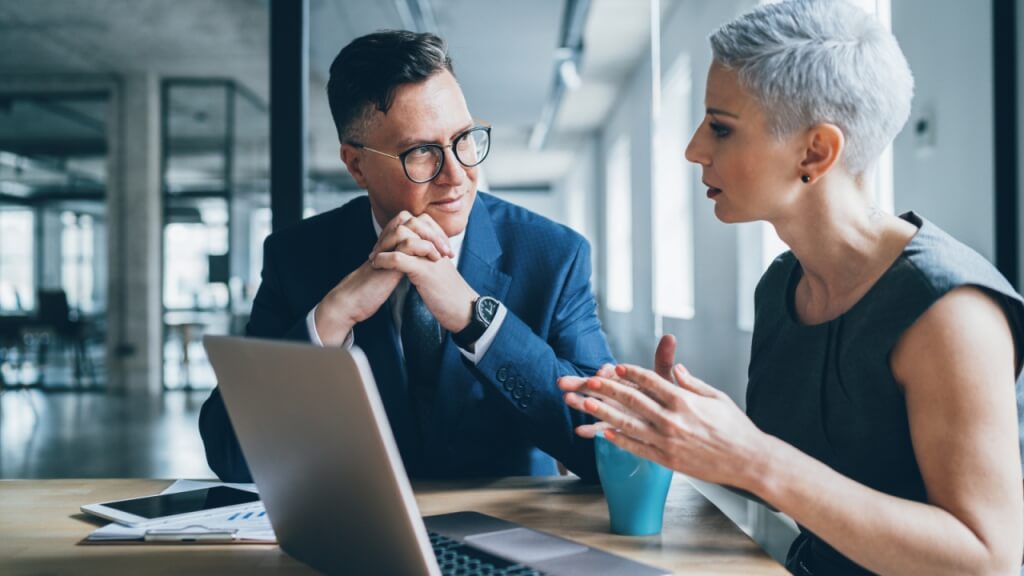
Liquidity planning in SAP Analytics Cloud (SAC) is quite likely SAP’s response to the modernization of Cash Flow Forecasting (CFF) in Corporate Treasury, a key area in today’s treasury trends.
While SAC is a planning tool to be considered, it requires further exploration to evaluate its fit with business requirements and how it could unlock opportunities to potentially streamline the CFF process across the organization. For the organizations already using SAP BPC (Business Process & Consolidation) for planning, SAC could be seen as another ‘kid on the block’. It is important for them to have a clear business case for SAC.
In this article, we introduce SAC liquidity planning solution focusing on its integrated and predictive planning capabilities. We explain the concerns of corporate treasuries that have invested heavily in SAP BPC (either as standalone instance or embedded on S/4 HANA) and discuss the business case for SAC under different scenarios of extending the BPC planning solution to SAC.
What is SAC?
SAC is the analytics and planning solution within SAP Business Technology Platform which brings together analytics and planning with unique integration to SAP applications and smooth access to heterogeneous data sources.
Among the key benefits of SAC are Extended Planning & Analysis (xP&A) and Predictive Planning based on machine learning models. While xP&A integrates (traditional) financial and operational planning resulting in one connected plan that also meets the needs of operational departments, predictive planning augments decision making through embedded AI & ML capabilities.
Extended Planning & Analysis (xP&A)
Historically, the corporate planning has been typically biased towards finance and it was quite inadequate for operational departments who have to resort to local planning in silos for their own decision making. The xP&A approach goes beyond finance and integrates strategic, financial and operational planning in one connected plan. Planning under xP&A also moves beyond budgeting and rolling forecasts to a more agile and collaborative planning process which is near real-time with faster reaction times.
SAC can be seen as the technology enabler for xP&A, as follows:
- It brings together financial, supply chain, and workforce planning in one connected plan deployed in the cloud;
- Unified planning content with a single version of the truth that everyone is working on (plans are fit for purpose for the individual departments and at the same time integrated into one connected plan);
- Predictive AI and ML models enables more realistic forecasts and facilitate near real-time planning & forecasting;
- Smart analytics features like scenario planning prepare for contingencies and quick reaction;
- SAC integrates with a wide range of data sources like S/4HANA , SuccessFactors, SAP IBP, Salesforce etc.
Predictive Planning
SAC Predictive planning model produces forecasts based on time series. The model is trained with historic data where a statistical algorithm will learn from the data set, i.e. find trends, seasonal variations and fluctuations that characterize the target variable. Upon completion of training, the model produces the forecast as the detailed time series chart for each segment.
