Redefining Credit Portfolio Strategies: Balancing Risk & Reward in a Volatile Economy
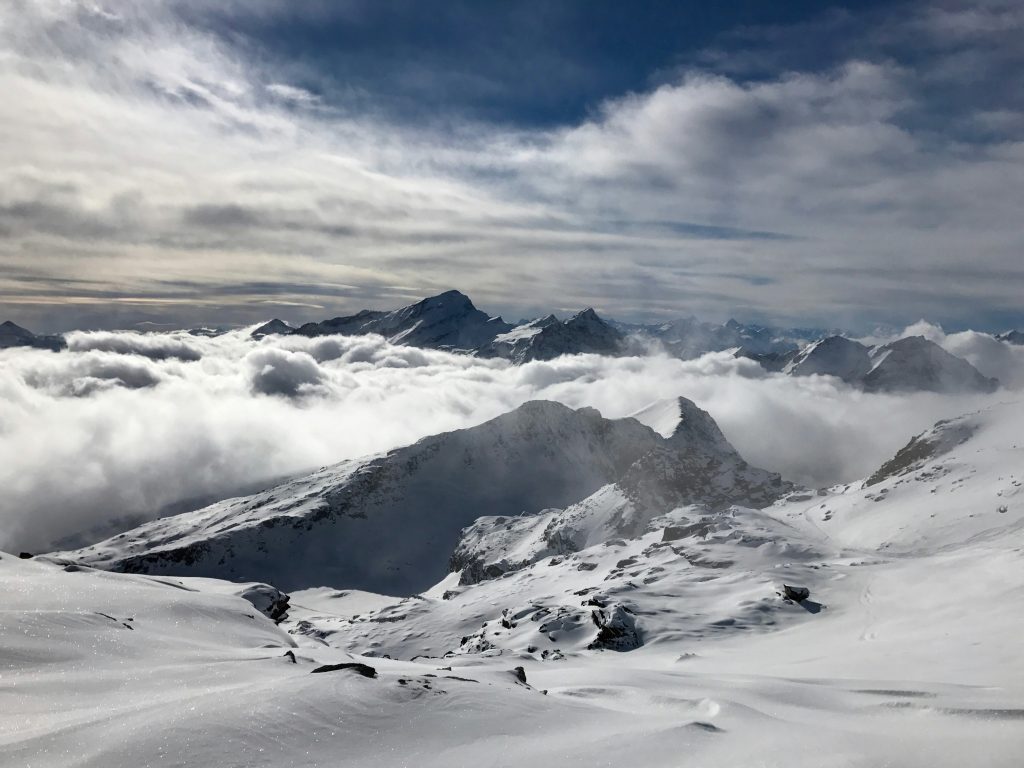
This article delves into a three-step approach to portfolio optimization by harnessing the power of advanced data analytics and state-of-the-art quantitative models and tools.
In today's dynamic economic landscape, optimizing portfolio composition to fortify against challenges such as inflation, slower growth, and geopolitical tensions is ever more paramount. These factors can significantly influence consumer behavior and impact loan performance. Navigating this uncertain environment demands banks adeptly strike a delicate balance between managing credit risk and profitability.
Why does managing your risk reward matter?
Quantitative techniques are an essential tool to effectively optimize your portfolio’s risk reward profile, as this aspect is often based on inefficient approaches.
Existing models and procedures across the credit lifecycle, especially those relating to loan origination and account management, may not be optimized to accommodate current macro-economic challenges.
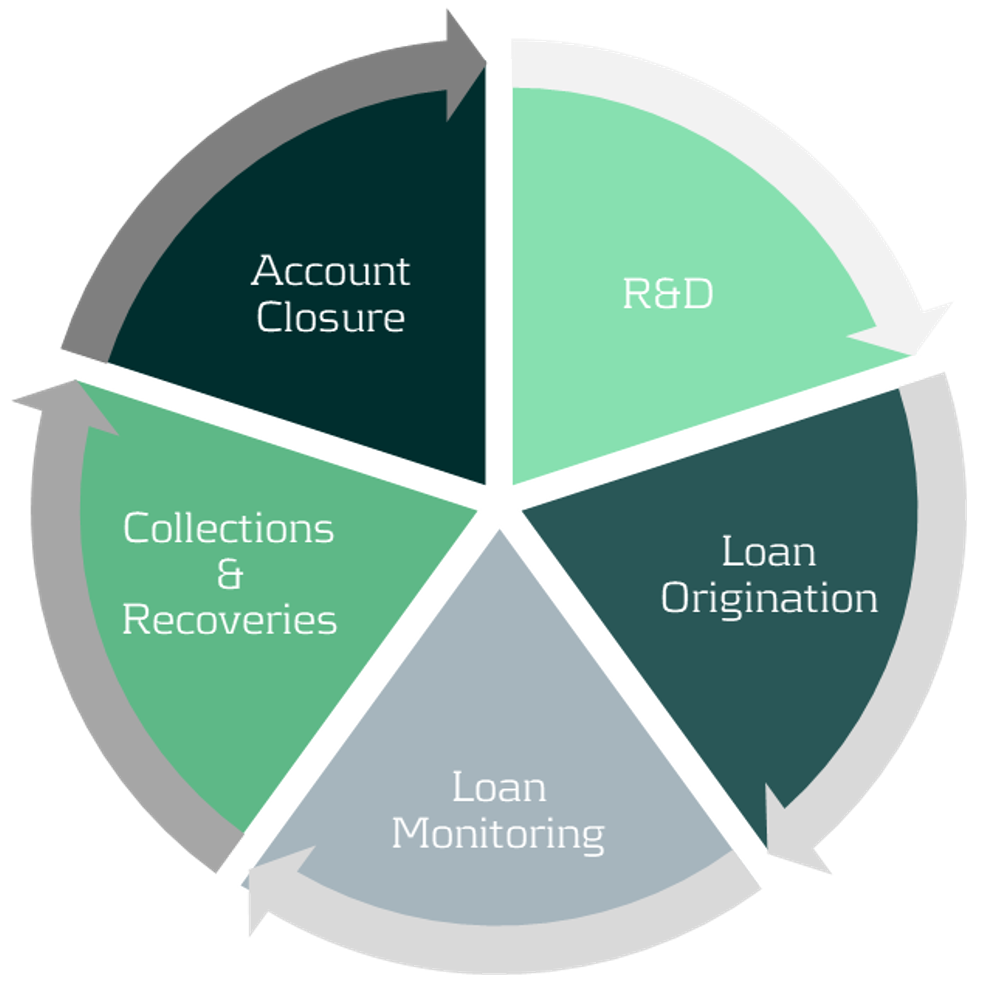
Figure 1: Credit lifecycle.
Current challenges facing banks
Some of the key challenges banks face when balancing credit risk and profitability include:
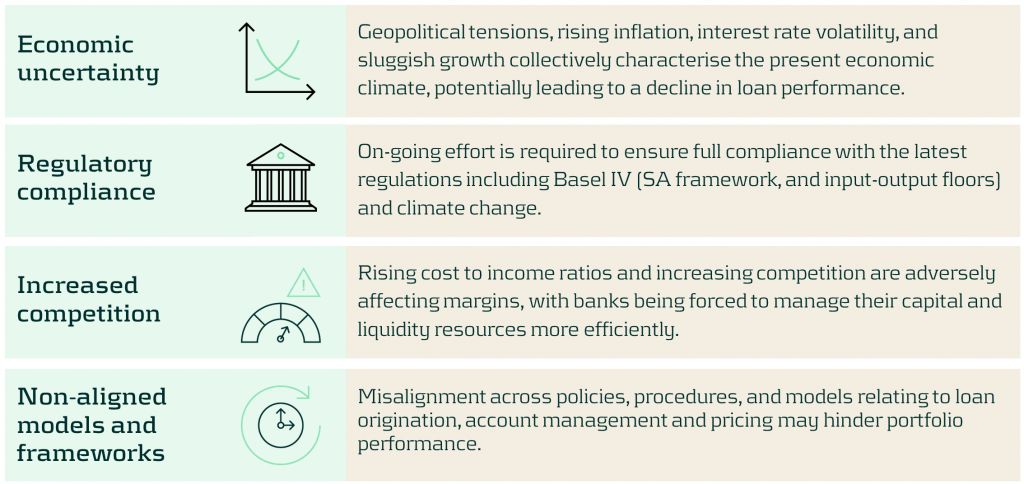
Our approach to optimizing your risk reward profile
Our optimization approach consists of a holistic three step diagnosis of your current practices, to support your strategy and encourage alignment across business units and processes.
The initial step of the process involves understanding your current portfolio(s) by using a variety of segmentation methodologies and metrics. The second step implements the necessary changes once your primary target populations have been identified. This may include reassessing your models and strategies across the loan origination and account management processes. Finally, a new state-of-the-art Early Warning System (EWS) can be deployed to identify emerging risks and take pro-active action where necessary.
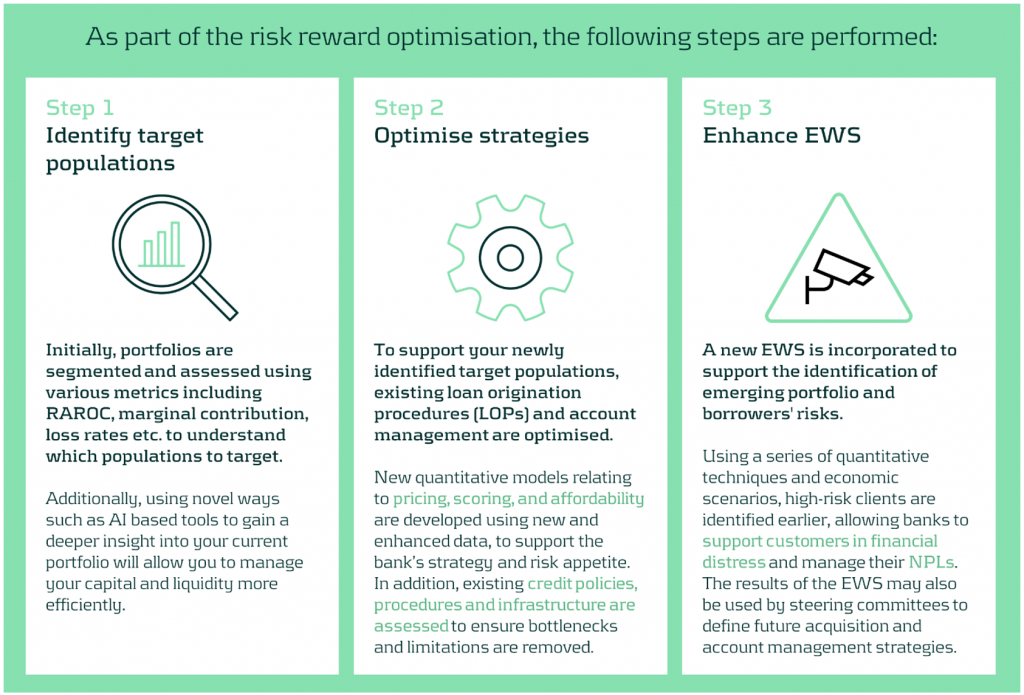
A closer look at redefining your target populations
With the proliferation of advanced data analytics, banks are now better positioned to identify profitable, low-risk segments. Machine Learning (ML) methodologies such as k-means clustering, neural networks, and Natural Language Processing (NLP) enable effective customer grouping, behavior forecasting, and market sentiment analysis.
Risk-based pricing remains critical for acquisition strategies, assessing segment sensitivity to different pricing strategies, to maximize revenue and reduce credit losses.
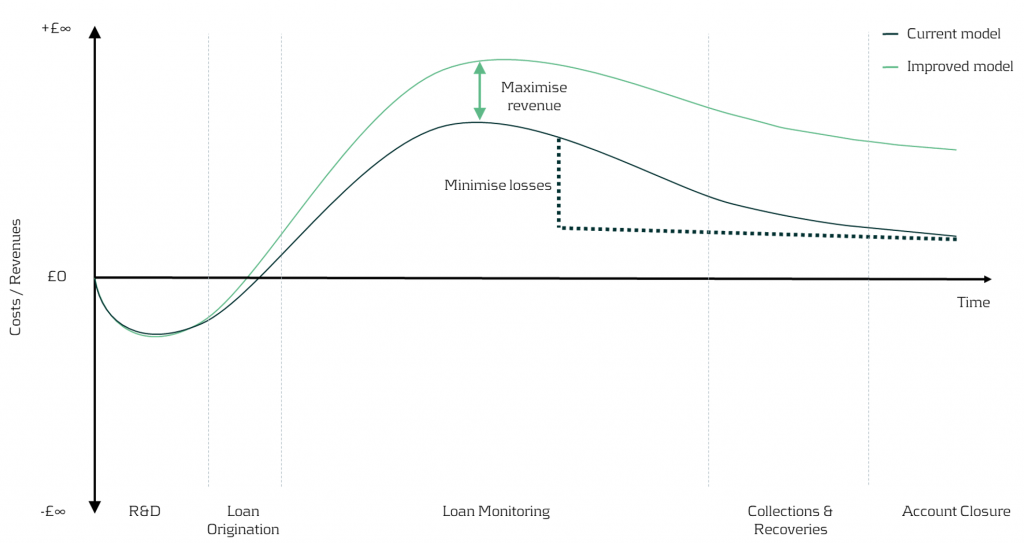
Figure 2: In the illustration above, we can visually see the impact on earnings throughout the credit lifecycle driven by redefining the target populations and application of different pricing strategies.