In addition to using historical costs to make a prediction, influencer variables can be used to improve the predictive forecasts. Some examples of influencers are energy price when forecasting the energy cost, weather on the day and workday/weekend classification when forecasting daily bike hires.
Influencers are part of the data set and when included in the forecasting model, they contribute towards determining the ‘trend’. They improve the predictive forecasts which can be measured by drop in MAPE (Mean Absolute Percentage Error) and a smaller confidence interval (difference between the Error Max and the Error Min).
Business cases for extension of SAP BPC to SAC
While it may be easier to evaluate the benefits of SAC in a greenfield implementation, it could be challenging to create a suitable business case for customers already using SAP BPC, either standalone or embedded, and interesting to understand how they can preserve their existing assets in a brownfield implementation.
Below we have provided the business case for the 3 planning scenarios for extending the BPC planning to SAC:
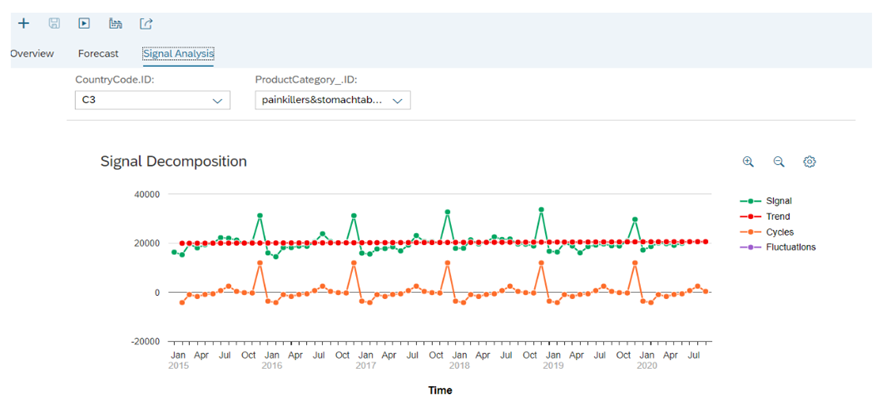
Figure: Decomposition of forecast into trend, cycle and fluctuation (left out after trend and cycle are extracted)
Scenario 1: Move planning use cases from BPC to SAC
The ‘Move’ scenario involves re-creation of planning models in SAC. Some of the existing work can be leveraged on; for example, a planning model in SAC can be built through a query coming out of SAP BPC which re-creates the planning dimensions with all their hierarchies. The planning scenarios require more effort as the planning use cases are realized in a different way in SAC than BPC. Functionalities such as disaggregations, version management and simulation are also conceptually different in SAC (compared to BPC).
Note: The re-creation of planning models in SAC through queries from BPC are more relevant for the embedded BPC model, running on SAP BW belonging to S/4 HANA NetWeaver. The integration under a standalone BPC model (using a standalone SAP BW) may not be supported and should be investigated with the vendor.
The key value drivers for this scenario include all the benefits of SAC, such as predictive planning and machine learning, xP&A, modern UX and smart analytics. SAC features on version control, real-time data analysis, lower maintenance cost and faster performance are also possible in the SAP BPC embedded model (advantages over BPC standalone model only).
Scenario 2: Complement existing BPC planning with SAC as planning UX
Here, SAC is used as a tool for entering planning data and data analysis. Data entered in SAC (e.g. via data entry form) is persisted directly in the SAP BPC planning model. LIVE planning is supported only in SAP BPC embedded model (one of the benefits of BPC embedded over standalone).
The key value drivers for this scenario are modern UX and smart analytics (from the firstt scenario), while the functional plans are maintained in BPC.
Scenario 3: Extend BPC with SAC for new functional plans
In this scenario, new functional planning is done in SAC on top of BPC, data is stored in both systems and replicated in both directions.
Key value drivers for this scenario are predictive planning and xP&A (to some extent). A specific use case for the first one is where planning data is brought into SAC, predictive forecasting applied on top of the planned data together with any manual adjustments and data is replicated back to BPC. A use case for the second one is where financial planning done in BPC is integrated with the operational planning done exclusively in SAC.
In conclusion
SAC can be seen as SAP’s vision for Extensible Planning & Analysis (xP&A) as it unifies planning across multiple lines of business while ensuring that plans are meaningful and can be put to action. The predictive time series, AI and ML based models of SAC are key enablers for a near real-time and driver-based planning.
For customers already using BPC (standalone or embedded on S/4 HANA) there are possibilities to complement the existing planning process through SAC. However, to exploit the full benefits in xP&A, it is important to understand the integrated planning approach of SAC which is conceptually different from BPC. While the immediate requirement in a BPC complement scenario could be to realize the current use cases in SAC (in a different way), it makes sense to maintain a strategic outlook, e.g. when creating the planning models in SAC, to achieve the full transformation in future.
References:
Top 10 reasons to move from SAP Business Planning and Consolidation to SAP Analytics Cloud
White-Paper-Extended-Planning-and-Analysis-2022.pdf (fpa-trends.com)
Extended Planning and Analysis | xP&A (sap.com)
SAP Analytics Cloud | BI, Planning, and Predictive Analysis Tools
Hands-On Tutorial: Predictive Planning | SAP Blogs
Predictive Planning – How to use influencers | SAP Blogs
Complement Your SAP Business Planning and Consolidation with SAP Analytics Cloud | SAP Blogs
SAP Business Planning & Consolidation for S/4HANA – In a Nutshell | SAP Blogs
Preventing a next bank failure like Credit Suisse: More capital is not the solution
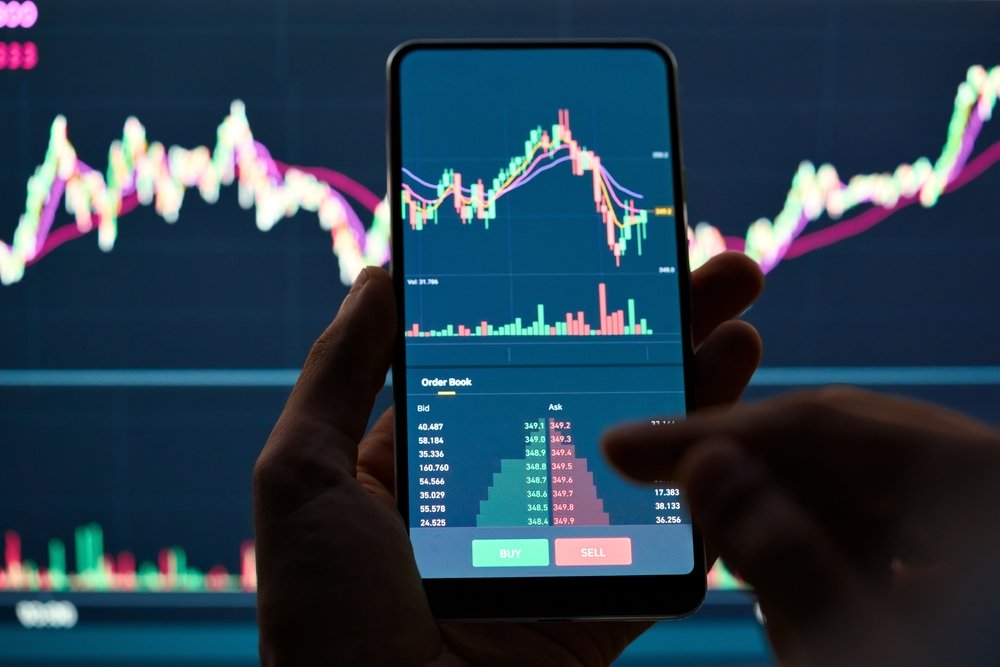
Liquidity planning in SAP Analytics Cloud (SAC) is quite likely SAP’s response to the modernization of Cash Flow Forecasting (CFF) in Corporate Treasury, a key area in today’s treasury trends.
After the collapse of Credit Suisse and the subsequent orchestrated take-over by UBS, there are widespread calls for increasing capital requirements for too big too fail banks to prevent future defaults of such institutions. However, more capital will not prevent the failure of a bank in a bank-run like Credit Suisse experienced in the first quarter of 2023.
A solid capital base is clearly important for a bank to maintain the trust of its clients, counterparties and lenders, including depositors. At the end of 2022, Credit Suisse had a BIS Common Equity Tier 1 (CET1) capital ratio of 14.1% and a CET1 leverage ratio of 5.4%, in line with its peers and well above regulatory minimum requirements. For example, UBS had a CET1 capital and leverage ratio of 14.2% and 4.4%, respectively. Hence, the capital situation by itself will not have been the reason that depositors and lenders lost trust in the bank, withdrew money in large amounts, refrained from rolling over maturing funding and/or asked for additional collateral.