In our simplified example, based on the RAROC metric applied to an unsecured loans portfolio, we take a 2-step approach:
1- Identify target populations by comparing RAROC across different combinations of credit scores and debt-to-income (DTI) ratios. This helps identify the most capital efficient segments to target.
2- Assess the sensitivity of RAROC to different pricing strategies to find the optimal price points to maximize profit over a select period - in this scenario we use a 5-year time horizon.
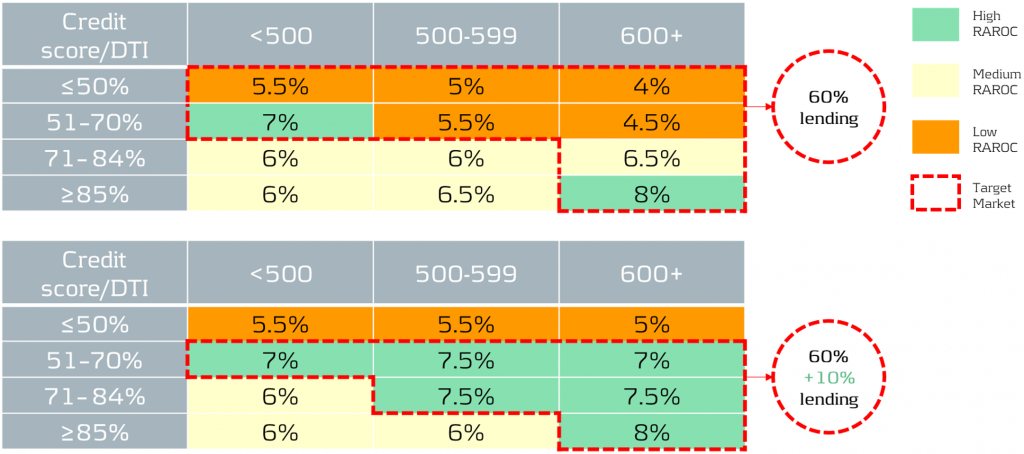
Figure 3: The top table showcases the current portfolio mix and performance, while the bottom table illustrates the effects of adjusting the pricing and acquisition strategy. By redefining the target populations and changing the pricing strategy, it is possible to reallocate capital to the most profitable segments whilst maintaining within credit risk appetite. For example, 60% of current lending is towards a mix of low to high RAROC segments, but under the new proposed strategy, 70% of total capital is allocated to the highest RAROC segments.
Uncovering risks and seizing opportunities
The current state of Early Warning Systems
Many organizations rely on regulatory models and standard risk triggers (e.g., no. of customers 30 day past due, NPL ratio etc.) to set their EWS thresholds. Whilst this may be a good starting point, traditional models and tools often miss timely deteriorations and valuable opportunities, as they typically use limited and/or outdated data features.
Target state of Early Warning Systems
Leveraging timely and relevant data, combined with next-generation AI and machine learning techniques, enables early identification of customer deterioration, resulting in prompt intervention and significantly lower impairment costs and NPL ratios.
Furthermore, an effective EWS framework empowers your organization to spot new growth areas, capitalize on cross-selling opportunities, and enhance existing strategies, driving significant benefits to your P&L.
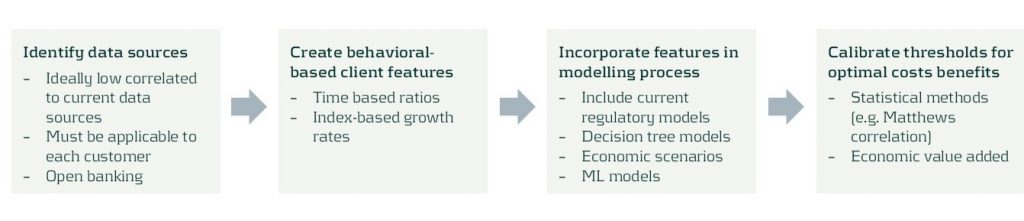
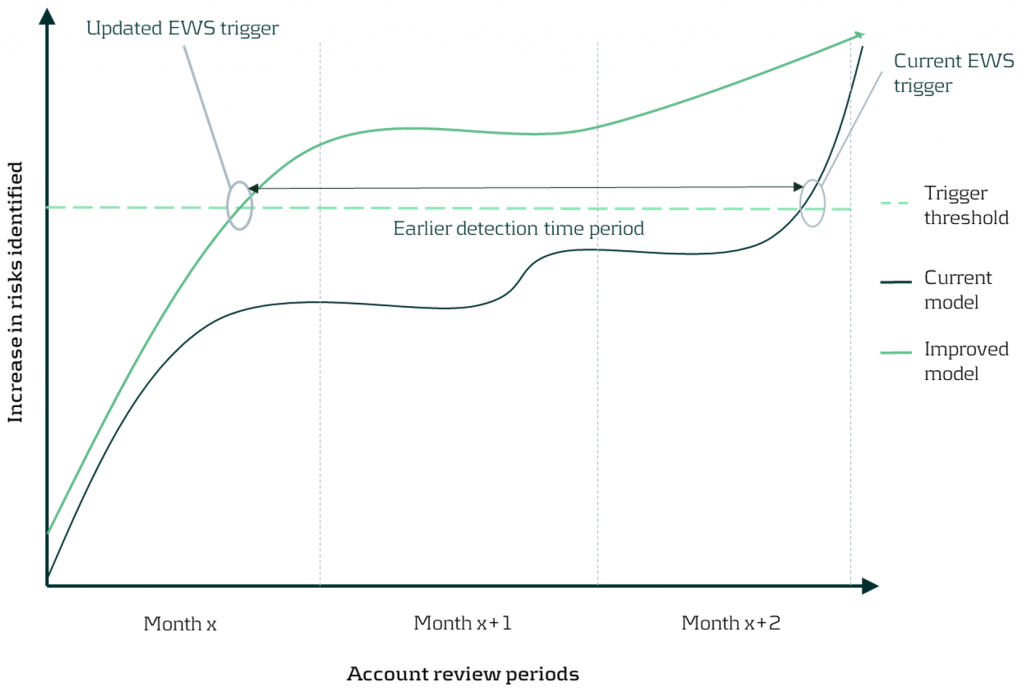
Figure 4: By updating the early warning triggers using new timely data and advanced techniques, detection of customer deterioration can be greatly improved enabling firms to proactively support clients and enhance the firm’s financial position.
Discover the benefits of optimizing your portfolios
Discover the benefits in optimizing your portfolios’ risk-reward profile using our comprehensive approach as we turn today’s challenges into tomorrow’s advantages. Such benefits include:
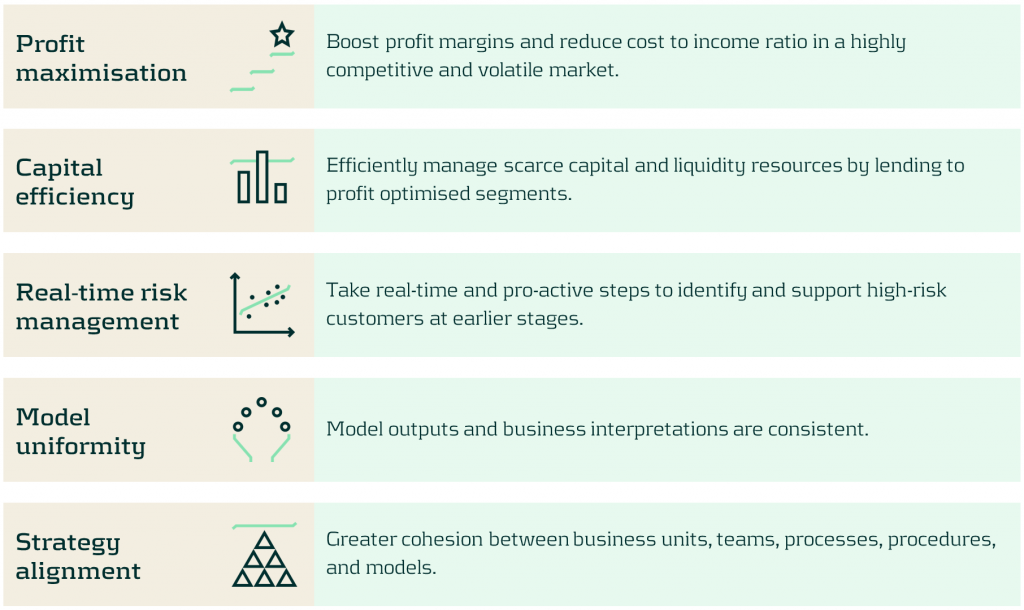
Conclusion
In today's rapidly evolving market, the need for sophisticated credit risk portfolio management is ever more critical. With our comprehensive approach, banks are empowered to not merely weather economic uncertainties, but to thrive within them by striking the optimal risk-reward balance. Through leveraging advanced data analytics and deploying quantitative tools and models, we help institutions strategically position themselves for sustainable growth, and comply with increasing regulatory demands especially with the advent of Basel IV. Contact us to turn today’s challenges into tomorrow’s opportunities.
For more information on this topic, contact Martijn de Groot (Partner) or Paolo Vareschi (Director).
Optimizing Liquidity Management with SAP: Cash Flow Analyzer and Short-Term Cash Positioning
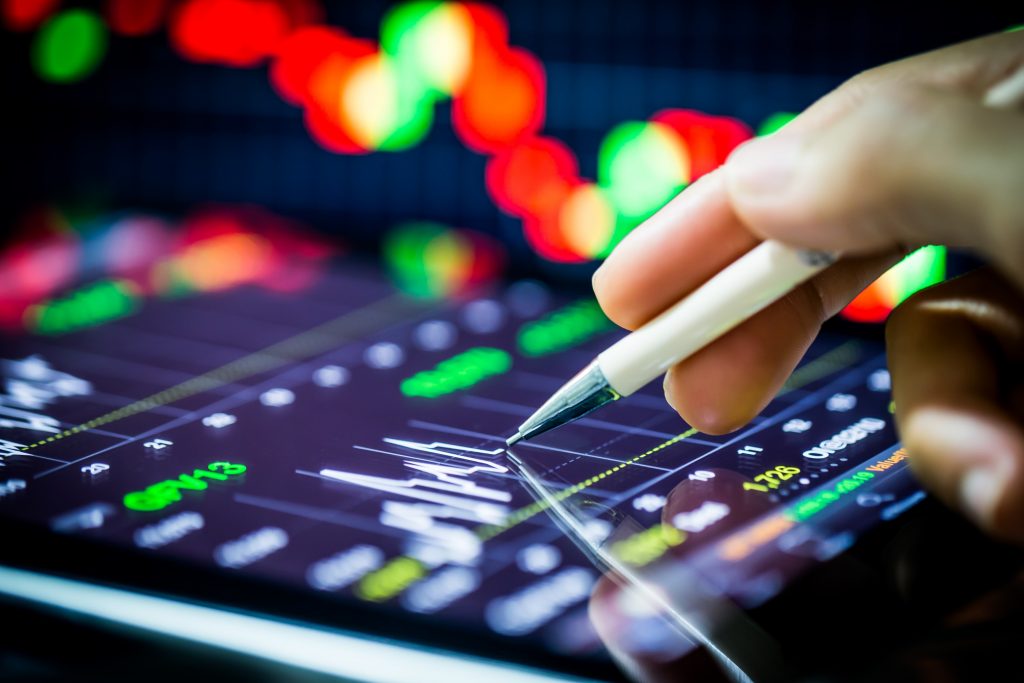
Mastering liquidity management is key to business success. With SAP’s Cash Flow Analyzer and Short-Term Cash Positioning apps, companies can streamline financial planning and make smarter, data-driven decisions.
Effective liquidity management is essential for businesses of all sizes, yet achieving it is often challenging. Many organizations face difficulties due to fragmented data, inconsistent reporting, and the complexity of managing cash flows across different time horizons. These challenges are amplified in companies with complex cash flow structures, where tailored configurations and precise tracking are crucial for accurate planning and decision-making.
SAP offers two tools to support liquidity management: the Cash Flow Analyzer and the Short-Term Cash Positioning apps. This article examines how these tools address different aspects of liquidity management, helping businesses navigate their financial challenges and make informed decisions.
Exploring the Cash Flow Analyzer
The Cash Flow Analyzer app offers a versatile approach to liquidity management. It enables companies to screen and analyze cash flows with filters, drill-down options, and flexible time horizons—whether for medium or long-term planning.
A key strength of this app lies in its integration across SAP modules like FI (Finance), MM (Materials Management), SD (Sales and Distribution), and TRM (Treasury and Risk Management). This ensures real-time updates through SAP’s One Exposure functionality, giving companies a single source of truth for cash flow data.