Why capital does not help
Already during 2022, Credit Suisse experienced a large decrease in funding from customer deposits, falling by CHF 160 billion from CHF 393 billion to CHF 233 billion during the year. In the first quarter of 2023, a further CHF 67 billion of customer deposits were withdrawn. Even if capital could be used to cope with funding outflows (which it cannot, as we will clarify shortly), the amount will never be sufficient to cope with outflows of such magnitude. For comparison, at the end of 2021, Credit Suisse’s CET1 capital was equal to CHF 38.5 billion.
But, as mentioned, capital does not help to cope with funding outflows. A reduction in funding (liabilities) must be either replaced with new funding from other lenders or by a corresponding reduction in assets (e.g., cash or investments), leaving the amount of capital (equity) in principle unchanged[1]. If large amounts of funding are withdrawn at the same time, as was the case for Credit Suisse in 2022, it is usually not feasible to find replacement funding quickly enough at a reasonable price. In that case, there is no alternative to reducing cash and/or selling assets[2].
In such a scenario, leverage and capital ratios may actually improve, since the available capital will then support a smaller amount of assets. This is what happened at Credit Suisse during 2022. Although the amount of CET1 capital decreased from CHF 38.5 billion to CHF 35.3 billion (-8.4%), its leverage exposure[3] decreased by 27%. Consequently, the bank’s CET1 leverage ratio improved from 4.3% to 5.4%. Risk-weighted assets (RWA) also decreased, but only by 6%, resulting in a small decrease in the CET1 capital ratio from 14.4% to 14.1%. The changes in CET1 capital, leverage exposure and RWA are depicted in Figure 1.
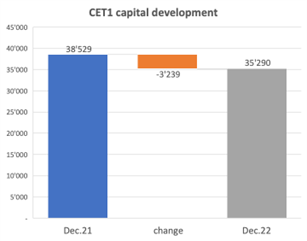
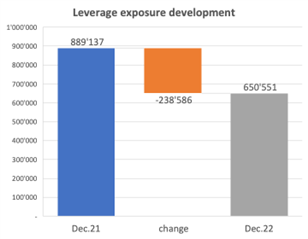
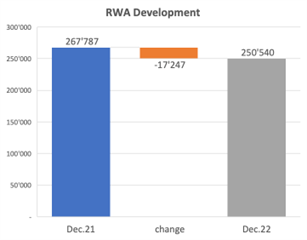
Figure 1: Development in CET1 capital, leverage exposure and risk-weighted assets (RWA) at Credit Suisse between end of December 2021 and end of December 2022 (amounts in CHF million). Source: Credit Suisse Annual Reports 2021 and 2022.
Cash is king
In a situation of large funding withdrawals, it is critical that the bank has a sufficiently large amount of liquid assets. At the end of 2021, Credit Suisse reported CHF 230 billion of liquid assets, consisting of cash held at central banks (CHF 144 billion) and securities[4] that could be pledged to central banks in exchange for cash (CHF 86 billion). At the end of 2022, the amount of liquid assets had decreased to just over CHF 118 billion. Hence, a substantial part of the withdrawal of deposits was met by a reduction in liquid assets. The remainder was met with cash inflows from maturing loans and other assets on the one hand, and replacement with alternative funding on the other.
Lack of sufficient liquid assets was one cause of bank problems during the financial crisis in 2007-08, resulting in extensive liquidity support by central banks. With the aim to prevent this from happening again, the final Basel III rules require banks to satisfy a liquidity coverage ratio (LCR) of at least 100%. This LCR intends to ensure that a bank has sufficient liquidity to sustain significant cash outflows over a 30-day period. The regulatory rules prescribe what cash outflow assumptions need to be made for each type of liability. For example, the FINMA rules for the calculation of the LCR (see FINMA ordinance 2015/2) prescribe that for retail deposits an outflow between 3% and 20% needs to be assumed, with the percentage depending on whether the deposit is insured by a deposit insurance scheme, whether it is on a transactional or non-transactional account, and whether it is a ‘high-value’ deposit. Other outflow assumptions apply to unsecured wholesale funding, secured funding, collateral requirements for derivatives as well as loan and liquidity commitments. The amount of available liquid assets needs to be larger than the cash outflows calculated in this way, net of contractual cash inflows from loans, reverse repos and secured lending within the next 30-day period (all weighted with prescribed percentages). In that case, the LCR exceeds 100% (it is calculated as the amount of liquid assets divided by the difference between assumed cash outflows and contractual cash inflows with prescribed weightings).
At the end of 2022, Credit Suisse had an LCR of 144%, compared to 203% at the end of 2021. Hence, the amount of liquid assets relative to the amount of assumed net cash outflow decreased substantially but remained well above 100%.
Figure 2 compares the balances of the individual liability categories that are subject to cash outflows in the LCR calculation:
- The first column depicts the actual balances at the end of December 2021.
- The second column shows what the remaining balances would be after applying the cash outflow assumptions in the LCR calculation to the December 2021 balances.
- The third column represents the actual balances at the end of December 2022.
This comparison is not fully fair as we compare the actual balances between the start and the end of the full year of 2022, whereas the assumed cash outflows in the LCR calculation relate to a 30-day period. However, Credit Suisse communicated that the largest outflows occurred during the month of October 2022, so the comparison is still instructive.
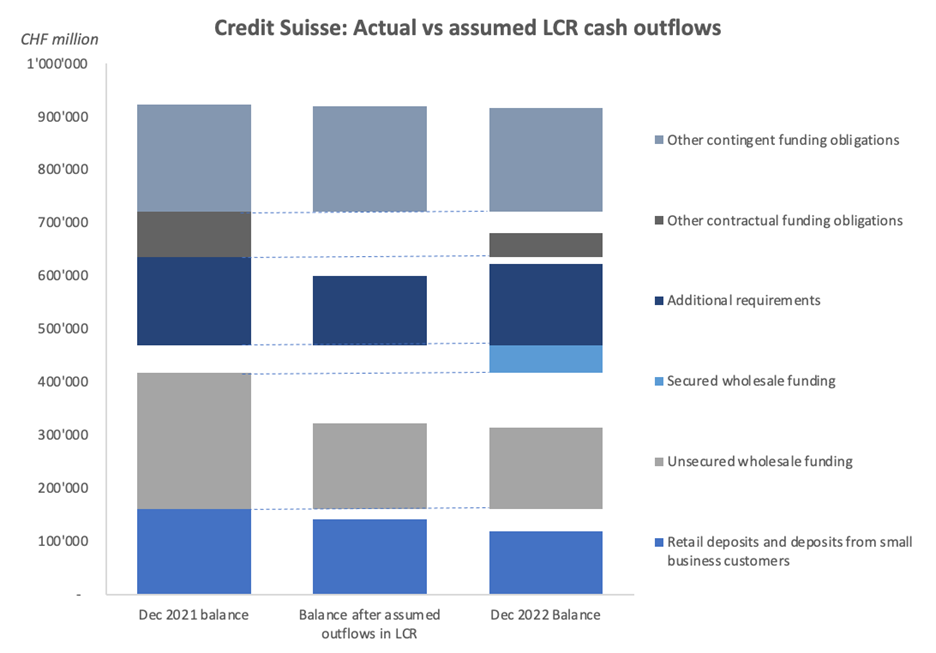
Figure 2: Comparison of balances of liability categories[5] that are subject to cash outflow assumptions in the LCR calculation: Actual balances at the end of December 2021 (first column), balances that result when applying the LCR cash outflow assumptions to the December 2021 balances (second column) and actual balances at the end of December 2022 (third column). Source: Credit Suisse Annual Reports 2021 and 2022.
In aggregate, the actual balances at the end of 2022 are higher than the balances that would result after applying the LCR cash outflow assumptions (CHF 714 billion vs CHF 633 billion, compared to CHF 872 billion at the end of 2021). However, for ‘Retail deposits and deposits from small business customers’ and ‘Unsecured wholesale funding’, the actual outflow was higher than assumed in the LCR calculation. This was more than compensated by an increase in secured wholesale funding and lower outflows in other categories than assumed in the LCR calculation.
In summary, the amount of liquid assets that Credit Suisse had were sufficient to absorb the large withdrawal of funds in October 2022 without the LCR falling below 100%. Trust then seemed to be restored, but only until a new wave of withdrawals took place in March of this year, necessitating a request for liquidity support from the Swiss National Bank (SNB). Unfortunately, the liquidity support by the SNB apparently did not suffice to save Credit Suisse.
What if worse comes to worst?
That both retail depositors and wholesale lenders lost trust in Credit Suisse and withdrew large amounts of money cannot be attributed to its capital and leverage ratios by itself, because they were well above minimum requirements and in line with – if not higher than – those of its peers. Apparently, depositors and lenders lost trust because of doubts that are not visible on a balance sheet, for example:
- Doubts whether the bank would be able to stop losses quickly enough when executing the planned strategy, after a loss of CHF 7.2 billion in 2022.