SAP continues to enhance this app with updates, such as the Liquidity Calculation: G/L Classification, available in the public cloud. This feature simplifies configurations by replacing the older Define Default Liquidity Items for G/L Accounts while also offering a migration option for existing setups. With this new feature, old document chain stopping functionality in the predecessor version will be replaced by G/L account classification. Also, you will be able to see the prioritization logic behind the liquidity item assignment when there are multiple derivations for the same G/L account. Although currently available only for public cloud users, SAP is expected to bring these enhancements to private cloud and on-premises systems in the future.
Query-Based Liquidity Derivations
For companies with complex cash flow structures, query-based derivations allow for tailored liquidity item configurations in addition to defining default liquidity items for G/L accounts. This feature lets you define rules for assigning liquidity items based on unique needs. You can set different conditions in the query to pull up the correct liquidity item from the different sources which are captured by different origins of queries.
These queries seamlessly integrate data from various SAP modules, such as Finance, Treasury, and Sales, ensuring comprehensive coverage of liquidity-related processes. Additionally, hierarchical rules can be applied to manage overlapping conditions, providing clear prioritization for liquidity item assignments. By accommodating both actual and forecasted cash flows, query-based derivations support precise tracking and planning across different time horizons. Furthermore, the approach enhances transparency by offering visibility into the logic behind liquidity item assignments, enabling finance teams to validate and adapt their configurations effectively.
Cash Flow Analyzer in Action
The app’s flexibility doesn’t stop at data collection. It allows users to create custom layouts and views based on filters like liquidity items, company codes, bank accounts, or cash pools. By defining hierarchies, businesses can drill down into the details of their liquidity positions, analyze trends, and make data-driven decisions. Please find a sample screenshot from a demo system:
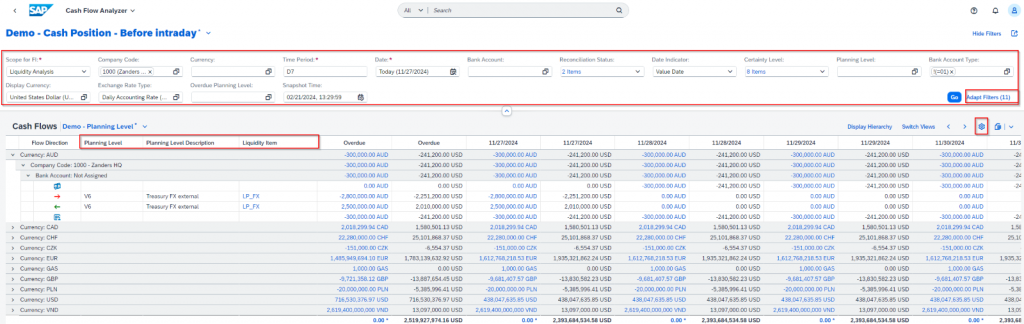
As shown in the screenshot above, the upper red rectangle allows you to set or remove a variety of filters as needed. The red rectangle below is another element that can be added or removed from your layout. If you want to see more details about cash flows, click the gear button and add or remove more.
Why Use Short-Term Cash Positioning?
With the Cash Flow Analyzer’s extensive capabilities, you may wonder why SAP offers a separate app for short-term cash management. The answer lies in its focus.
Short-Term Cash Positioning (STCP) is an SAP Fiori app designed to give treasury teams a focused view of their immediate cash position. Unlike broader tools like the Cash Flow Analyzer, STCP hones in on short-term liquidity, offering a straightforward way to manage cash inflows and outflows over a defined horizon—usually within a few days or weeks.
At its core, STCP organizes and presents cash flow data based on planning levels and cash position profiles. These profiles help group bank accounts or cash pools, providing treasury teams with a clear snapshot of their short-term liquidity. With intuitive visuals and fast data processing, the app simplifies decision-making in fast-paced environments. While the Cash Flow Analyzer provides a broad, flexible view, the Short-Term Cash Positioning (STCP) app is purpose-built for immediate liquidity analysis.
Here are some reasons to consider using STCP:
1- Speed and Simplicity: Designed for quick, straightforward analysis, the STCP app helps companies handle large volumes of cash flow data without getting bogged down by complexity.
2- Focus on Planning Levels: This app emphasizes planning levels to categorize cash flows for short-term financial planning.
3- Customizable Cash Position Profiles: Users can define hierarchies based on bank account master data, existing bank account hierarchies, or cash pools. These profiles allow for real-time monitoring of cash flows within defined groupings.
4- Clear Visualization: By focusing on shorter time horizons, STCP provides an actionable snapshot of a company’s cash position. This can be helpful for businesses with frequent cash inflows and outflows.
5- Efficient Decision-Making: The STCP app offers a focused approach, helping decision-makers access relevant information to manage liquidity effectively while avoiding unnecessary complexities.
For businesses balancing long-term planning with immediate liquidity needs, the Short-Term Cash Positioning app can complement the Cash Flow Analyzer. Together, they provide a comprehensive set of tools that address various aspects of liquidity management. However, businesses should carefully assess their specific requirements to determine the most effective way to use these tools, ensuring they align with their operational priorities and evolving needs.
A Holistic Approach to Liquidity Management
The Cash Flow Analyzer provides broader liquidity insights, while the Short-Term Cash Positioning app focuses on immediate needs. Together, these tools can help businesses address liquidity challenges across different time horizons, supporting better visibility and informed decision-making in both the short and long term. Since these two tools have distinct capabilities, a thorough evaluation of these differences should be conducted to ensure the chosen solution aligns with the organization’s specific needs and priorities.
If you would like to learn more about the Cash Flow Analyzer and Short-Term Cash Positioning apps - or explore how to customize these tools for your business needs - please reach out to our Senior Consultant Merve Korukcu.
Strategic Insights on S/4HANA Treasury Innovations and Migration Options
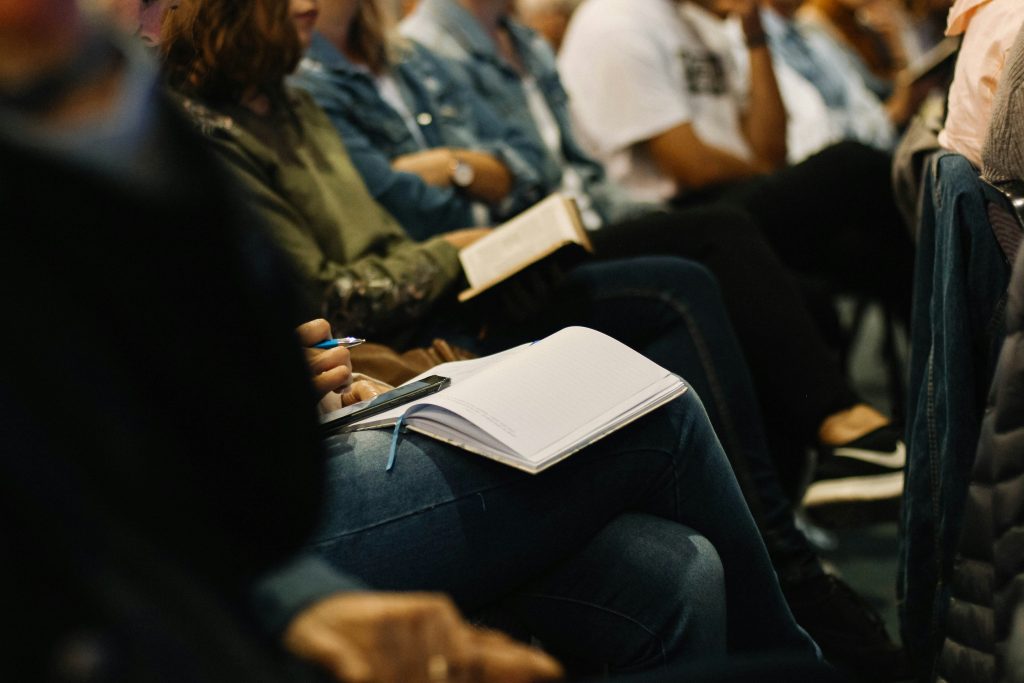
On Thursday, November 14th, SAP Netherlands and Zanders hosted a roundtable focused on upgrading to S/4HANA. Nineteen participants representing nine companies, actively engaged in the discussions. This article will focus on the specifics of the discussions.
Exploring S/4HANA Functionalities
The roundtable session started off with the presentation of SAP on some of the new S/4HANA functionalities. New functionalities in the areas of Cash Management, Financial Risk Management, Working Capital Management and Payments were presented and discussed. In the area of Cash Management, the main enhancements can be found in the management of bank relationships, managing cash operations, cash positioning, and liquidity forecasting and planning. These enhancements provide greater visibility into bank accounts and cash positions, a more controlled liquidity planning process across the organization, increased automation, and better execution of working capital strategies. In Financial Risk Management, the discussion highlighted S/4HANA’s support for smart trading processes, built-in market data integration, and more advanced on-the-fly analysis capabilities. All providing companies with a more touchless, automated and straight through process of their risk management process. The session also covered Working Capital enhancements, including a presentation on the Taulia solution offered by SAP, which provides insights into supporting Payables and Receivables Financing. Finally, the session explored innovations in the Payments area, such as payment verification against sanction lists, format mapping tools, the SAP Digital Payments Add-on, and automated corporate-to-bank cloud connectivity.
Migration Strategies: Getting to S/4HANA
While the potential of S/4HANA was impressive, the focus shifted to migration strategies. Zanders presented various options for transitioning from an ECC setup to an S/4HANA environment, sparking a lively discussion. Four use cases were defined, reflecting the diverse architectural setups in companies. These setups include:
- An integrated architecture, where the SAP Treasury solution is embedded within the SAP ERP system
- A treasury sidecar approach, where the SAP Treasury solution operates on a separate box and needs to integrate with the SAP ERP system box
- Treasury & Cash & Banking side car
- Leveraging Treasury on an S/4HANA Central Finance box
The discussion also covered two key migration strategies: the brownfield approach and the greenfield approach. In a brownfield approach, the existing system setup is technically upgraded to the new version, allowing companies to implement S/4HANA enhancements incrementally. In contrast, a greenfield approach involves building a new system from scratch. While companies can reuse elements of their ECC-based SAP Treasury implementation, starting fresh allows them to fully leverage S/4HANA’s standard functionalities without legacy constraints. However, the greenfield approach requires careful planning for data migration and testing, as legacy data must be transferred to the new environment.
Decoupling Treasury: The Sidecar Approach
The greenfield approach also raised the question of whether treasury activities should migrate to S/4HANA first using a sidecar system. This would involve decoupling treasury from the integrated ECC setup and transitioning to a dedicated S/4HANA sidecar system. This approach allows treasury to access new S/4HANA functionalities ahead of the rest of the organization, which can be beneficial if immediate enhancements are required. However, this setup comes with challenges, including increased system maintenance complexity, additional costs, and the need to establish new interfaces.
However, this setup comes with challenges, including increased system maintenance complexity, additional costs, and the need to establish new interfaces. Companies need to weigh the benefits of an early treasury migration against these potential drawbacks as part of their overall S/4HANA strategy. With this consideration in mind, participants reflected on the broader lessons from companies already using S/4HANA.
Lessons from Early Adopters
Companies that have already migrated to S/4HANA emphasized two critical planning areas: testing and training. Extensive testing—ideally automated—should be prioritized, especially for diverse payment processes. Similarly, training is essential to ensure effective change management, reducing potential issues after migration.
These insights highlight the importance of preparation in achieving a smooth migration. As organizations transition to S/4HANA, another important consideration is the potential impact on the roles and responsibilities within treasury teams.
Impact on Treasury Roles
Participants discussed whether S/4HANA would alter roles and responsibilities within treasury departments. The consensus was that significant changes are unlikely, particularly in a brownfield approach. Even in a greenfield approach, roles and responsibilities are expected to remain largely unchanged.
Conclusion
The roundtable highlighted the significant value S/4HANA brings to treasury operations, particularly through enhanced functionalities in Cash Management, Financial Risk Management, Working Capital Management, and Payments.
Participants discussed the pros and cons of brownfield and greenfield migration strategies, with insights into the sidecar approach for treasury as a potential transitional strategy. Early adopters emphasized the critical importance of thorough testing and training for a successful migration, while noting that treasury roles and responsibilities are unlikely to see major changes
If you would like to hear more about the details of the discussion, please reach out to Laura Koekkoek, Partner at Zanders, l.koekkoek@zandersgroup.com
The Benefits of Exposure Attribution in Counterparty Credit Risk
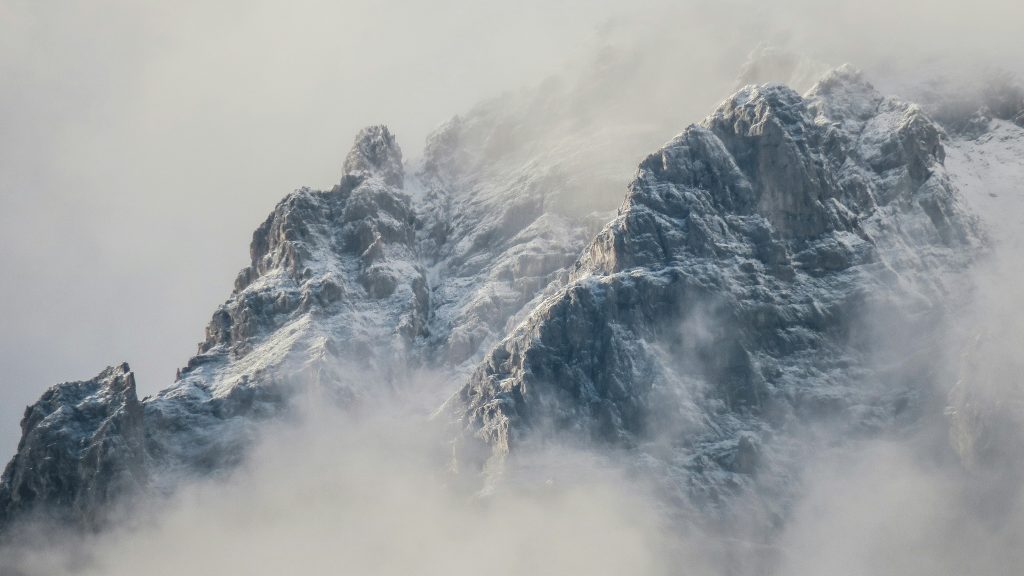
In an increasingly complex regulatory landscape, effective management of counterparty credit risk is crucial for maintaining financial stability and regulatory compliance.
Accurately attributing changes in counterparty credit exposures is essential for understanding risk profiles and making informed decisions. However, traditional approaches for exposure attribution often pose significant challenges, including labor-intensive manual processes, calculation uncertainties, and incomplete analyses.
In this article, we discuss the issues with existing exposure attribution techniques and explore Zanders’ automated approach, which reduces workloads and enhances the accuracy and comprehensiveness of the attribution.
Our approach to attributing changes in counterparty credit exposures
The attribution of daily exposure changes in counterparty credit risk often presents challenges that strain the resources of credit risk managers and quantitative analysts. To tackle this issue, Zanders has developed an attribution methodology that efficiently automates the attribution process, improving the efficiency, reactivity and coverage of exposure attribution.
Challenges in Exposure Attribution
Credit risk managers monitor the evolution of exposures over time to manage counterparty credit risk exposures against the bank’s risk appetite and limits. This frequently requires rapid analysis to attribute the changes to exposures, which presents several challenges:
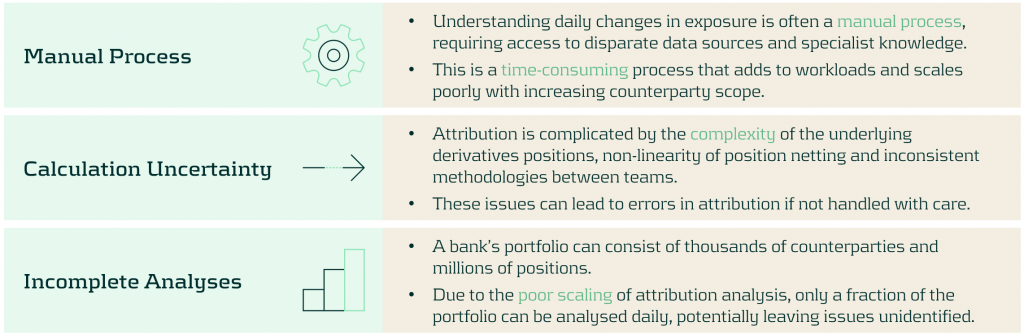
Zanders’ approach: an automated approach to exposure attribution
Our methodology resolves these problems with an analytics layer that interfaces with the risk engine to accelerate and automate the daily exposure attribution process. The results can also be accessed and explored via an interactive web portal, providing risk managers and senior management with the tools they need to rapidly analyze and understand their risk.
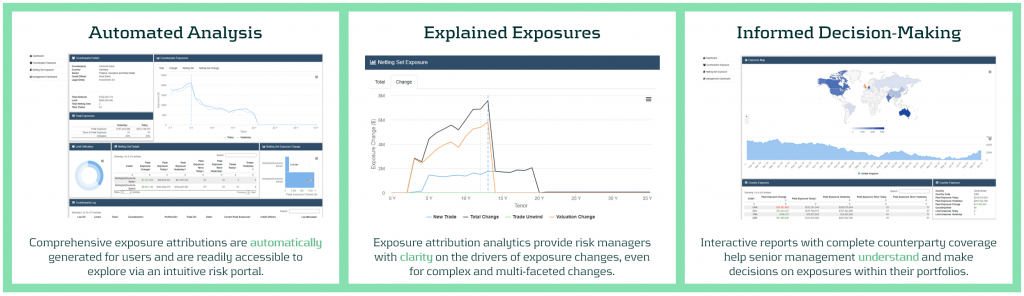
Key features and benefits of our approach
Zanders’ approach provides multiple improvements to the exposure attribution process. This reduces the workloads of key risk teams and increases risk coverage without additional overheads. Below, we describe the benefits of each of the main features of our approach.
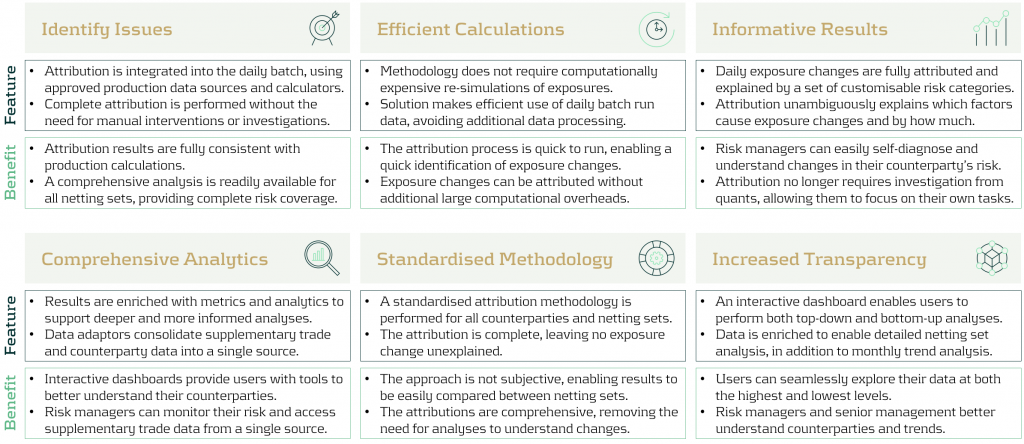
Zanders Recommends
An automated attribution of exposures empowers banks teams to better understand and handle their counterparty credit risk. To make the best use of automated attribution techniques, Zanders recommends that banks:
- Increase risk scope: The increased efficiency of attribution should be used to provide a more comprehensive and granular coverage of the exposures of counterparties, sectors and regions.
- Reduce quant utilization: Risk managers should use automated dashboards and analytics to perform their own exposure investigations, reducing the workload of quantitative risk teams.
- Augment decision making: Risk managers should utilize dashboards and analytics to ensure they make more timely and informed decisions.
- Proactive monitoring: Automated reports and monitoring should be reviewed regularly to ensure risks are tackled in a proactive manner.
- Increase information transfer: Dashboards should be made available across teams to ensure that information is shared in a transparent, consistent and more timely manner.
Conclusion
The effective management of counterparty credit risk is a critical task for banks and financial institutions. However, the traditional approach of manual exposure attribution often results in inefficient processes, calculation uncertainties, and incomplete analyses. Zanders' innovative methodology for automating exposure attribution offers a comprehensive solution to these challenges and provides banks with a robust framework to navigate the complexities of exposure attribution. The approach is highly effective at improving the speed, coverage, and accuracy of exposure attribution, supporting risk managers and senior management to make informed and timely decisions.
For more information about how Zanders can support you with exposure attribution, please contact Dilbagh Kalsi (Partner) or Mark Baber (Senior Manager).
Converging on resilience: Integrating CCR, XVA, and real-time risk management
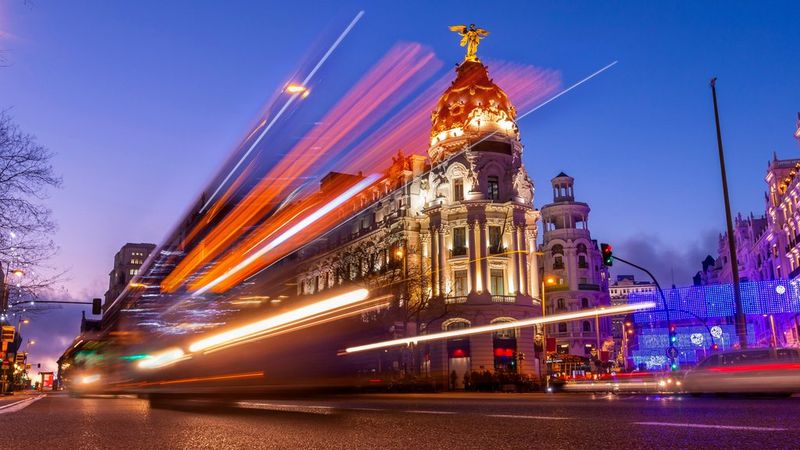
In a world where the Fundamental Review of the Trading Book (FRTB) commands much attention, it’s easy for counterparty credit risk (CCR) to slip under the radar.
However, CCR remains an essential element in banking risk management, particularly as it converges with valuation adjustments. These changes reflect growing regulatory expectations, which were further amplified by recent cases such as Archegos. Furthermore, regulatory focus seems to be shifting, particularly in the U.S., away from the Internal Model Method (IMM) and toward standardised approaches. This article provides strategic insights for senior executives navigating the evolving CCR framework and its regulatory landscape.
Evolving trends in CCR and XVA
Counterparty credit risk (CCR) has evolved significantly, with banks now adopting a closely integrated approach with valuation adjustments (XVA) — particularly Credit Valuation Adjustment (CVA), Funding Valuation Adjustment (FVA), and Capital Valuation Adjustment (KVA) — to fully account for risk and costs in trade pricing. This trend towards blending XVA into CCR has been driven by the desire for more accurate pricing and capital decisions that reflect the true risk profile of the underlying instruments/ positions.
In addition, recent years have seen a marked increase in the use of collateral and initial margin as mitigants for CCR. While this approach is essential for managing credit exposures, it simultaneously shifts a portion of the risk profile into contingent market and liquidity risks, which, in turn, introduces requirements for real-time monitoring and enhanced data capabilities to capture both the credit and liquidity dimensions of CCR. Ultimately, this introduces additional risks and modelling challenges with respect to wrong way risk and clearing counterparty risk.
As banks continue to invest in advanced XVA models and supporting technologies, senior executives must ensure that systems are equipped to adapt to these new risk characteristics, as well as to meet growing regulatory scrutiny around collateral management and liquidity resilience.
The Internal Model Method (IMM) vs. SA-CCR
In terms of calculating CCR, approaches based on IMM and SA-CCR provide divergent paths. On one hand, IMM allows banks to tailor models to specific risks, potentially leading to capital efficiencies. SA-CCR, on the other hand, offers a standardised approach that’s straightforward yet conservative. Regulatory trends indicate a shift toward SA-CCR, especially in the U.S., where reliance on IMM is diminishing.
As banks shift towards SA-CCR for Regulatory capital and IMM is used increasingly for internal purposes, senior leaders might need to re-evaluate whether separate calibrations for CVA and IMM are warranted or if CVA data can inform IMM processes as well.
Regulatory focus on CCR: Real-time monitoring, stress testing, and resilience
Real-time monitoring and stress testing are taking centre stage following increased regulatory focus on resilience. Evolving guidelines, such as those from the Bank for International Settlements (BIS), emphasise a need for efficiency and convergence between trading and risk management systems. This means that banks must incorporate real-time risk data and dynamic monitoring to proactively manage CCR exposures and respond to changes in a timely manner.
CVA hedging and regulatory treatment under IMM
CVA hedging aims to mitigate counterparty credit spread volatility, which affects portfolio credit risk. However, current regulations limit offsetting CVA hedges against CCR exposures under IMM. This regulatory separation of capital for CVA and CCR leads to some inefficiencies, as institutions can’t fully leverage hedges to reduce overall exposure.
Ongoing BIS discussions suggest potential reforms for recognising CVA hedges within CCR frameworks, offering a chance for more dynamic risk management. Additionally, banks are exploring CCR capital management through LGD reductions using third-party financial guarantees, potentially allowing for more efficient capital use. For executives, tracking these regulatory developments could reveal opportunities for more comprehensive and capital-efficient approaches to CCR.
Leveraging advanced analytics and data integration for CCR
Emerging technologies in data analytics, artificial intelligence (AI), and scenario analysis are revolutionising CCR. Real-time data analytics provide insights into counterparty exposures but typically come at significant computational costs: high-performance computing can help mitigate this, and, if coupled with AI, enable predictive modelling and early warning systems. For senior leaders, integrating data from risk, finance, and treasury can optimise CCR insights and streamline decision-making, making risk management more responsive and aligned with compliance.