- Doubts about the management quality of the bank after incurring large losses in isolated incidents (Archegos, Greensill).
- Doubts whether provisions taken for outstanding litigation cases would cover the ultimate fines.
Once such and other material doubts arise, possibly fed by rumors in the market, a bank may end up in a negative spiral of fund withdrawals. In such a situation, it will be unclear to lenders and depositors what the actual financial situation is, even though the last reported figures may have been solid. This unclarity will accelerate further withdrawals. As the developments at Credit Suisse have shown, even a very large pool of liquid assets (for Credit Suisse at the end of 2021 more than twice the amount of net cash outflows assumed in the LCR calculation and almost one-third the size of its balance sheet) will then not be enough. Since such a lack of confidence can escalate within a matter of days, as was the case not only for Credit Suisse but for example also for the Silicon Valley Bank as well as Northern Rock in 2008, there is no time to implement a recovery or resolution plan that the bank may have prepared.
Short of implementing a sovereign-money (‘Vollgeld’) banking system, which has various drawbacks as highlighted for example by the Swiss National Bank (SNB), the only realistic solution to save a bank in such a situation is for the government and/or central bank to step in and publicly commit to providing all necessary liquidity to the bank. It is important to note that this does not have to lead to losses for the government (and therefore the tax payer) as long as the capital situation of the bank in question is adequate. For all we know, that was the case at Credit Suisse.
Footnotes
[1] Only if assets are reduced at a value that differs from the book value (e.g., investments are sold below their book value), then this difference will be reflected in the amount of capital.
[2] In the first quarter of 2023, the Swiss National Bank (SNB) supported Credit Suisse with emergency liquidity funding. As a result, short-term borrowings increased from CHF 12 billion to CHF 118 billion during the quarter. This prevented that Credit Suisse had to further reduce its cash position and/or sell assets, possibly at a loss compared to their book value.
[3] The leverage exposure is equal to the bank’s assets plus a number of regulatory adjustments related mainly to derivative financial instruments and off-balance sheet exposures.
[4] At Credit Suisse, these were mostly US and UK government bonds.
[5] The categories ‘Additional requirements’, ‘Other contractual funding obligations’ and ‘Other contingent funding obligations’ comprise mostly (contingent) off-balance sheet commitments, such as liquidity and loan commitments, guarantees and conditional collateralization requirements.
A comparison between Survival Analysis and Migration Matrix Models
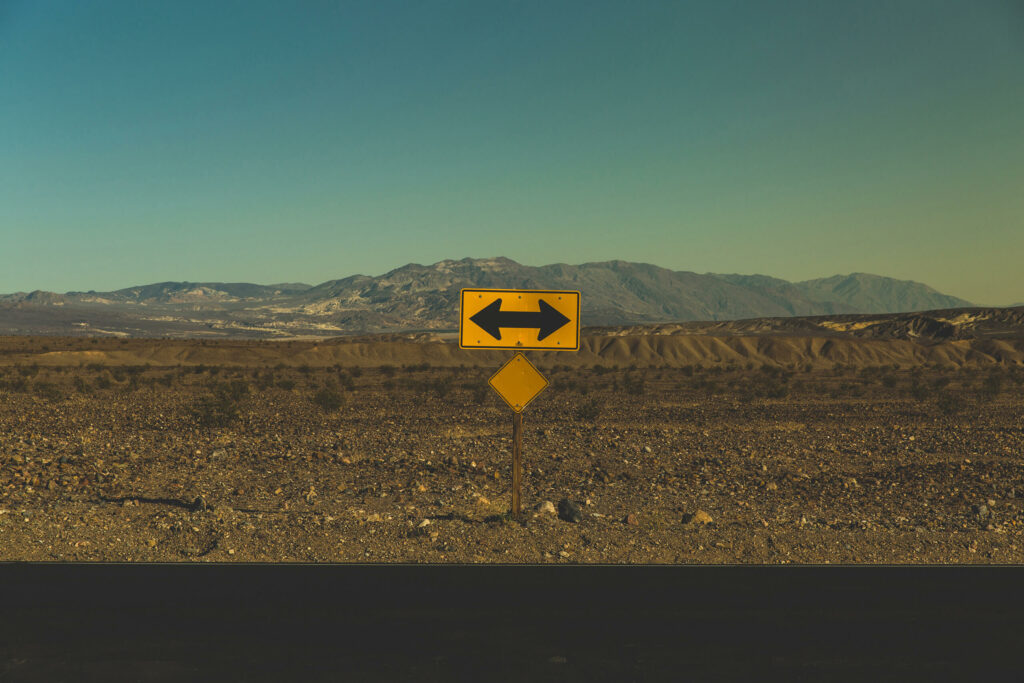
Liquidity planning in SAP Analytics Cloud (SAC) is quite likely SAP’s response to the modernization of Cash Flow Forecasting (CFF) in Corporate Treasury, a key area in today’s treasury trends.