By leveraging advanced analytics, banks can respond proactively to potential CCR threats, particularly in scenarios where early intervention is critical. These technologies equip executives with the tools to not only mitigate CCR but also enhance overall risk and capital management strategies.
Strategic considerations for senior executives: Capital efficiency and resilience
Balancing capital efficiency with resilience requires careful alignment of CCR and XVA frameworks with governance and strategy. To meet both regulatory requirements and competitive pressures, executives should foster collaboration across risk, finance, and treasury functions. This alignment will enhance capital allocation, pricing strategies, and overall governance structures.
For banks facing capital constraints, third-party optimisation can be a viable strategy to manage the demands of SA-CCR. Executives should also consider refining data integration and analytics capabilities to support efficient, resilient risk management that is adaptable to regulatory shifts.
Conclusion
As counterparty credit risk re-emerges as a focal point for financial institutions, its integration with XVA, and the shifting emphasis from IMM to SA-CCR, underscore the need for proactive CCR management. For senior risk executives, adapting to this complex landscape requires striking a balance between resilience and efficiency. Embracing real-time monitoring, advanced analytics, and strategic cross-functional collaboration is crucial to building CCR frameworks that withstand regulatory scrutiny and position banks competitively.
In a financial landscape that is increasingly interconnected and volatile, an agile and resilient approach to CCR will serve as a foundation for long-term stability. At Zanders, we have significant experience implementing advanced analytics for CCR. By investing in robust CCR frameworks and staying attuned to evolving regulatory expectations, senior executives can prepare their institutions for the future of CCR and beyond thereby avoiding being left behind.
Confirmed Methodology for Credit Risk in EBA 2025 Stress Test
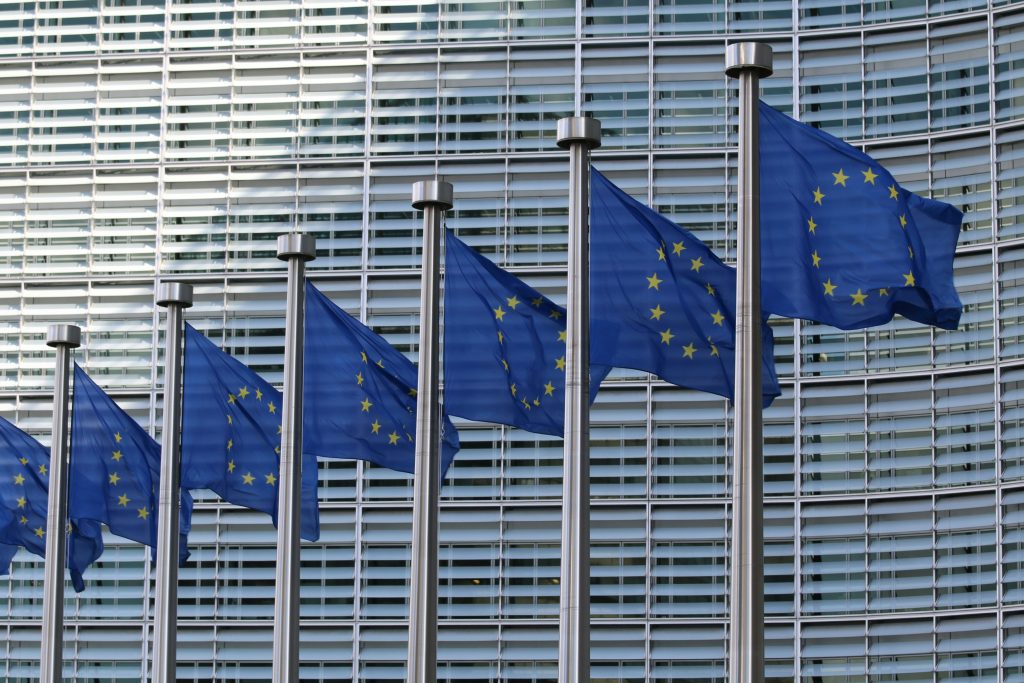
On November 12 2024, the confirmed methodology for the EBA 2025 stress testing exercise was published on the EBA website. This is the final version of the draft for initial consultation that was published earlier.
The timelines for the entire exercise have been extended to accommodate the changes in scope: | |
Launch of exercise (macro scenarios) | Second half of January 2025 |
First submission of results to the EBA | End of April 2025 |
Second submission to the EBA | Early June 2025 |
Final submission to the EBA | Early July 2025 |
Publication of results | Beginning of August 2025 |
Below we share the most significant aspects for Credit Risk and related challenges. In the coming weeks we will share separate articles to cover areas related to Market Risk, Net Interest Income & Expenses and Operational Risk.
The final methodology, along with the requirements introduced by the CRR3 poses significant challenges on the execution of the Credit Risk stress testing. Earlier we provided details on this topic and possible impacts on stress testing results, see our article: “Implications of CRR3 for the 2025 EU-wide stress test” Regarding the EBA 2025 stress test we view the following 5 points as key areas of concern:
1- The EBA stress test requires different starting points; actual and restated CRR3 figures. This raises requirements in data management, reporting and implementation of related processes.
2- The EBA stress test requires banks to report both transitional and fully loaded results under CRR3; this requires the execution of additional calculations and implementation of supporting data processes.
3- The changes in classification of assets require targeted effort on the modelling side, stress test approach and related data structures.
4- Implementation of the Standardized Approach output floor as part of the stress test logic.
5- Additional effort is needed to correctly align Pillar 1 and Pillar 2 models, in terms of development, implementation and validation.
At Zanders, we specialize in risk advisory and our consultants have participated in every single EU wide stress testing exercise, as well as a few others going back to the initial stress tests in 2009 following the Great Financial Crisis. We can support you throughout all key stages of the stress testing exercise across all areas to ensure a successful submission of the final templates.
Based on the expertise in Stress Testing we have gained over the last 15 years, our clients benefit the most from our services in these areas:
- Full gap analysis against latest set of requirements
- Review, design and implementation of data processes & relevant data quality controls
- Alignment of Pillar 2 models to Pillar 1 (including CCR3 requirements)
- Design, implementation and execution of stress testing models
- Full automation of populating EBA templates including reconciliation and data quality checks.
Contact us for more information about how we can help make this your most successful run yet. Reach out to Martijn de Groot, Partner at Zanders.
Insights into cracking model risk for prepayment models
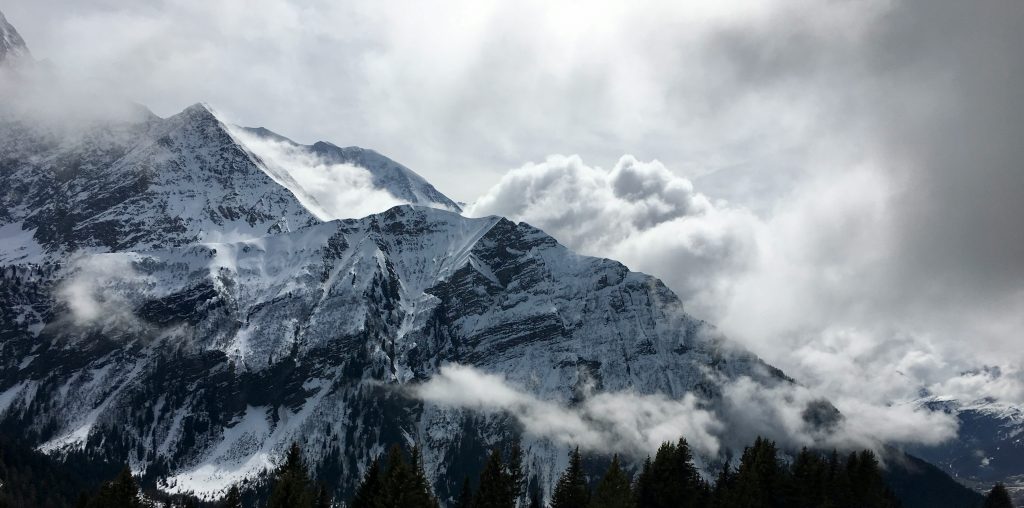
This article examines different methods for quantifying and forecasting model risk in prepayment models, highlighting their respective strengths and weaknesses.
Within the field of financial risk management, professionals strive to develop models to tackle the complexities in the financial domain. However, due to the ever-changing nature of financial variables, models only capture reality to a certain extent. Therefore, model risk - the potential loss a business could suffer due to an inaccurate model or incorrect use of a model - is a pressing concern. This article explores model risk in prepayment models, analyzing various approaches to quantify and forecast this risk.
There are numerous examples where model risk has not been properly accounted for, resulting in significant losses. For example, Long-Term Capital Management was a hedge fund that went bankrupt in the late 1990s because its model was never stress-tested for extreme market conditions. Similarly, in 2012, JP Morgan experienced a $6 billion loss and $920 million in fines due to flaws in its new value-at-risk model known as the ‘London Whale Trade’.
Despite these prominent failures, and the requirements of CRD IV Article 85 for institutions to develop policies and processes for managing model risk,1 the quantification and forecasting of model risk has not been extensively covered in academic literature. This leaves a significant gap in the general understanding and ability to manage this risk. Adequate model risk management allows for optimized capital allocation, reduced risk-related losses, and a strengthened risk culture.
This article delves into model risk in prepayment models, examining different methods to quantify and predict this risk. The objective is to compare different approaches, highlighting their strengths and weaknesses.
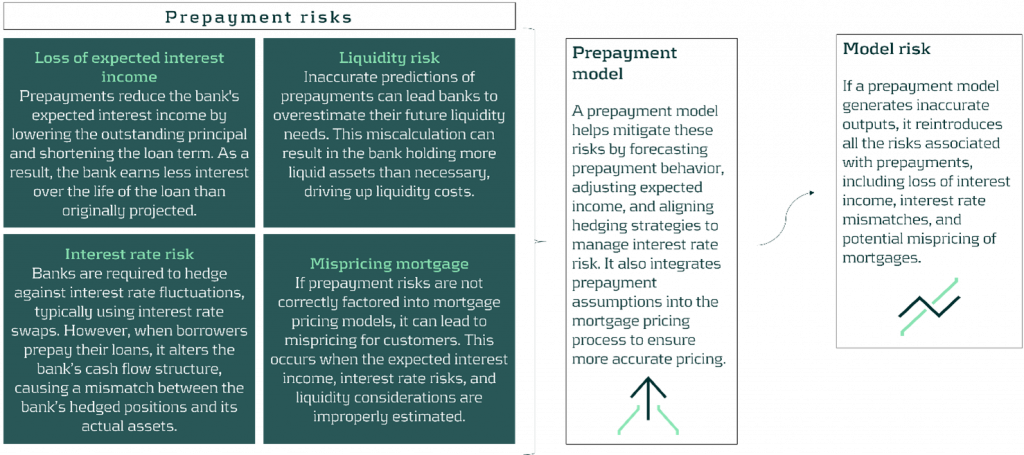
Definition of Model Risk
Generally, model risk can be assessed using a bottom-up approach by analyzing individual model components, assumptions, and inputs for errors, or by using a top-down approach by evaluating the overall impact of model inaccuracies on broader financial outcomes. In the context of prepayments, this article adopts a bottom-up approach by using model error as a proxy for model risk, allowing for a quantifiable measure of this risk. Model error is the difference between the modelled prepayment rate and the actual prepayment rate. Model error occurs at an individual level when a prepayment model predicts a prepayment that does not happen, and vice versa. However, banks are more interested in model error at the portfolio level. A statistic often used by banks is the Single Monthly Mortality (SMM). The SMM is the monthly percentage of prepayments and can be calculated by dividing the amount of prepayments for a given month by the total amount of mortgages outstanding.
Using the SMM, we can define and calculate the model error as the difference between the predicted SMM and the actual SMM:

The European Banking Authority (EBA) requires financial institutions when calculating valuation model risk to set aside enough funds to be 90% confident that they can exit a position at the time of the assessment. Consequently, banks are concerned with the top 5% and lowest 5% of the model risk distribution (EBA, 2016, 2015). 2 Thus, banks are interested in the distribution of the model error as defined above, aiming to ensure they allocate the capital optimally for model risk in prepayment models.
Approaches to Forecasting Model Risk
By using model error as a proxy for model risk, we can leverage historical model errors to forecast future errors through time-series modelling. In this article, we explore three methods: the simple approach, the auto-regressive approach, and the machine learning challenger model approach.
Simple Approach
The first method proposed to forecast the expected value, and the variance of the model errors is the simple approach. It is the most straightforward way to quantify and predict model risk by analyzing the mean and standard deviation of the model errors. The model itself causes minimal uncertainty, as there are just two parameters which have to be estimated, namely the intercept and the standard deviation.
The disadvantage of the simple approach is that it is time-invariant. Consequently, even in extreme conditions, the expected value and the variance of model errors remain constant over time.
Auto-Regressive Approach
The second approach to forecast the model errors of a prepayment model is the auto-regressive approach. Specifically, this approach utilizes an AR(1) model, which forecasts the model errors by leveraging their lagged values. The advantage of the auto-regressive approach is that it takes into account the dynamics of the historical model errors when forecasting them, making it more advanced than the simple approach.
The disadvantage of the auto-regressive approach is that it always lags and that it does not take into account the current status of the economy. For example, an increase in the interest rate by 200 basis points is expected to lead to a higher model error, while the auto-regressive approach is likely to forecast this increase in model error one month later.
Machine Learning Challenger Model Approach
The third approach to forecast the model errors involves incorporating a Machine Learning (ML) challenger model. In this article, we use an Artificial Neural Network (ANN). This ML challenger model can be more sophisticated than the production model, as its primary focus is on predictive accuracy rather than interpretability. This approach uses risk measures to compare the production model with a more advanced challenger model. A new variable is defined as the difference between the production model and the challenger model.
Similar to the above approaches, the expected value of the model errors is forecasted by estimating the intercept, the parameter of the new variable, and the standard deviation. A forecast can be made and the difference between the production model and ML challenger model can be used as a proxy for future model risk.
The advantage of using the ML challenger model approach is that it is forward looking. This forward-looking method allows for reasonable estimates under both normal and extreme conditions, making it a reliable proxy for future model risk. In addition, when there are complex non-linear relationships between an independent variable and the prepayment rate, an ML challenger can be more accurate. Its complexity allows it to predict significant impacts better than a simpler, more interpretable production model. Consequently, employing an ML challenger model approach could effectively estimate model risk during substantial market changes.
A disadvantage of the machine learning approach is its complexity and lack of interpretability. Additionally, developing and maintaining these models often requires significant time, computational resources, and specialized expertise.
Conclusion
The various methods to estimate model risk are compared in a simulation study. The ML challenger model approach stands out as the most effective method for predicting model errors, offering increased accuracy in both normal and extreme conditions. Both the simple and challenger model approach effectively predicts the variability of model errors, but the challenger model approach achieves a smaller standard deviation. In scenarios involving extreme interest rate changes, only the challenger model approach delivers reasonable estimates, highlighting its robustness. Therefore, the challenger model approach is the preferred choice for predicting model error under both normal and extreme conditions.
Ultimately, the optimal approach should align with the bank’s risk appetite, operational capabilities, and overall risk management framework. Zanders, with its extensive expertise in financial risk management, including multiple high-profile projects related to prepayments at G-SIBs as well as mid-size banks, can provide comprehensive support in navigating these challenges. See our expertise here.
Ready to take your IRRBB strategy to the next level?
Zanders is an expert on IRRBB-related topics. We enable banks to achieve both regulatory compliance and strategic risk goals by offering support from strategy to implementation. This includes risk identification, formulating a risk strategy, setting up an IRRBB governance and framework, and policy or risk appetite statements. Moreover, we have an extensive track record in IRRBB [EU1] and behavioral models such as prepayment models, hedging strategies, and calculating risk metrics, both from model development and model validation perspectives.
Contact our experts today to discover how Zanders can help you transform risk management into a competitive advantage. Reach out to: Jaap Karelse, Erik Vijlbrief, Petra van Meel, or Martijn Wycisk to start your journey toward financial resilience.
- https://www.eba.europa.eu/regulation-and-policy/single-rulebook/interactive-single-rulebook/11665
CRD IV Article 85: Competent authorities shall ensure that institutions implement policies and processes to evaluate and manage the exposures to operational risk, including model risk and risks resulting from outsourcing, and to cover low-frequency high-severity events. Institutions shall articulate what constitutes operational risk for the purposes of those policies and procedures. ↩︎ - https://extranet.eba.europa.eu/sites/default/documents/files/documents/10180/642449/1d93ef17-d7c5-47a6-bdbc-cfdb2cf1d072/EBA-RTS-2014-06%20RTS%20on%20Prudent%20Valuation.pdf?retry=1
Where possible, institutions shall calculate the model risk AVA by determining a range of plausible valuations produced from alternative appropriate modelling and calibration approaches. In this case, institutions shall estimate a point within the resulting range of valuations where they are 90% confident they could exit the valuation exposure at that price or better. In this article, we generalize valuation model risk to model risk. ↩︎
Biodiversity risks scoring: a quantitative approach
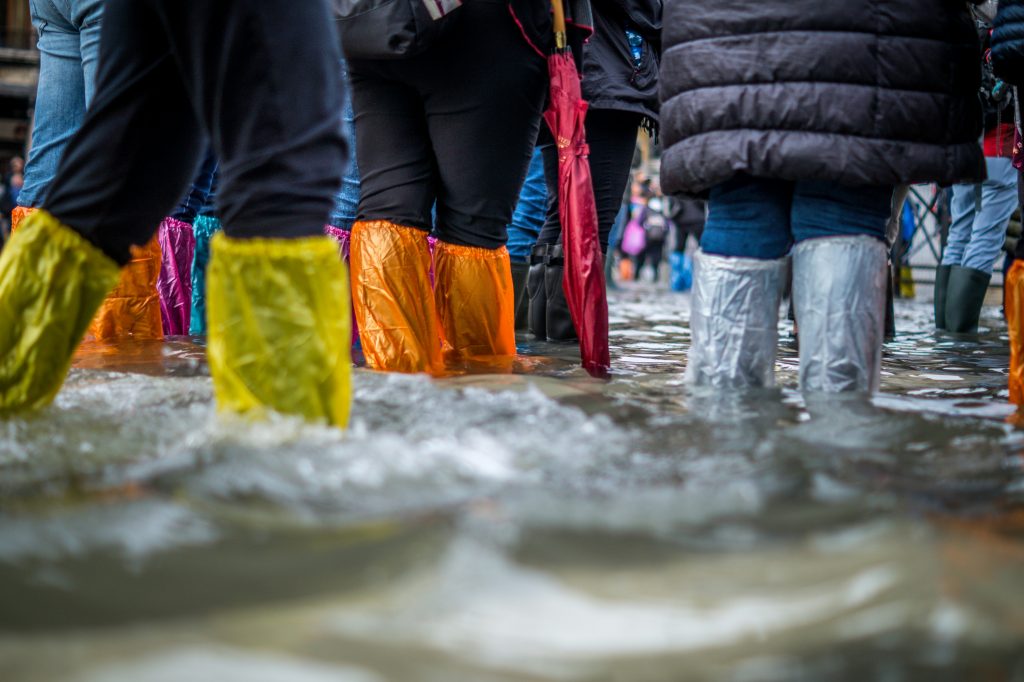
Explore how Zanders’ scoring methodology quantifies biodiversity risks, enabling financial institutions to safeguard portfolios from environmental and transition impacts.
Addressing biodiversity (loss) is not only relevant from an impact perspective; it is also quickly becoming a necessity for financial institutions to safeguard their portfolios against financial risks stemming from habitat destruction, deforestation, invasive species and/or diseases.
In a previous article, published in November 2023, Zanders introduced the concept of biodiversity risks, explained how it can pose a risk for financial institutions, and discussed the expectations from regulators.1 In addition, we touched upon our initial ideas to introduce biodiversity risks in the risk management framework. One of the suggestions was for financial institutions to start assessing the materiality of biodiversity risk, for example by classifying exposures based on sector or location. In this article, we describe Zanders’ approach for classifying biodiversity risks in more detail. More specifically, we explore the concepts behind the assessment of biodiversity risks, and we present key insights into methodologies for classifying the impact of biodiversity risks; including a use case.
Understanding biodiversity risks
Biodiversity risks can be related to physical risk and/or transition risk events. Biodiversity physical risks results from environmental decay, either event-driven or resulting from longer-term patterns. Biodiversity transition risks results from developments aimed at preventing or restoring damage to nature. These risks are driven by impacts and dependencies that an undertaking has on natural resources and ecosystem services. The definition of impacts and dependencies and its relation to physical and transitional risks is explained below:
- Companies impact natural assets through their business operations and output. For example, the production process of an oil company in a biodiversity sensitive area could lead to biodiversity loss. Impacts are mainly related to transition risk as sectors and economic activities that have a strong negative impact on environmental factors are likely to be the first affected by a change in policies, legal charges, or market changes related to preventing or restoring damage to nature.
- On the other hand, companies are dependent on certain ecosystem services. For example, agricultural companies are dependent on ecosystem services such as water and pollination. Dependencies are mainly related to physical risk as companies with a high dependency will take the biggest hit from a disruption or decay of the ecosystem service caused by e.g. an oil spill or pests.
For banks, the impacts and dependencies of their own operations and of their counterparties can impact traditional financial (credit, liquidity, and market) and non-financial (operational and business) risks. In our biodiversity classification methodology, we assess both impacts and dependencies as indicators for physical and transition risk. This is further described in the next section.
Zanders’ biodiversity classification methodology
An important starting point for climate-related and environmental (C&E) risk management is the risk identification and materiality assessment. For C&E risks, and biodiversity in particular, obtaining data is a challenge. A quantitative assessment of materiality is therefore difficult to achieve. To address this, Zanders has developed a data driven classification methodology. By classifying the biodiversity impact and dependencies of exposures based on the sector and location of the counterparty, scores that quantify the portfolio’s physical and transition risks related to biodiversity are calculated. These scores are based on the databases of Exploring Natural Capital Opportunities, Risks and Exposure (ENCORE) and the World Wide Fund for Nature (WWF).
Sector classification
The sector classification methodology is developed based on the ENCORE database. ENCORE is a public database that is recognized by global initiatives such as Taskforce on Nature-related Financial Disclosures (TNFD) and Partnership for Biodiversity Accounting Financials (PBAF). ENCORE is a key tool for the “Evaluate” phase of the TNFD LEAP approach (Locate, Evaluate, Assess and Prepare).
ENCORE was developed specifically for financial institutions with the goal to assist them in performing a high-level but data-driven scan of their exposures’ impacts and dependencies. The scanning is made across multiple dimensions of the ecosystem, including biodiversity-related environmental drivers. ENCORE evaluates the potential reliance on ecosystem services2 and the changes of impacts drivers3 on natural capital assets4. It does so by assigning scores to different levels of a sector classification (sector, subindustry and production process). These scores are assigned for 11 impact drivers and 21 ecosystem services. ENCORE provides a score ranging from Very Low to Very High for a broad range of production processes, sub-sectors and sectors.
To compute the sector scores, ENCORE does not offer a methodology for aggregating scores for impacts drivers and ecosystem services. Therefore, ENCORE does not provide an overall dependency and impact per sector, sub-industry, or production process. However, Zanders has created a methodology to calculate a final aggregated impact and dependency score. The result of this aggregation is a single impact and a single dependency score for each ENCORE sector, sub-industry or production process. In addition, an overall impacts and dependencies scores are computed for the portfolio, based on its sector distribution. In both cases, scores range from 0 (no impact/dependency) to 5 (very high impact or dependency).
Location classification
The location scoring methodology is developed based on the WWF Biodiversity Risk Filter (hereafter called WWF BRF).5 The WWF BRF is a public tool that supports a location-specific analysis of physical- and transition-related biodiversity risks.
The WWF BRF consists of a set of 33 biodiversity indicators: 20 related to physical risks and 13 related to reputational risks, which are provided at country, but also on a more granular regional level. These indicators are aggregated by the tool itself, which ultimately provides one single scape physical risk and scape reputational risk per location.
To compute overall location scores, the WWF BRF does not offer a methodology for aggregating scores for countries and determine the overall transition risk (based on the scape reputational risk scores) and physical risk (based on the scape physical risk scores). However, Zanders has created a methodology to calculate a final aggregated transition and physical risk score for the portfolio, based on its geographical distribution. The result of this aggregation is a single transition and physical risk score for the portfolio, ranging from 0 (no risk) to 5 (very high risk).
Use case: RI&MA for biodiversity risks in a bank portfolio
In this section, we present a use case of classifying biodiversity risks for the portfolio of a fictional financial institution, using the sector and location scoring methodologies developed by Zanders.
The exposures of this financial institution are concentrated in four sectors: Real estate, Oil & Gas, Soft commodities and Luxury goods. Moreover, the operations of these sectors are located across four different countries: the Netherlands, Switzerland, Morocco and China. The following matrix shows the percentage of exposures of the financial institution for each combination of sector and country:

ENCORE provides scores for 11 ecosystem services and 21 impacts drivers. Those related to biodiversity risks are transformed to a range from 0 to 5. After that, biodiversity ecosystem services and biodiversity impacts drivers are aggregated into an overall biodiversity impacts and dependencies scores, respectively. The following table shows the mapping between the sectors in the portfolio and the corresponding sub-industry in the ENCORE database, including the aggregated biodiversity impacts and dependencies scores computed for those sub-industries. The mapping is done at sub-industry level, since it is the level of granularity of the ENCORE sector classification that better fits the sectors defined in the fictional portfolio. In addition, the overall impacts and dependencies scores are computed, by taking the weighted average sized by the sector distribution of the portfolio. This leads to scores of 3.8 and 2.4 for the impacts and dependencies scores, respectively.