This article provides a thorough comparison of the Survival Analysis and Migration Matrix approach for modeling losses under the internal ratings-based (IRB) approach and IFRS 9. The optimal approach depends on the bank’s situation, and this article highlights that there is no one-size-fits-all solution.
The focus of this read is on the probability of default (PD) component since IFRS 9 differs mainly with regards to the PD component as compared to the IRB Accords (Bank & Eder, 2021), and that most time and effort is given to this component.
Did you implement one approach and are you now wondering what the other approach would have meant for your IFRS 9 modeling? This article compares the two approaches of IFRS 9 modeling and can, thereby, support in answering the question if this approach is still the best approach for your institution.
Background
As of January 2018, banks reporting under IFRS faced the challenge of calculating their expected credit losses in a different way (IASB, 2023). Although IFRS 9 describes principles for calculating expected credit losses, it is not prescribed exactly how to calculate these losses. This in contrast to the IRB requirements, which prescribe how to calculate (un)expected credit losses. As a consequence, banks had to define the best approach to comply with the IFRS 9 requirements. Based on our experience, we look at two prominent approaches, namely: 1) Survival Analysis and 2) the Migration Matrix approach.
Survival Analysis approach
In the credit risk domain, the basic idea behind Survival Analysis is to estimate how long an obligor remains in the portfolio as of the moment of calculation. Survival Analysis models the time to default instead of the event of default and is therefore considered appropriate for modeling lifetime processes. This approach looks at the number of obligors that are at risk at a certain moment in time and the number of obligors that default during a certain period after that moment. Results are used to construct a cumulative distribution function of the time to default. Finally, the marginal probabilities can be obtained, which, after multiplication with the LGD and EAD, yield an estimation of the expected losses over the entire lifetime of a product.
Survival Analysis is particularly useful in addressing censoring in data, which occurs when the event of interest has not occurred yet for some individuals in the data set. Censoring is generally present in the realm of lifetime PD estimations of loans. Especially mortgage loan data is usually heavily censored due to its large maturity. Therefore, defaults may not yet have occurred in the relatively small data span available.
Various extensions of Survival Analysis are proposed in academic literature, enabling the inclusion of individual characteristics (covariates) that may or may not be varying over time, which is relevant if macroeconomic variables have to be included (PIT vs. TTC). For more background on Survival Analysis used for IFRS 9 PD modeling, please refer to Bank & Eder (2021).
We encounter Survival Analysis models frequently at institutions where credit risk portfolios are not (yet) modeled through advanced IRB models. This is due to the fact that IRB models, more specifically the PD models, form a very good basis for the Migration Matrix approach (see next paragraph). In the absence of IRB models, we observe that many institutions chose for the Survival Analysis approach in order to end with one single model, rather than two separate models.
One of the issues when using the Survival Analysis approach is that banks need to develop IRB and IFRS 9 PD models independently, which generally require different data sources and structures, and various methodologies for calculating PD. Consequently, inconsistencies in the estimated PD have been observed due to the utilization of different models and misalignment of IRB and IFRS 9 results. An example of such an inconsistency is an observed increase in estimated creditworthiness according to the IRB PD model, while the IFRS 9 PD decreases. Therefore, banks that chose to independently develop IRB and IFRS 9 PD models have regularly encountered difficulties in explaining these differences to regulators and management.
Migration Matrix approach
The existing infrastructure for estimating the expected loss for capital adequacy purposes, as prescribed by IRB, may be used as source to accommodate the use of IFRS 9 provision modeling. This finding is supported by a monitoring report published by the EBA, which indicates that 59% of the institutions examined have a dependence of their IFRS 9 on their IRB model (EBA, 2021).