The WWF BRF provides biodiversity indicators at country level. It already provides an aggregated score for physical risk (namely, scape physical score) and for transition risk (namely, scape reputational risk score), so no further aggregation is needed. Therefore, the corresponding scores for the four countries within the bank portfolio are selected. As the last step, the location scores are transformed to a range similar to the sector scores, i.e., from 0 (no physical/transition risk) to 5 (very high physical/transition risk). The results are shown in the following table. In addition, the overall impacts and dependencies scores are computed, by taking the weighted average sized by the geographical distribution of the portfolio. This leads to scores of 3.9 and 3.3 for the physical and transition risk scores, respectively.
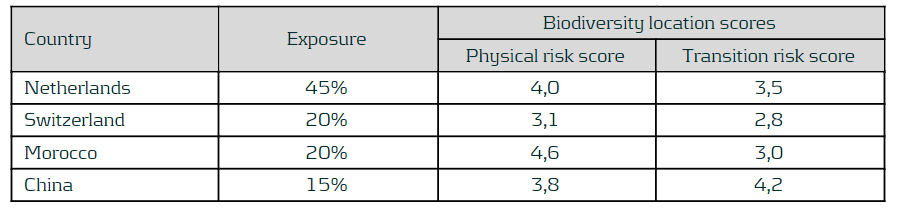
Results of the sector and location scores can be displayed for a better understanding and to enable comparison between sectors and countries. Bubble charts, such as the ones show below, present the sectors and location scores together with the size of the exposures in the portfolio (by the size of each bubble).
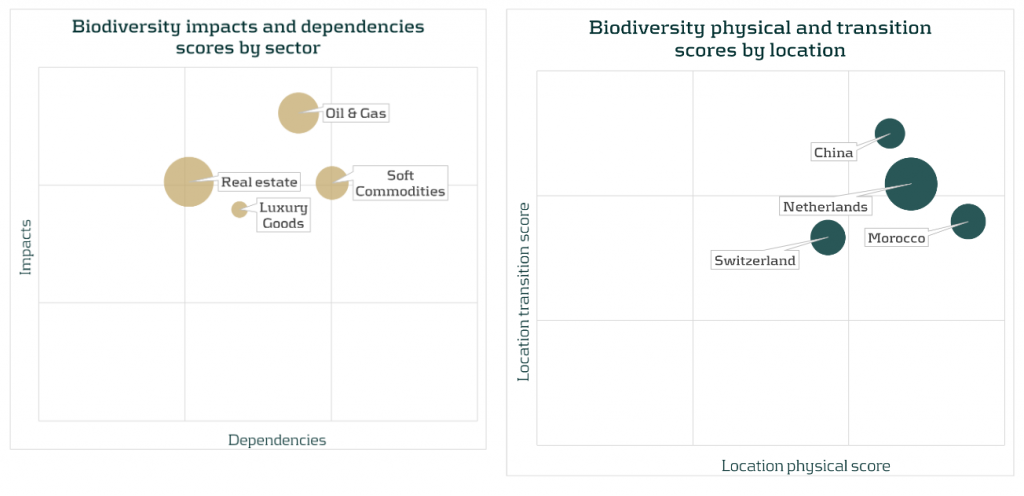
Combined with the size of the exposures, the results suggest that biodiversity-related physical and transition risks could result in financial risks for Soft commodities and Oil & Gas. This is due to high impacts and dependencies and their relevant size in the portfolio. Moreover, despite a low dependencies score, biodiversity risks could also impact the Real estate sector due to a combination of its high impact score and the high sector concentration (45% of the portfolio). From a location perspective, exposures located in China could face high biodiversity transition risks, while exposures located in Morocco are the most vulnerable to biodiversity physical risks. In addition, relatively high scores for both physical and transition risk scores for Netherlands, combined with the large size of these exposures in the portfolio, could also lead to additional financial risk.’
These results, combined with other information such as loan maturities, identified transmission channels, or expert inputs, can be used to inform the materiality of biodiversity risks.
Conclusion
Assessing the materiality of biodiversity risks is crucial for financial institutions in order to understand the risks and opportunities in their loan portfolios. In this article, Zanders has presented its approach for an initial quantification of biodiversity risks. Curious to learn how Zanders can support your financial institutions with the identification and quantification of biodiversity risks and the integration into the risk frameworks? Please reach out to Marije Wiersma, Iryna Fedenko or Miguel Manzanares.
- https://zandersgroup.com/en/insights/blog/biodiversity-risks-and-opportunities-for-financial-institutions-explained ↩︎
- In accordance with ENCORE, ecosystem services are the links between nature and business. Each of these services represent a benefit that nature provides to enable or facilitate business production processes. ↩︎
- In accordance with ENCORE AND Natural Capital Protocol (2016), an impacts driver is a measurable quantity of a natural resource that is used as an input to production or a measurable non-product output of business activity. ↩︎
- In accordance with ENCORE, natural capital assets are specific elements within nature that provide the goods and services that the economy depends on. ↩︎
- The WWF also provides a similar tool, the WWF Water Risk Filter, which could be used as to assess specific water-related environmental risks. ↩︎
Navigating Treasury Transformation: Key Insights from TAC’s SAP Conference in Brussels
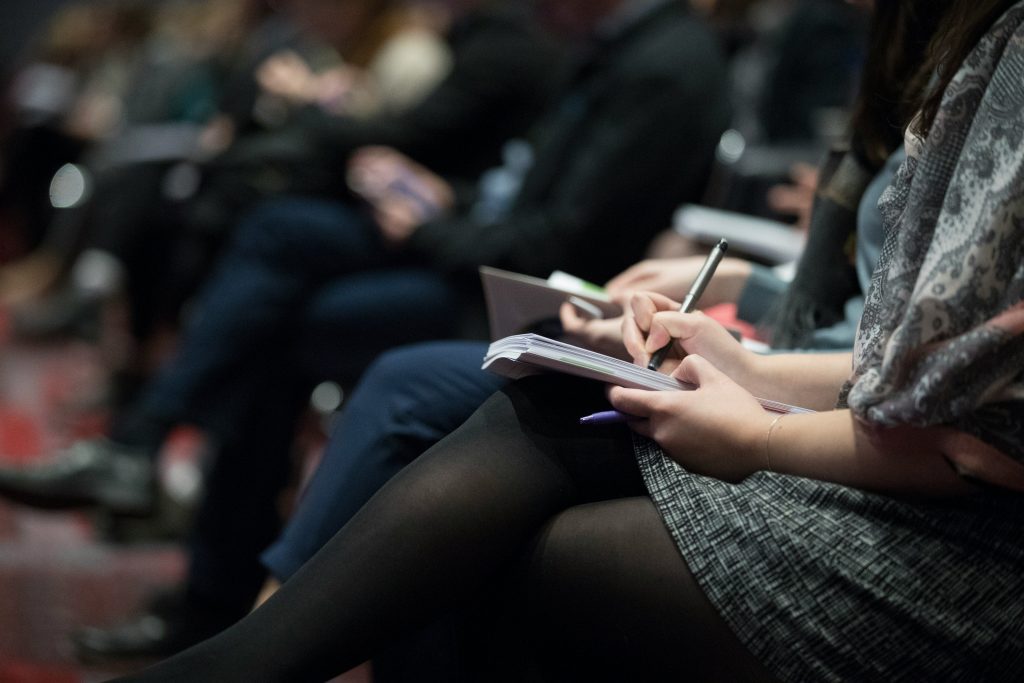
At TAC’s recent SAP for Treasury and Working Capital Management in Brussels, SAP alongside some of their clients present several topics that rank highly on the treasurer’s agenda.
SAP highlighted their public vs. private cloud offerings, RISE and GROW products, new AI chatbot applications, and their SAP Analytics Cloud solution. In addition to SAP's insights, several clients showcased their treasury transformation journeys with a focus on in-house banking, FX hedge management, and payment factory implementation. This article provides a brief overview of SAP's RISE and GROW offerings, with a larger focus on SAP’s public vs. private cloud offerings and their new AI virtual assistant, Joule.
SAP RISE and GROW
The SAP RISE solution seeks to help companies transition to cloud-based services. It is designed as a comprehensive offering that combines software, services, and support into a single package, including the core components of SAP S/4HANA cloud, Business Process Intelligence (BPI), SAP Business Network, and SAP Business Technology Platform (BTP). On the other hand, SAP GROW is a program that facilitates the implementation and organization of SAP solutions. This offering is more tailored towards optimizing, rather than transitioning, company processes. SAP GROW still includes S/4HANA public cloud solution, enabling growing companies to manage their operations without requiring extensive on-site infrastructure.
Ultimately, companies experiencing significant growth and seeking scalable, efficient solutions would benefit most from the SAP GROW offering, while SAP RISE is more suited for companies looking to accelerate their digital transformation with a focus on agility, rapid innovation, and business resilience.
Public Cloud vs. Private Cloud
SAP systems can be hosted both on the public and private cloud. The public cloud delivers greater scalability, whereas the private cloud provides enhanced security and complete control of data and governance. Often the choice between SAP public or private cloud is driven by business requirements, budget, compliance needs, and desired levels of customization. These variables, along with other important factors, are compared in Figure 1.
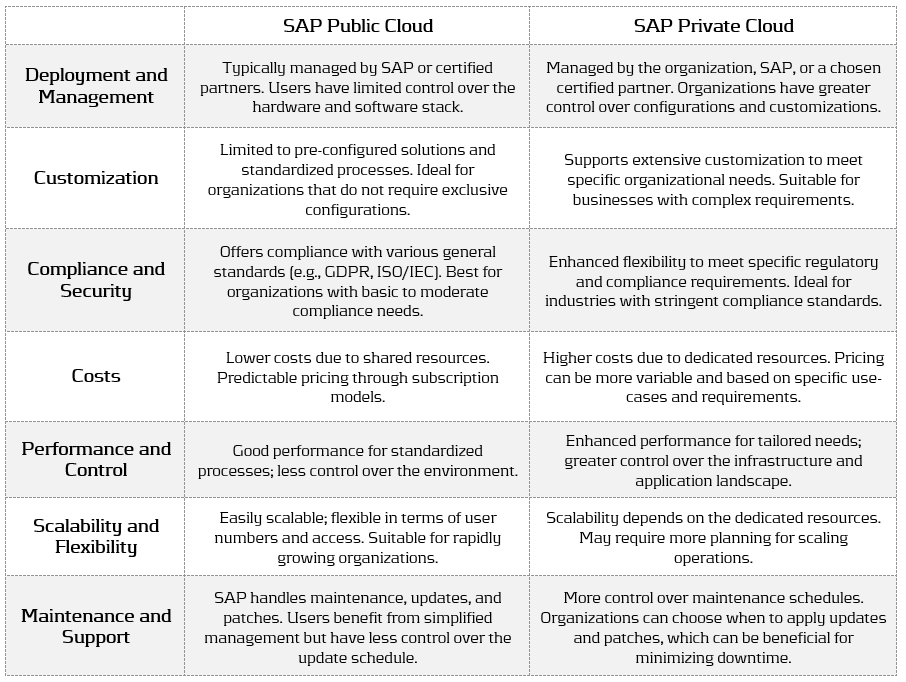
Figure 1: SAP Public Cloud vs. SAP Private Cloud
In summary, organizations considering SAP should carefully weigh these differences when choosing between public and private cloud. SAP is actively developing the functionality within its public cloud offering, making it an increasingly suitable option for both small-to-medium enterprises seeking rapid deployment and cost efficiency, as well as larger enterprises that require powerful solutions with limited customization needs. On the other hand, SAP private cloud remains a preferred choice for larger enterprises with complex, unique process requirements, extensive customization needs, and strict data compliance regulations.
Joule's Virtual Assistant
SAP's Business AI solutions initiative is introducing its newest member, the Joule Copilot. Similar to OpenAI's ChatGPT, the Joule virtual assistant is available at the user's command. Users simply need to ask the copilot questions or explain a particular issue, and Joule will provide intelligent answers drawn from the vast amount of business data stored across the SAP systems and third-party sources.
Joule Key Features
Contextual Recommendations
Provide personalized, context-specific suggestions based on the user's role and activities. Joule can help users by suggesting possible next steps, identifying potential issues, and offering insights that can be actioned upon by users.
Enhanced User Experience
Offers an intuitive, interactive interface designed to simplify user interaction with SAP applications. Joule aims to reduce complexity and streamline workflows, allowing users to simplify their daily processes.
Real-Time Insights
Artificial Intelligence and Machine Learning capabilities enable Joule to analyze vast amounts of data in real-time, providing predictive insights and analytics to support the user's decision-making process.
Integration with SAP Ecosystem
Joule is fully integrated with SAP’s existing products, such as S/4HANA and SAP Business Technology Platform (BTP), ensuring seamless data flow and interconnectivity across various SAP solutions.
Customization and Extensibility
Joule can be tailored to the specific needs of different industries and business processes. It also accounts for the specific role of the user when providing recommendations and can be customized to align with a company’s organizational requirements and workflows within their system.
Applications of Joule in Finance
SAP Joule can significantly enhance financial operations by leveraging AI-driven insights, automation, and predictive analytics. Joule has many applications within finance, the most important being:
Automated Financial Reporting
SAP Joule can automatically generate and distribute financial reports, offering insights based on real-time data. Joule uses its AI and ML capabilities to identify trends, flag anomalies, and provide explanations for variances, ultimately helping finance teams to make informed decisions quickly. Not only does Joule provide insight, but it also increases operational efficiency, allowing finance professionals to focus on strategic activities rather than report gathering and distribution.
Predictive Analytics and Forecasting
SAP Joules embedded ML capabilities enable the prediction of future financial outcomes based on historical data and current trends. Whether you are forecasting revenues, cash flows, or expenses, Joule provides the ideal tools for an accurate forecast. Alongside the forecasting capabilities, Joule can also assess financial risks by analyzing market conditions, historical data, and other relevant factors, which allows risk management to take a proactive approach to risk mitigation.
Accounts Receivable and Payable Management
Joule can predict payment behaviors, which can help organizations optimize their cash flows by forecasting when payments are likely to be received or when outgoing payments will occur. In addition to this, Joule has automatic invoice processing capabilities, which can reduce errors and speed up the accounts payable process.
Investment Analysis
For organizations managing investments, Joule can analyze portfolio performance and suggest adjustments to maximize return while still complying with risk limits. Embedded scenario analysis capabilities assist finance teams to assess the potential impact of various investment decisions on their portfolio.
Real-Time Financial Monitoring
Finance teams can use Joule to create real-time dashboards that provide an overview of key financial metrics, enabling quick responses to emerging issues or opportunities. Joule can set up alerts for critical financial thresholds, such as reserves dropping below a certain level, to ensure timely intervention.
All in all, SAP Joule represents a significant step forward in SAP’s strategy to embed AI and ML into its core products, empowering business users with smarter, data-driven capabilities.
Conclusion
This conference summary briefly highlights SAP’s RISE and GROW offerings, with RISE driving cloud-based digital transformation and GROW striving to optimize operations. It contrasts the scalability and cost-efficiency of the public cloud with the control and customization offered by the private cloud. Lastly, it introduces SAP’s new virtual assistant seeking to enhance financial operations through AI-driven insights, automation, and scalability to improve productivity while still maintaining user control over decisions and data security. If you have any further questions regarding the SAP conference or any information in this article, please contact j.vinson@zandersgroup.com.
In-House Banking vs. In-House Cash: Should You Make the Change?
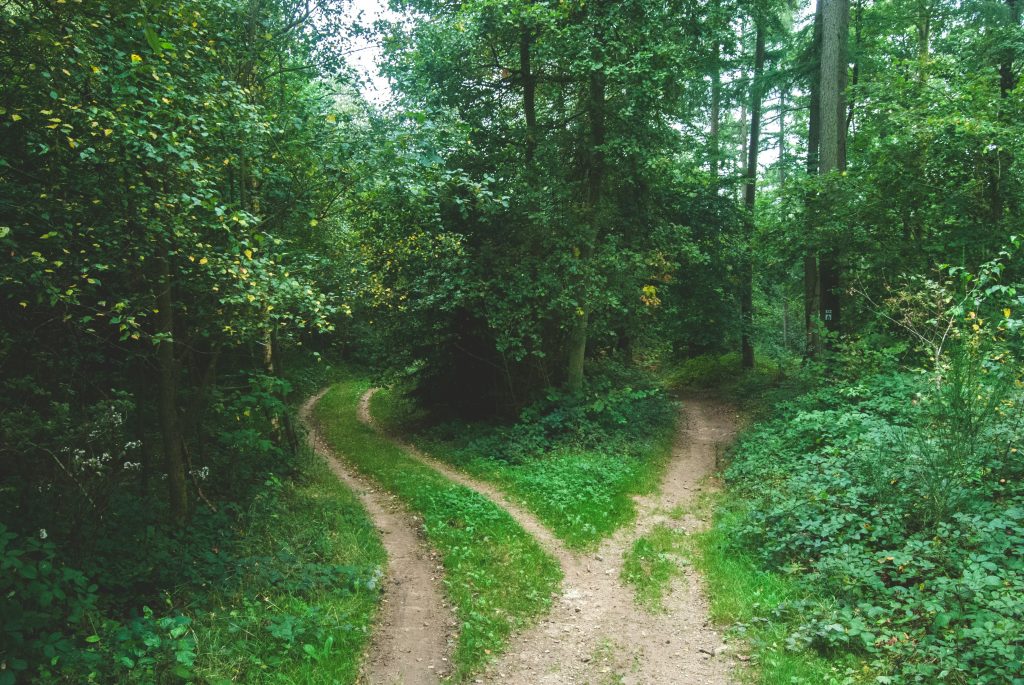
An introduction to IHB for companies planning a new implementation, along with key considerations for those transitioning from IHC.
SAP In-House Cash (IHC) has enabled corporates to centralize cash, streamline payment processes, and recording of intercompany positions via the deployment of an internal bank. S/4 HANA In-House Banking (IHB) , released in 2022, in combination with Advanced Payment Management (APM), is SAP’s revamped internal banking solution.
This article will introduce IHB for corporates planning a new implementation and highlight some key considerations for those looking to transition from IHC.
IHB is embedded in APM and included in the same license. It leverages APM’s payment engine functionality and benefits from direct integration for end-to-end processing, monitoring/reporting, and exception handling.
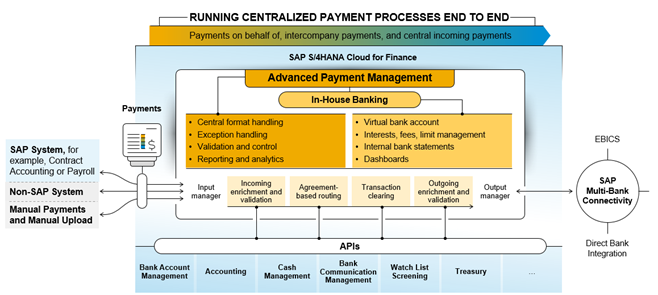
Figure 1: Solution architecture / Integration of In-House Banking (SAP, 2023)
IHC and IHB share several core functionalities, including a focus on managing intercompany financial transactions and balances effectively and ensuring compliance with regulatory requirements. Both solutions also integrate seamlessly with the broader SAP ecosystem and offer robust reporting capabilities.
However, there are significant differences between the two. While IHC relies on the traditional SAP GUI interface, IHB runs on the more modern and intuitive SAP Fiori interface, offering a better user experience. IHB overcomes limitations of IHC, namely in areas such as cut-off times and payment approval workflows and provides native support for withholding tax. Moreover, it also offers tools for managing master data, including the mass download and upload of IHB accounts, features that are otherwise missing in IHC.
Two key distinctions exist in payment routing flexibility and the closing process. IHB, when deployed with APM, manages payment routing entirely as master data, enabling organizations to more easily adapt to evolving business requirements, whereas IT involvement for configuration changes is required for those running IHC exclusively. Lastly, IHB supports multiple updates throughout the day, such as cash concentration, statement reporting, and transfers to FI, and is hence more in tune with the move towards real-time information, whereas IHC is restricted to a rigid end-of-day closing process.
Intrigued? Continue reading to delve deeper into how IHB compares with IHC.
Master data
2.1 Business Partners
The Business Partner (BP) continues to be a pre-requisite for the opening of IHB accounts, but new roles have been introduced.
Tax codes for withholding tax applicable to credit or debit interest can now be maintained at the BP level and feed into the standard account balancing process for IHB. The Withholding Tax set up under FI is leveraged and hence avoids the need for custom development as currently required for IHC.
2.2 In-House Bank Accounts
Relative to IHC, the process of maintaining accounts in IHB is simplified and more intuitive.
Statements can be sent to various recipients and in different formats (e.g., CAMT.53, PDF) based on settings maintained at account level. Intraday statement reporting functionality is included, as well as PDF notifications for balances on accounts and interest calculated as part of the account balancing process.
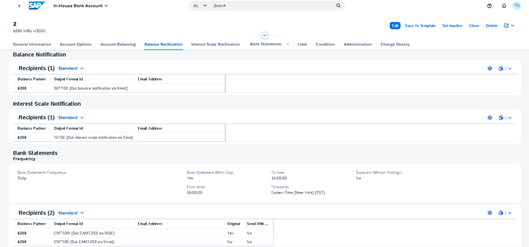
Figure 2: Maintaining IHB Account Correspondence
IHB offers native functionality for mass account download/upload, a feature that is missing in IHC. The mass download option allows data to be exported to Excel, adjusted offline, and subsequently loaded into BAM .
In the upcoming release, the bank account subledger concept will also be supported for IHB accounts managed in BAM.
2.3 Conditions
The underlying setup has been simplified and can now be performed entirely as master data, unlike for IHC, which in comparison requires some customization to be done as part of the implementation.
IHC technically offers slightly more interest conditions (e.g., commitment interest), but IHB covers the fundamentals for account balancing. More importantly, average/linear compound interest calculation methodology is available with IHB to support risk-free rates.
2.4 Workflows
Unlike IHC, which only offers the option of activating “dual control” for some processes (e.g., closure of IHC accounts), IHB introduces flexible workflows for all core master data attributes (e.g., accounts, conditions, limits, etc.).
IHB's flexible workflows allow for multiple approval steps and dynamic workflow recipient determination based on predefined conditions.
Transactional Data
3.1 Scenarios & payment integration/routing
The following set of scenarios are in scope for IHB:
- Intercompany payments
- Payments On-Behalf-Of (POBO)
- Central Incoming
- Cash Pooling
Payment integration is achieved via APM and supports several options, namely IDocs, connectors for Multi-Bank Connectivity (MBC ), file uploads, etc. Moreover, the connector for MBC can be used to support more elaborate integration scenarios, such as connecting decentralized AP systems or a public cloud instance to APM.
More noteworthy is that the flexible payment routing in APM is used to handle the routing of payments and is managed entirely according to business needs as master data. This is particularly relevant for corporates running IHC as a “payment factory” who are considering the adoption of APM & IHB, as routing is entirely configuration-based when using IHC exclusively. There are additional advantages of using APM as a payment factory, especially in terms of payment cut-offs and approval workflows. However, these benefits can be obtained by using APM in conjunction with IHB or IHC.
3.2 Foreign Currency Payments
A distinct set of bid/offer rates can be assigned per transaction type and used to convert between payment currency and IHC account currency at provisional and final posting stages. In contrast, for IHB, a single exchange rate type is maintained at the IHB Bank Area level and drives the FX conversion.
Compared to IHC, applying different rates depending on the payment scenario will require a different design, and special consideration is needed for corporates running complex multilateral netting processes in IHC that are planning to transition to IHB.
Intraday/End of Day Processing
4.1 End of Day Sequence
The end-of-day closing concept applies to IHB as well. Unlike IHC, IHB allows many of the related steps, such as intraday statement reporting, cash concentration, and transfers to FI, to be triggered throughout the day.
A dedicated app further streamlines processing by enabling the scheduling and management of jobs via pre-delivered templates.
4.2 Bank Statements
APM converters are leveraged to produce messages in the desired format (MT940, CAMT.53, or PDF ). Unlike IHC, FINSTA IDocs are no longer supported, which is an important factor to consider when migrating participants that are still on legacy ERP systems.
The settings maintained under the bank statement section of the IHB account drive the format and distribution method (e.g., delivery via MBC or email) to the participants.
4.3 General Ledger Transfer
The new Accounting Business Transaction Interface (ABTI) supports general ledger transfers from IHB to FI several times a day, unlike IHC, which is triggered only once at the end of the day.
Overall, the accounting schemas are more straightforward, which is reflected in the underlying setup required to support IHB. However, relative to IHC, there is technically less flexibility in determining the relevant G/Ls for end-of-day transfers to FI. Due diligence is recommended for corporates moving from IHC to ensure that existing processes are adapted to the new ways of working.
Conclusion
There is no official end-of-life support date for IHC, so corporates can still implement it with or without APM, though this approach presents challenges. Key considerations include
the lack of ongoing development for IHC, SAP’s focus on ensuring IHB matches IHC’s capabilities, and the fact that IHB is already included in the APM license, while IHC requires a separate license.
Initial issues with IHB are expected but will likely be resolved as more companies adopt the functionality and additional features are rolled out. For corporates with moderately complex requirements or those willing to align their processes with standard functionality, IHB is ultimately easier to implement and manage operationally.
To ensure a smooth transition to or adoption of IHB, Zanders offers expert implementation services. If your organization is contemplating IHB or transitioning from IHC, contact Zanders for guidance and support with any questions you may have.
References
SAP, 2023. Solution architecture - Integration of In-House Banking [Online] SAP. Available from: https://help.sap.com/docs/SAP_S4HANA_ON-PREMISE/e200555127f24878bed8d1481c9d5a0b/3dbe688b4c8840da8567f811be2bc1b4.html?locale=en-US&version=2023.001