IRB outcomes can be used as feeder model for IFRS 9 by utilizing migration matrices. Migration matrices can be established based on the existing rating system, i.e. the IRB rating system used for capital requirements. Each of these ratings can be seen as a state of a Markov Chain, for which the migration probabilities are illustrated in a Migration Matrix. Consequently, along with the probability of default, transformations in creditworthiness may also be observed. A convenient feature of this approach is the ability to extend the horizon on which the PD is estimated by straightforward matrix multiplication. This is especially useful for complying with both IRB and IFRS 9 regulations, where 12-month and multi-period predictions are required, respectively.
Estimating the PD under IRB and IFRS 9 comes with an additional challenge; PDs for capital are required to be Through-The-Cycle (TTC), while IFRS 9 requires them to be Point-In-Time (PIT), depending on macro-economic conditions. A popular model that facilitates the conversion between these two objectives is the Single Factor Vasicek Model (Vasicek, 2002).This model shocks the TTC Migration Matrix with a single risk factor, Z, which is dependent on macroeconomic risk drivers. Consequently, PIT migration matrices are attained, conditional on a future value of Z. Forecasting Z multiple periods ahead enables one to create a series of PIT transition matrices that can be viewed as a time-inhomogeneous Markov Chain. Subsequently, lifetime estimates of the PDs can be calculated by multiplying these matrices.
One of the main issues in applying the migration matrix approach is that you cannot redevelop or recalibrate IRB and IFRS 9 models in parallel. You require to first finish the IRB model before you finish your IFRS 9 model.
Comparison Migration Matrix approach and Survival Analysis
We will now zoom in on the differences between the two approaches mentioned above. This doesn’t imply that these approaches do not share characteristics. Commonalities are, amongst other, that both approaches yield an estimate for future PD, can incorporate macro-economic expectations and are often used during stress test exercises. Table 1 presents a summary of the key features of both the Migration Matrix approach and Survival Analysis, and their interrelationships. The Migration Matrix approach is characterized by its use of a unified PD structure. When estimating different PDs for IRB and IFRS 9, this allows for a simpler explanation on why these differ. Survival Analysis offers the advantage of estimating PD on a per-obligor basis, as opposed to the Migration Matrix approach, which calculates the average PD per rating category. Accordingly, the Migration Matrix approach operates under the assumption that obligors within the same rating category possess similar average PDs over the long term which might not be true.
Whilst the above constitute the primary differences, the two approaches demonstrate many variations across the diverse categories in Table 1. Accordingly, each situation may require a distinct optimal approach implying the absence of a universal best practice.
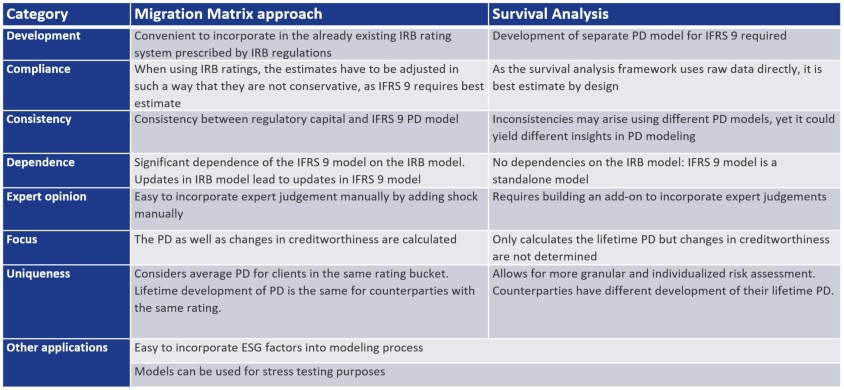
Table 1: Migration Matrix approach vs Survival Analysis
The table of differences indicates that selecting the best approach can be challenging as both approaches have their respective advantages and disadvantages. Therefore, there is no one-size-fits-all solution, and the optimal choice depends on the specific institution’s situation. Fortunately, our experts in this field are available and eager to collaborate with you in identifying and implementing the best possible modeling approach for your institution.
References:
Bank, M., & Eder, B. (2021). A review on the Probability of Default for IFRS 9. Available at SSRN 3981339.
Gae-Carrasco, C. (2015). IFRS 9 Will Significantly Impact Banks’ Provisions and Financial Statements. Moody’s Analytics Risk Perspectives.
IASB (2023). IFRS 9 Financial Instruments. Retrieved from https://www.ifrs.org/issued-standards/list-of-standards/ifrs-9-financial-instruments/
Vasicek, O. (2002). The distribution of loan portfolio value. Risk, 160-162.